Heterogeneous graph knowledge distillation neural network incorporating multiple relations and cross-semantic interactions
INFORMATION SCIENCES(2024)
摘要
In recent years, the study of real-world graphs has revealed their inherent heterogeneity, prompting growing research interest in heterogeneous graphs. Characterized by diverse node and relation types, heterogeneous graphs have led to the development of heterogeneous graph neural networks, which possess the remarkable ability of modeling such heterogeneity. Consequently, researchers have embraced these networks, applying them in various domains. A prevalent approach is using meta-path based methods in heterogeneous graph neural networks. However, a significant limitation arises from the fact that such methods tend to overlook vital attribute information within intermediate nodes and disregard relevant semantics across various meta-paths. To address the above limitations, we propose a new model named HGNN-MRCS. Specifically, HGNN-MRCS incorporates three key components, i.e., a relation aware module to encapsulate the attribute information of the intermediate nodes; a meta-path aware technique to facilitate learning of semantic information of each meta-path and enable higher-order representation learning; and a knowledge distillation strategy to learn relevant semantics across meta-paths and fuse them. Experimental results on four real-world datasets demonstrate the superior performance of this work over the SOAT methods. The source codes of this work are available at https://github .com /ZZY -GraphMiningLab /HGNN-MRCS.
更多查看译文
关键词
Heterogeneous graph neural network,Meta-path,Knowledge distillation
AI 理解论文
溯源树
样例
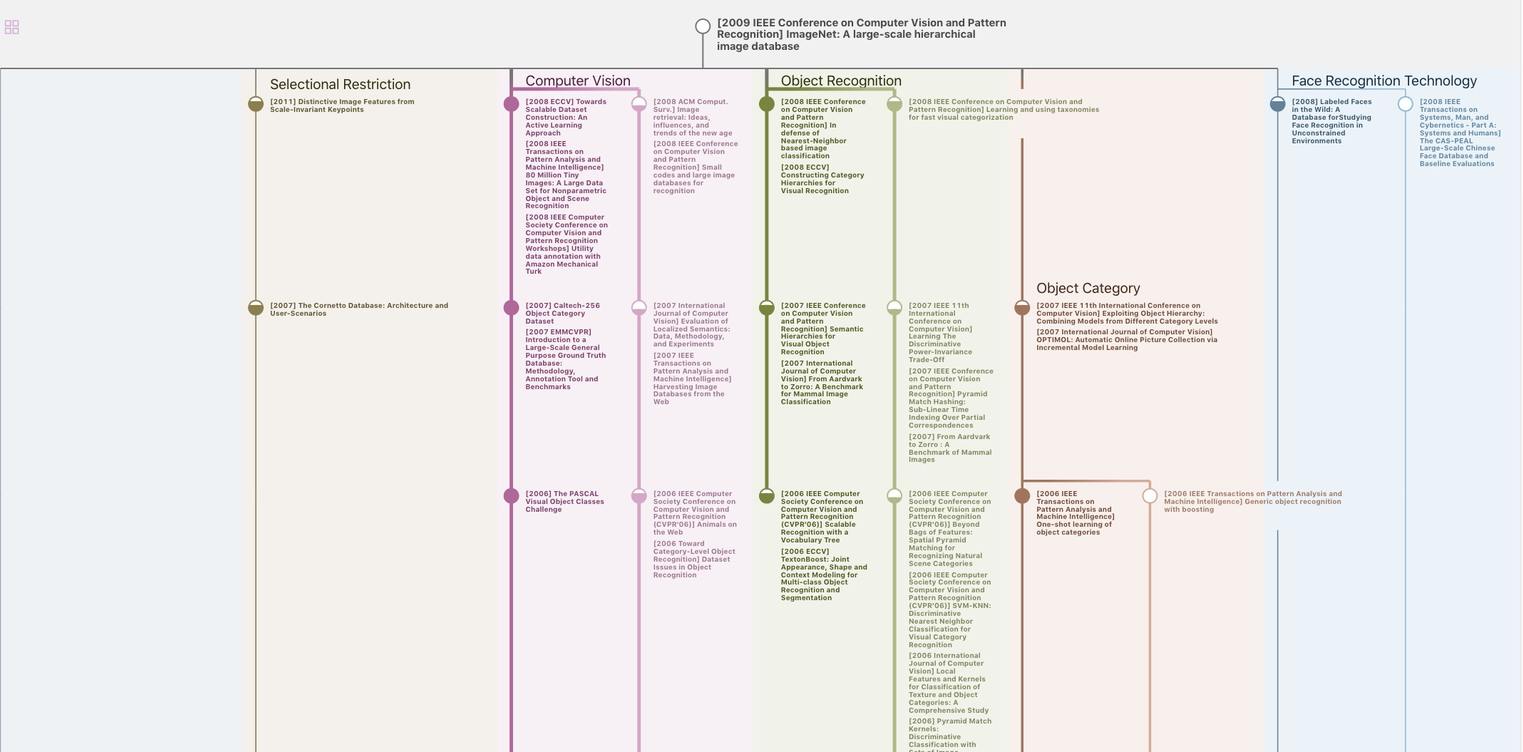
生成溯源树,研究论文发展脉络
Chat Paper
正在生成论文摘要