Where and How to Attack? A Causality-Inspired Recipe for Generating Counterfactual Adversarial Examples
AAAI 2024(2024)
摘要
Deep neural networks (DNNs) have been demonstrated to be vulnerable to well-crafted adversarial examples, which are generated through either well-conceived L_p-norm restricted or unrestricted attacks. Nevertheless, the majority of those approaches assume that adversaries can modify any features as they wish, and neglect the causal generating process of the data, which is unreasonable and unpractical. For instance, a modification in income would inevitably impact features like the debt-to-income ratio within a banking system. By considering the underappreciated causal generating process, first, we pinpoint the source of the vulnerability of DNNs via the lens of causality, then give theoretical results to answer where to attack. Second, considering the consequences of the attack interventions on the current state of the examples to generate more realistic adversarial examples, we propose CADE, a framework that can generate Counterfactual ADversarial Examples to answer how to attack. The empirical results demonstrate CADE's effectiveness, as evidenced by its competitive performance across diverse attack scenarios, including white-box, transfer-based, and random intervention attacks.
更多查看译文
关键词
ML: Adversarial Learning & Robustness,ML: Causal Learning
AI 理解论文
溯源树
样例
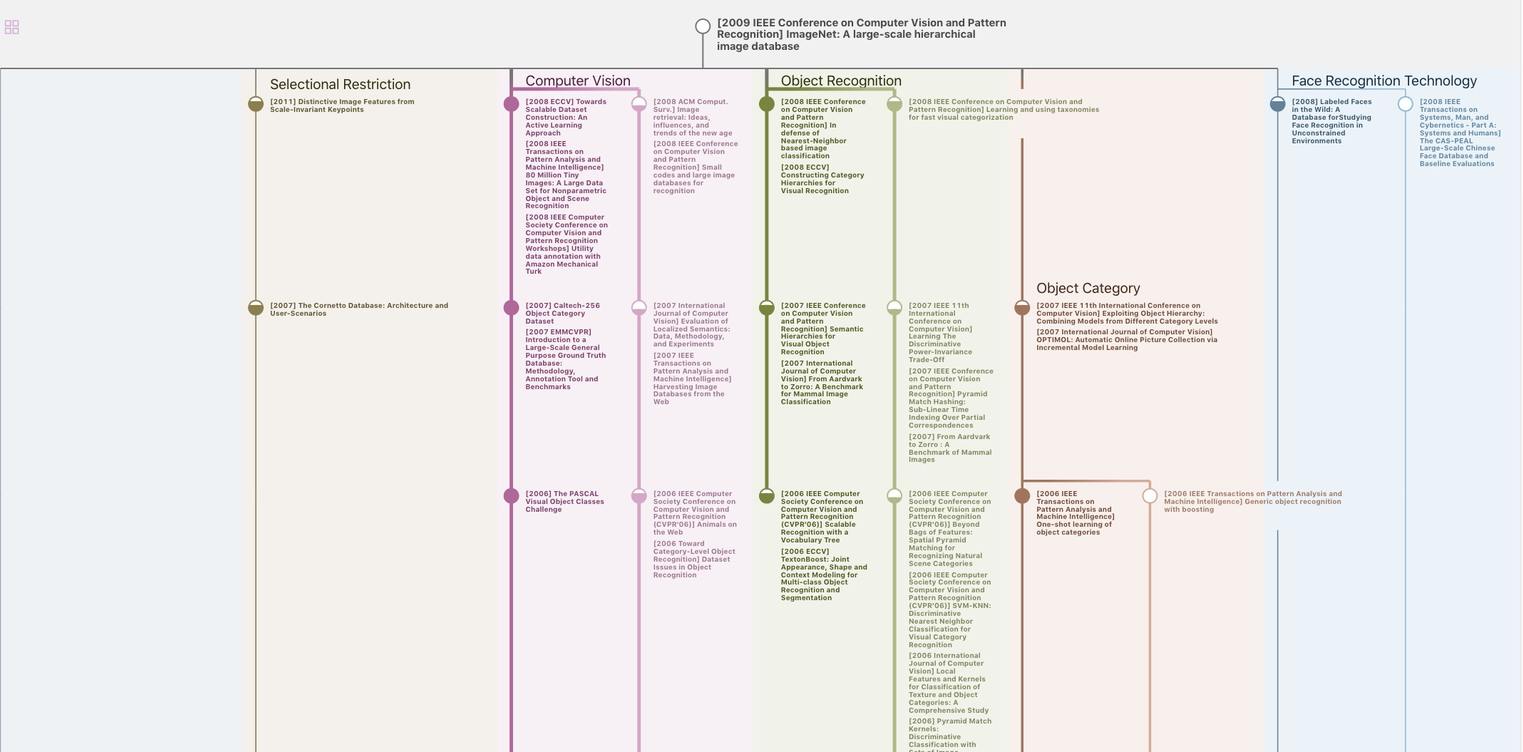
生成溯源树,研究论文发展脉络
Chat Paper
正在生成论文摘要