Hierarchical selection of genetic and gene by environment interaction effects in high-dimensional mixed models
arxiv(2023)
摘要
Interactions between genes and environmental factors may play a key role in
the etiology of many common disorders. Several regularized generalized linear
models (GLMs) have been proposed for hierarchical selection of gene by
environment interaction (GEI) effects, where a GEI effect is selected only if
the corresponding genetic main effect is also selected in the model. However,
none of these methods allow to include random effects to account for population
structure, subject relatedness and shared environmental exposure. In this
paper, we develop a unified approach based on regularized penalized
quasi-likelihood (PQL) estimation to perform hierarchical selection of GEI
effects in sparse regularized mixed models. We compare the selection and
prediction accuracy of our proposed model with existing methods through
simulations under the presence of population structure and shared environmental
exposure. We show that for all simulation scenarios, compared to other
penalized methods, our proposed method enforced sparsity by controlling the
number of false positives in the model while having the best predictive
performance. Finally, we apply our method to a real data application using the
Orofacial Pain: Prospective Evaluation and Risk Assessment (OPPERA) study, and
found that our method retrieves previously reported significant loci.
更多查看译文
AI 理解论文
溯源树
样例
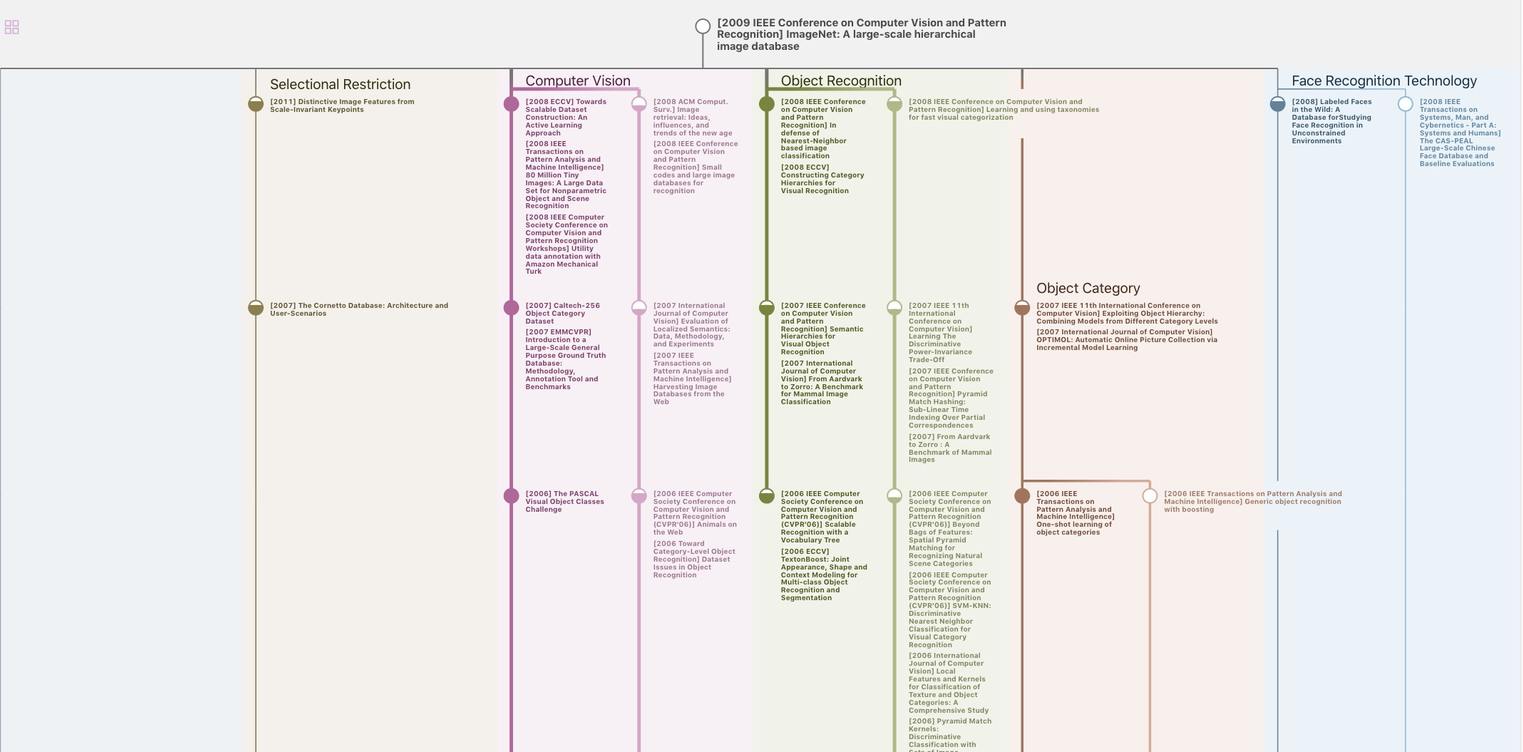
生成溯源树,研究论文发展脉络
Chat Paper
正在生成论文摘要