A novel reinforcement learning method for the plan of generator start-up after blackout
ELECTRIC POWER SYSTEMS RESEARCH(2024)
摘要
With the expansion of the power system and the impact of natural disasters, blackouts are almost inevitable. A scientific restoration plan can effectively shorten the economic and social losses caused by blackouts. As the first step of power system restoration, the generator start-up process impacts the success rate and time consumption. Considering the diversity of constraints in the generator start-up process and the complexity of the topology, this paper proposes a planning method for the generator start-up process based on reinforcement learning. Q-learning is a model-free reinforcement learning algorithm that can cope well with complex environments and diverse constraints. First, the generator start-up problem is modeled as a Markov Decision Process, and the restoration operation and constraints are considered. Q-learning uses an artificial intelligence agent to plan the generator start-up process and guide operators. Second, different from the restoration methods of sequential restarting the generators, this paper presents a novel strategy for restoration by restoring the path of the subsequent generator while the current generator acquires cranking power. Then, the action selection mechanism is optimized by using the Upper Confidence Bound algorithm to address the shortcoming of low exploration effectiveness in the conventional Q-learning. Finally, the simulation results provide evidence of the excellent performance of the proposed method in terms of optimality and convergence.
更多查看译文
关键词
Power system restoration,Generator start-up,Blackout,Q-learning,Upper Confidence Bound
AI 理解论文
溯源树
样例
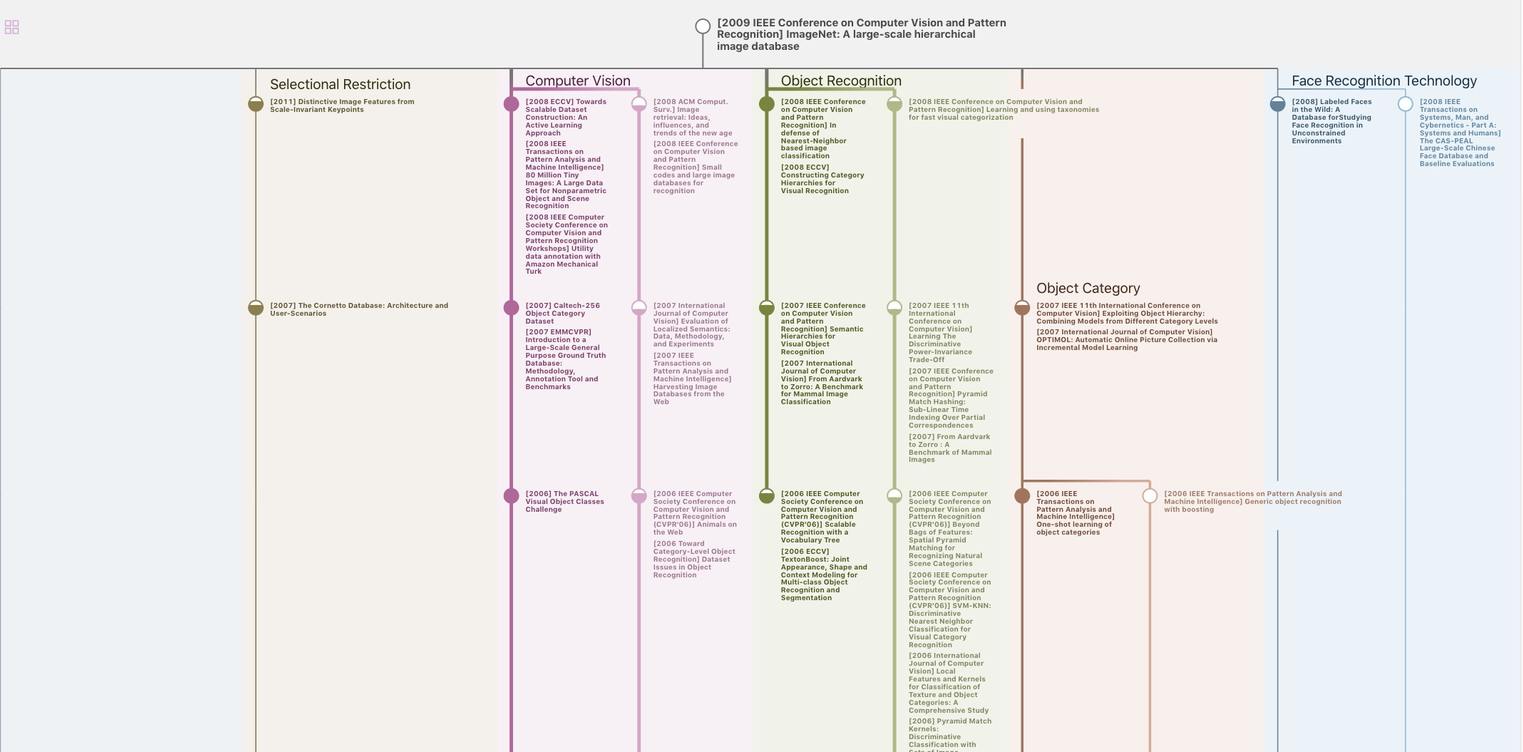
生成溯源树,研究论文发展脉络
Chat Paper
正在生成论文摘要