Sleep, physical activity and panic attacks: A two-year prospective cohort study using smartwatches, deep learning and an explainable artificial intelligence model
SLEEP MEDICINE(2024)
摘要
Background: Sleep and physical activity suggestions for panic disorder (PD) are critical but less surveyed. This two-year prospective cohort study aims to predict panic attacks (PA), state anxiety (SA), trait anxiety (TA) and panic disorder severity (PDS) in the upcoming week. Methods: We enrolled 114 PD patients from one general hospital. Data were collected using the DSM-5, the MINI, clinical app questionnaires (BDI, BAI, PDSS-SR, STAI) and wearable devices recording daily sleep, physical activity and heart rate from 16 June 2020 to 10 June 2022. Our teams applied RNN, LSTM, GRU deep learning and SHAP explainable methods to analyse the data. Results: The 7-day prediction accuracies for PA, SA, TA, and PDS were 92.8 %, 83.6 %, 87.2 %, and 75.6 % from the LSTM model. Using the SHAP explainable model, higher initial BDI or BAI score and comorbidities with depressive disorder, generalized anxiety disorder or agoraphobia predict a higher chance of PA. However, PA decreased under the following conditions: daily average heart rate, 72-87 bpm; maximum heart rate, 100-145 bpm; resting heart rate, 55-60 bpm; daily climbing of more than nine floors; total sleep duration between 6 h 23 min and 10 h 50 min; deep sleep, >50 min; and awake duration, <53 min. Limitations: Moderate sample size and self-report questionnaires were the limitations. Conclusions: Deep learning predicts recurrent PA and various anxiety domains with 75.6-92.8 % accuracy. Recurrent PA decreases under adequate daily sleep and physical activity.
更多查看译文
关键词
Panic attack,Panic disorder,Smartwatch,Deep learning,Explainable artificial intelligence,Heart rate variability
AI 理解论文
溯源树
样例
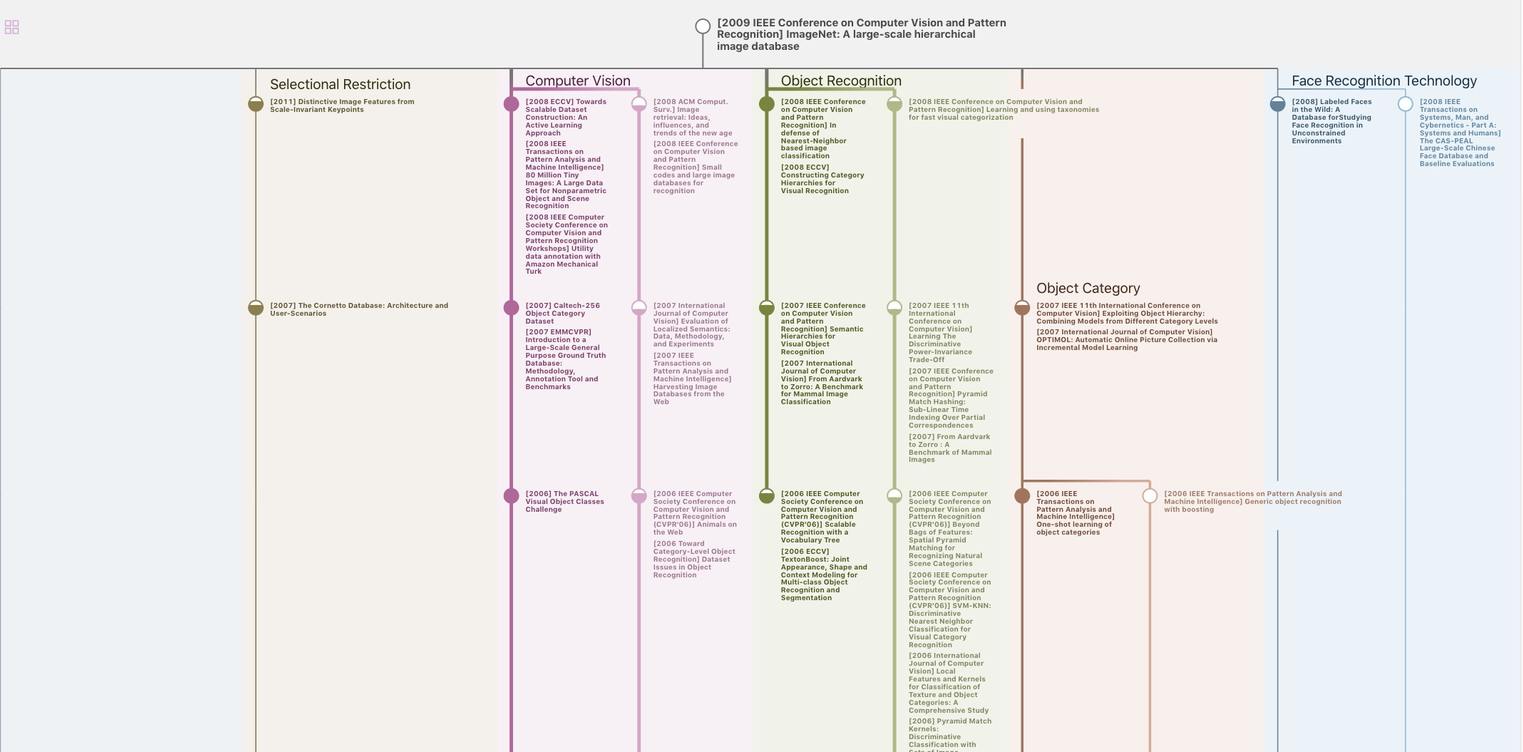
生成溯源树,研究论文发展脉络
Chat Paper
正在生成论文摘要