Skip-RCNN: A Cost-Effective Multivariate Time Series Forecasting Model
IEEE ACCESS(2023)
摘要
Multivariate time series (MTS) forecasting is a crucial aspect in many classification and regression tasks. In recent years, deep learning models have become the mainstream framework for MTS forecasting. Among these deep learning methods, the transformer model has been proved particularly effective due to its ability to capture long- and short-term dependencies. However, the computational complexity of transformer-based models sets the obstacles for resource-constrained scenarios. To address this challenge, we propose a novel and efficient Skip-RCNN network that incorporates Skip-RNN and Skip-CNN modules to split the MTS into multiple frames with various time intervals. Thanks to the skipping process of Skip-RNN and Skip-CNN, the resulting network could process information with different reception field together and achieves better performance than the state-of-the-art network. We conducted comparative experiments using our proposed method and six baseline models on seven publicly available datasets. The results demonstrate that our model outperforms other baseline methods in accuracy under most conditions and surpasses the transformer-based model with 0.098 for a short interval and 0.068 for a long interval. Our Skip-RCNN network presents a promising approach to MTS forecasting that can meet the demands of resource-constrained prediction scenarios.
更多查看译文
关键词
Deep learning,multivariate time series forecasting,feature fusion
AI 理解论文
溯源树
样例
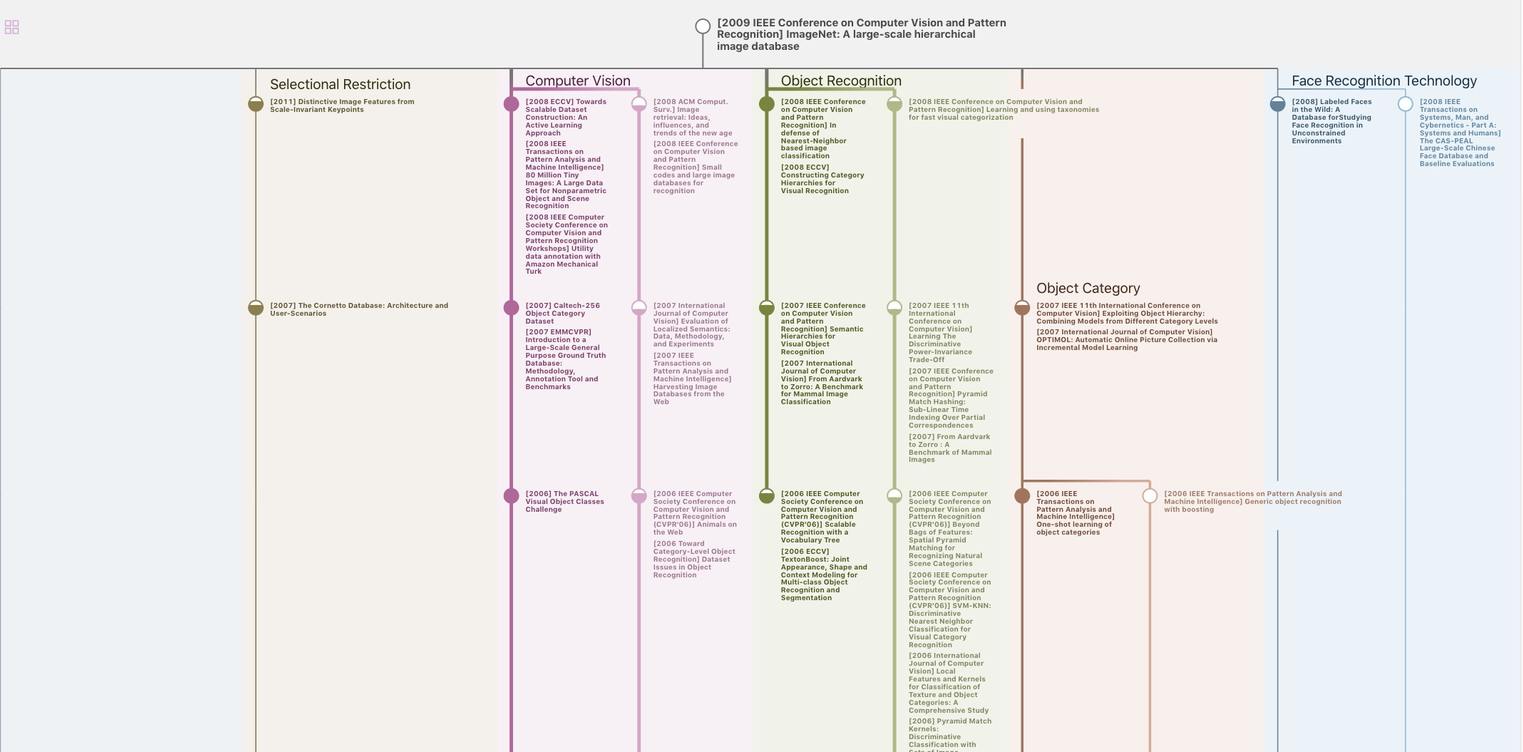
生成溯源树,研究论文发展脉络
Chat Paper
正在生成论文摘要