Multi-task label noise learning for classification
ENGINEERING APPLICATIONS OF ARTIFICIAL INTELLIGENCE(2024)
摘要
Multi-task classification improves generalization performance via exploiting the correlations between tasks. However, most multi-task learning methods fail to recognize and filter noisy labels for the classification problems with label noises. To address this issue, this paper proposes a novel multi-task label noise learning method based on loss correction, called MTLNL. MTLNL introduces the class-wise denoising (CWD) method for loss decomposition and centroid estimation of the loss function in multi-task learning, and eliminates the impact of label noise by using label flipping rate. It also extends to the multi-task positive-unlabeled (PU) learning domain, which offers better flexibility and generalization performance. Moreover, Nesterov's method is applied to accelerate the solution of the model. MTLNL is compared with other algorithms on five benchmark datasets, five image datasets, and a multi-task PU dataset to demonstrate its effectiveness.
更多查看译文
关键词
Multi-task learning,Label noise learning,Loss decomposition,Centroid estimation
AI 理解论文
溯源树
样例
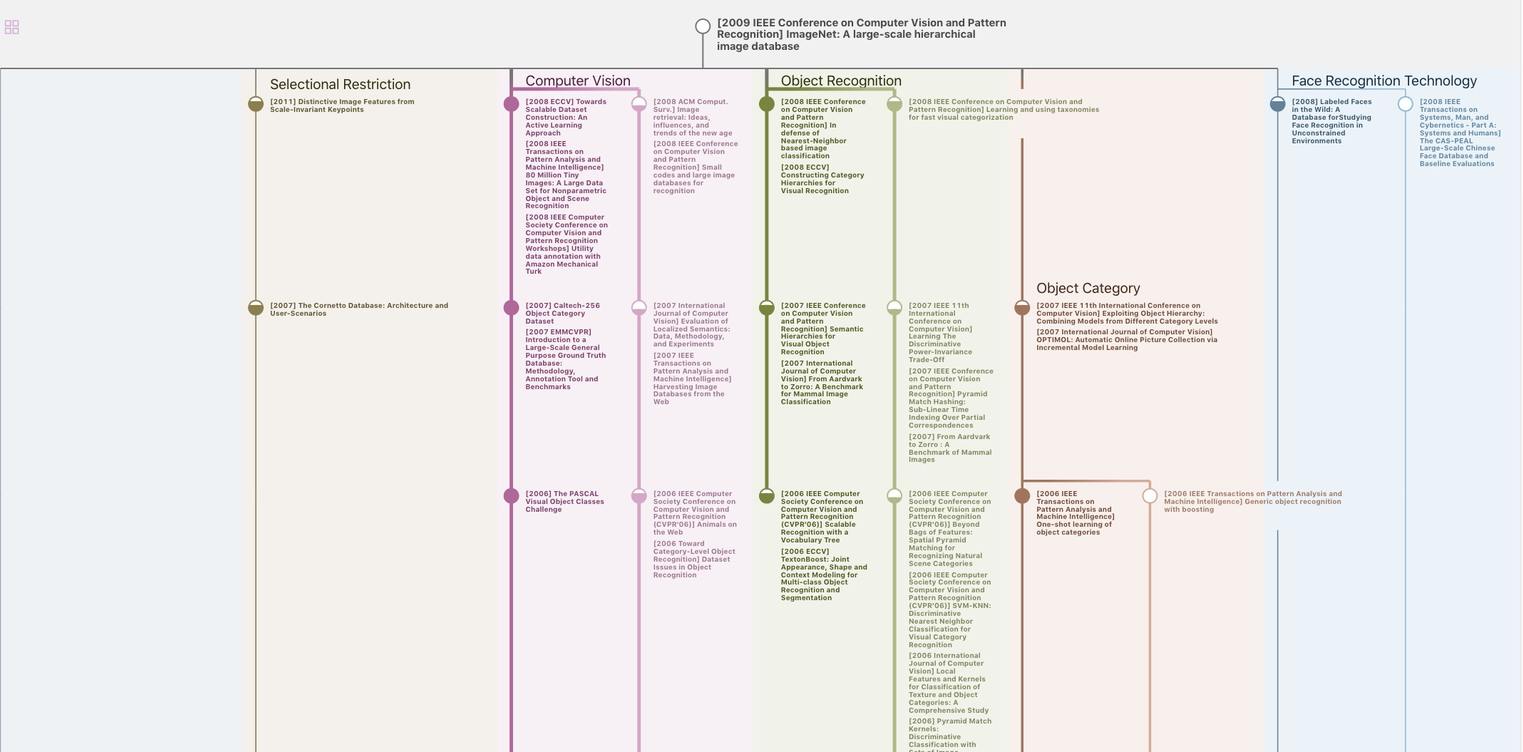
生成溯源树,研究论文发展脉络
Chat Paper
正在生成论文摘要