ARBiBench: Benchmarking Adversarial Robustness of Binarized Neural Networks
CoRR(2023)
摘要
Network binarization exhibits great potential for deployment on
resource-constrained devices due to its low computational cost. Despite the
critical importance, the security of binarized neural networks (BNNs) is rarely
investigated. In this paper, we present ARBiBench, a comprehensive benchmark to
evaluate the robustness of BNNs against adversarial perturbations on CIFAR-10
and ImageNet. We first evaluate the robustness of seven influential BNNs on
various white-box and black-box attacks. The results reveal that 1) The
adversarial robustness of BNNs exhibits a completely opposite performance on
the two datasets under white-box attacks. 2) BNNs consistently exhibit better
adversarial robustness under black-box attacks. 3) Different BNNs exhibit
certain similarities in their robustness performance. Then, we conduct
experiments to analyze the adversarial robustness of BNNs based on these
insights. Our research contributes to inspiring future research on enhancing
the robustness of BNNs and advancing their application in real-world scenarios.
更多查看译文
AI 理解论文
溯源树
样例
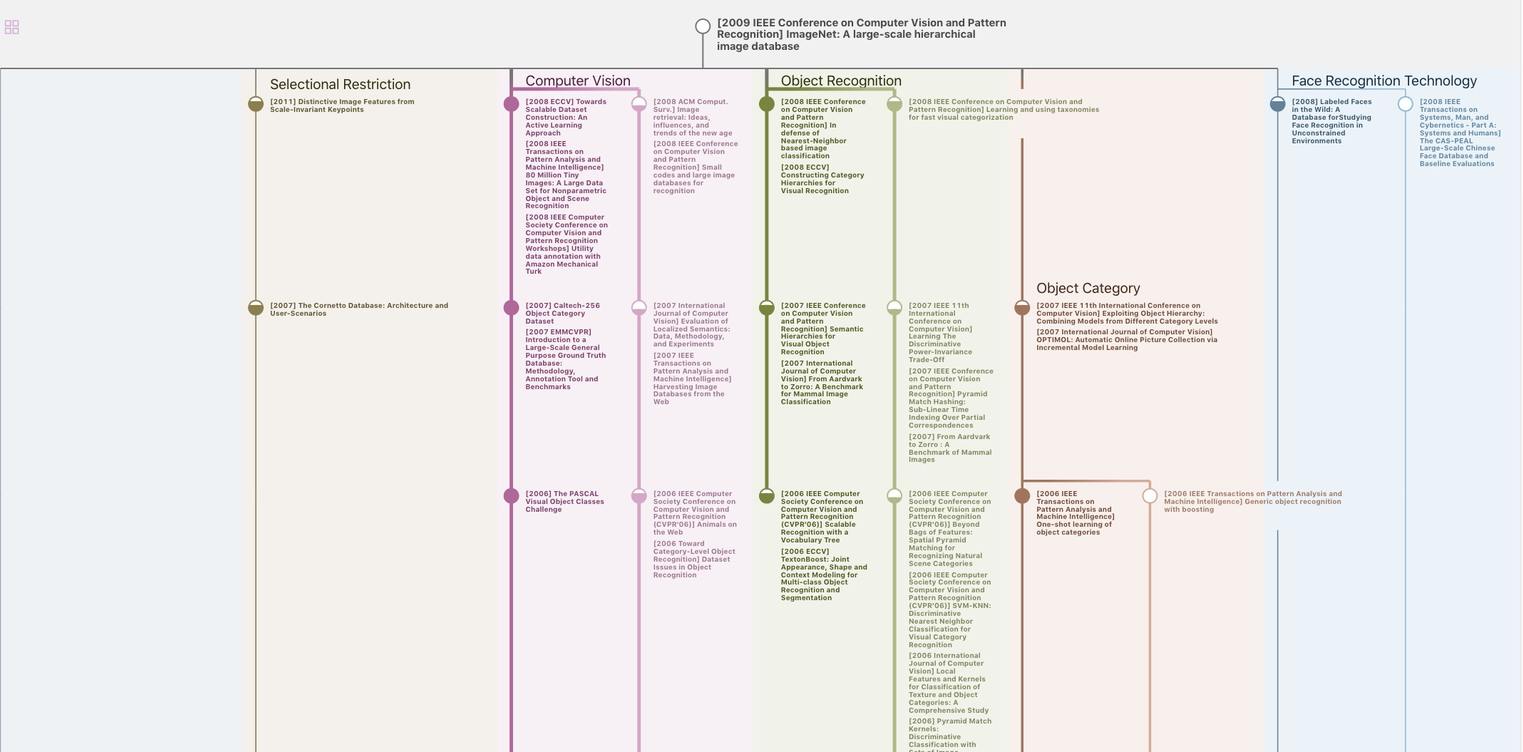
生成溯源树,研究论文发展脉络
Chat Paper
正在生成论文摘要