Random hierarchical model for estimation of wheat yield in the North China Plain at different spatial scales
FIELD CROPS RESEARCH(2024)
摘要
Accurate and large-scale wheat yield prediction in the North China Plain (NCP) can provide necessary information for agricultural policies and agricultural trade. Many studies have presented wheat yield estimation methods by using existing machine learning methods and remote sensing (RS) or environmental data. However, these methods only blindly input multi-source data into the model and do not consider the hierarchical relationships and interaction between different data types. In addition, there has been scant attention paid to the consistency of yield estimation models across varying spatial scales. To address these problems, a novel dynamic yield estimation model, known as random hierarchical model (RHM), which takes into account the hierarchical relationship of multi-source data, is constructed to estimate the wheat yield in the NCP. First, the time interval of wheat growth period is refined by using the 24 solar terms in China, and a time series multi-source dataset of climate, soil, and RS is constructed. Second, the hierarchical linear model is used to layer multi-source data and randomly select environmental and RS features with multiple time intervals. Multiple hierarchical models are constructed and optimized for integration, and the interrelationships between data collected at different levels are fully utilized, which can improve the accuracy of the yield estimation models in interannual and large-scale applications. Finally, the RHM at different spatial scales is cross-verified by using the measured and statistical data of the NCP for 4 years. The results indicated that the error of the RHM estimation is smaller than that of widely used machine learning models at different spatial scales of field-level measurement data (R2 = 0.52, nRMSE = 16.43%), county-level measurement integration data (R2 = 0.62, nRMSE = 12.83%), and county-level official statistics (R2 = 0.68, nRMSE = 11.41%). Our proposed RHM that considers the hierarchical structure of multi-source data is a reliable and a promising method for improving yield estimation. In addition, heterogeneity in the hierarchical relationships is observed between the different types of data in the RHM at different spatial scales, resulting in differences in the optimal lead time for estimating yield and the importance of key driving factors in the model, indicating that the cross-spatial scale applications of the model are not allowed. This study provides insights for large-scale wheat yield estimation and yield response to different environments and provided evidence and explanation for the prohibition of generalization of models at different spatial scales.
更多查看译文
关键词
Yield estimation,Remote sensing,Hierarchical model,Optimal lead time,Parameter importance
AI 理解论文
溯源树
样例
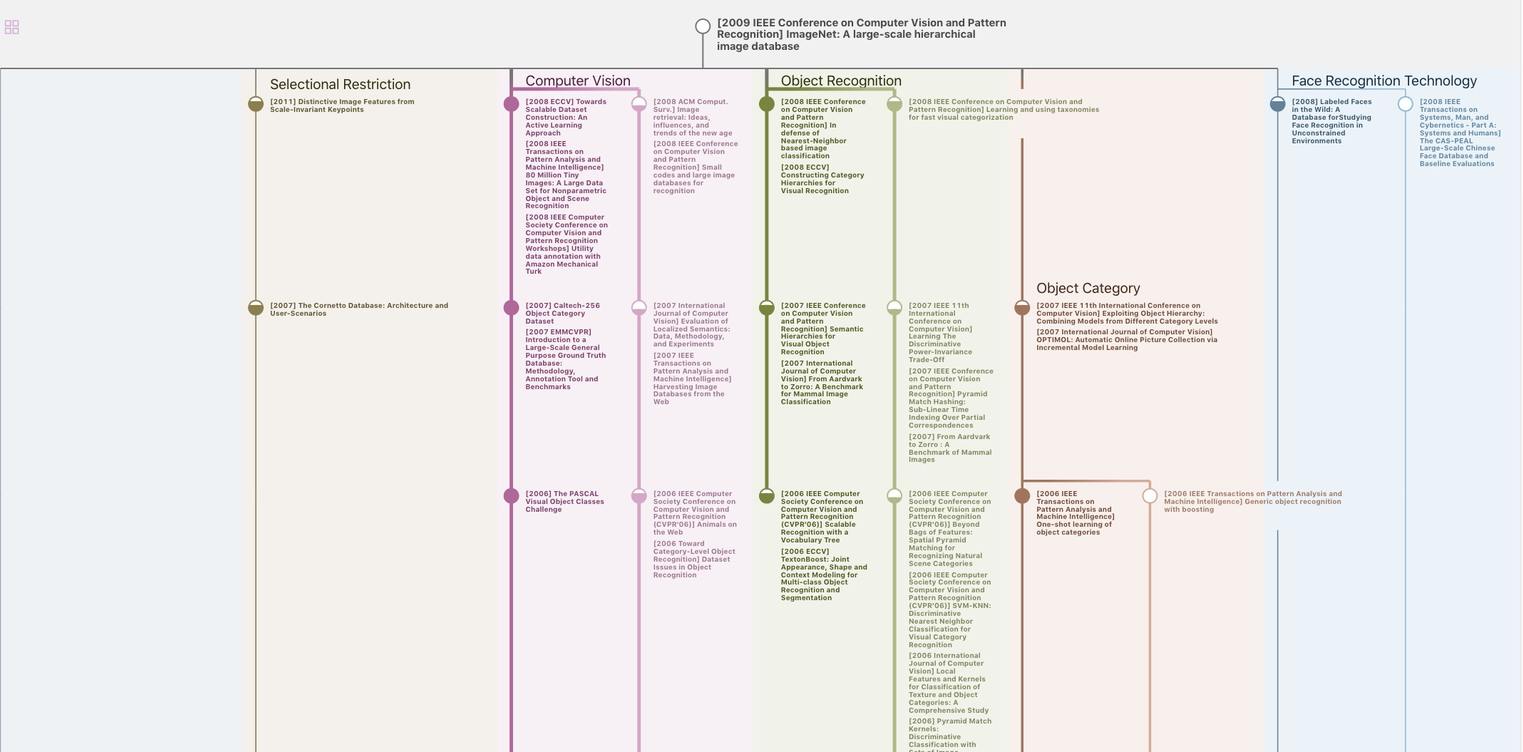
生成溯源树,研究论文发展脉络
Chat Paper
正在生成论文摘要