Variational autoencoder–based neural electrocardiogram synthesis trained by FEM-based heart simulator
Cardiovascular Digital Health Journal(2023)
摘要
Background:For comprehensive electrocardiogram (ECG) synthesis, a recent promising approach has been based on a heart model with physical and chemical cardiac parameters. However, the problem is that such approach requires a high-cost and limited environment using supercomputers owing to the massive computation.
Objective:The purpose of this study is to develop an efficient method for synthesizing 12-lead ECG signals from cardiac parameters.
Methods:The proposed method is based on a variational autoencoder (VAE). The encoder and decoder of the VAE are conditioned by the cardiac parameters so that it can model the relationship between the ECG signals and the cardiac parameters. The training data are produced by a comprehensive, finite element method (FEM)-based heart simulator. New ECG signals can then be synthesized by inputting the cardiac parameters into the trained VAE decoder without relying on enormous computational resources. We used 2 metrics to evaluate the quality of ECG signals synthesized by the proposed model.
Results:Experimental results showed that the proposed model synthesized adequate ECG signals while preserving empirically important feature points and the overall signal shapes. We also explored the optimal model by varying the number of layers and the size of latent variables in the proposed model that balances the model complexity and the simulation accuracy.
Conclusion:The proposed method has the potential to become an alternative to computationally expensive FEM-based heart simulators. It is able to synthesize ECGs from various cardiac parameters within seconds on a personal laptop computer.
更多查看译文
关键词
Variational autoencoder,Electrocardiogram,Synthesis,Cardiac parameters,Finite element method
AI 理解论文
溯源树
样例
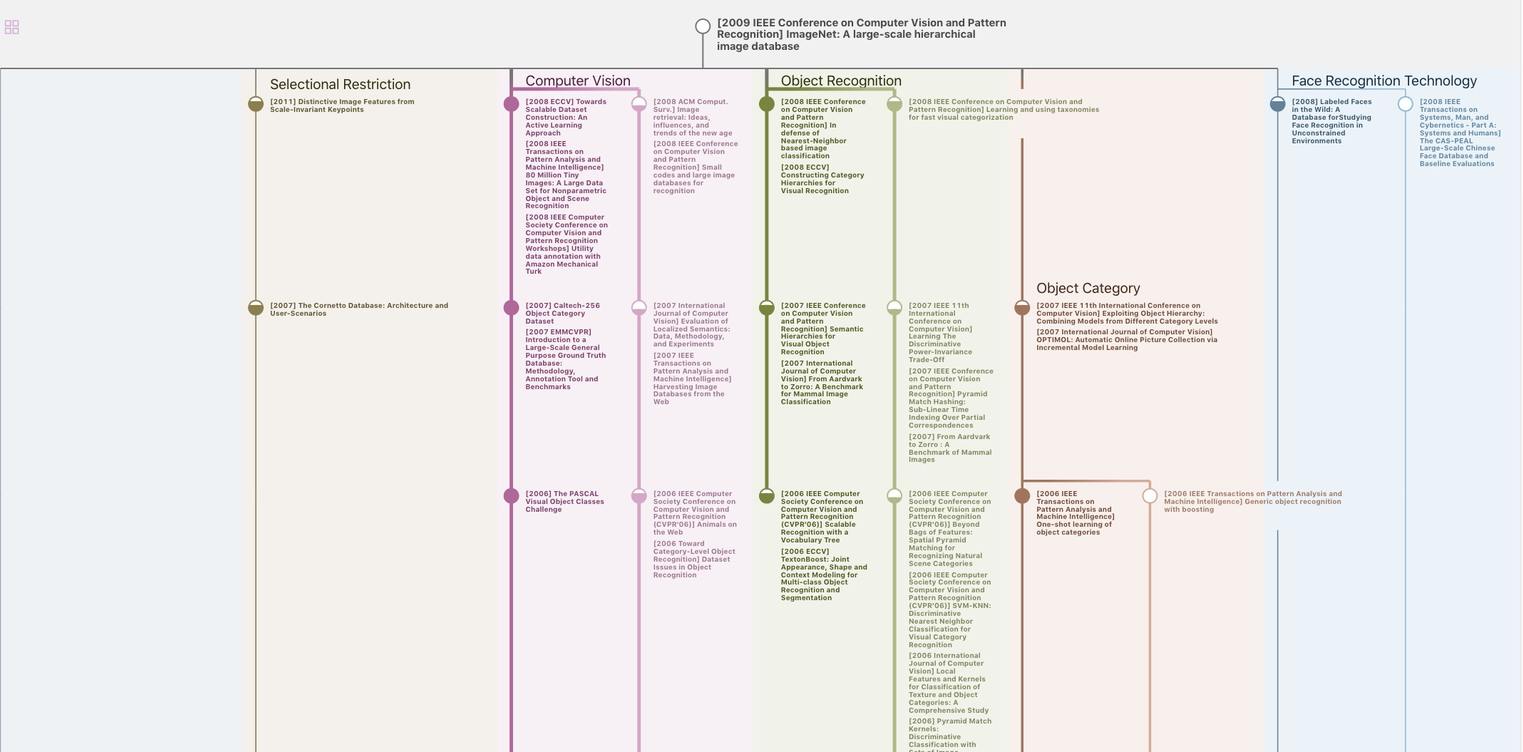
生成溯源树,研究论文发展脉络
Chat Paper
正在生成论文摘要