Intelligent active flow control of long-span bridge deck using deep reinforcement learning integrated transfer learning
JOURNAL OF WIND ENGINEERING AND INDUSTRIAL AERODYNAMICS(2024)
摘要
Aerodynamic forces of the Great Belt Bridge are mitigated using deep reinforcement learning (DRL) based active flow control (AFC) techniques at a Reynolds number of 5 x 105 in this study. The flow control method involves placing a suction slit at the bottom of the trailing edge of the bridge. The DRL agent as a controller is able to optimize the velocity of the suction to reduce the fluctuating coefficients of bending moment, drag, and lift by 99.1%, 73.7%, and 95.8% respectively. To reduce the high computational demands associated with DRL-based AFC training, this study integrates transfer learning technique into DRL, which calls transfer learning based deep reinforcement learning (TL-DRL) method. Specifically, the transfer learning method based on a DRL pre-trained model trained with a coarse mesh scheme is implemented. Results indicate that with TL-DRL method, the training cost can be reduced by 53%, while achieving the same control strategy and control effect as the DRL training from scratch. This study shows that the TL-DRL based flow control method is highly effective in reducing aerodynamic forces on long-span bridges. Furthermore, TL-DRL based flow control approach can adapt flexibly to different flow field environments, ultimately enhancing energy utilization efficiency.
更多查看译文
关键词
Deep reinforcement learning,Active flow control,Transfer learning,Aerodynamic force,Long-span bridge
AI 理解论文
溯源树
样例
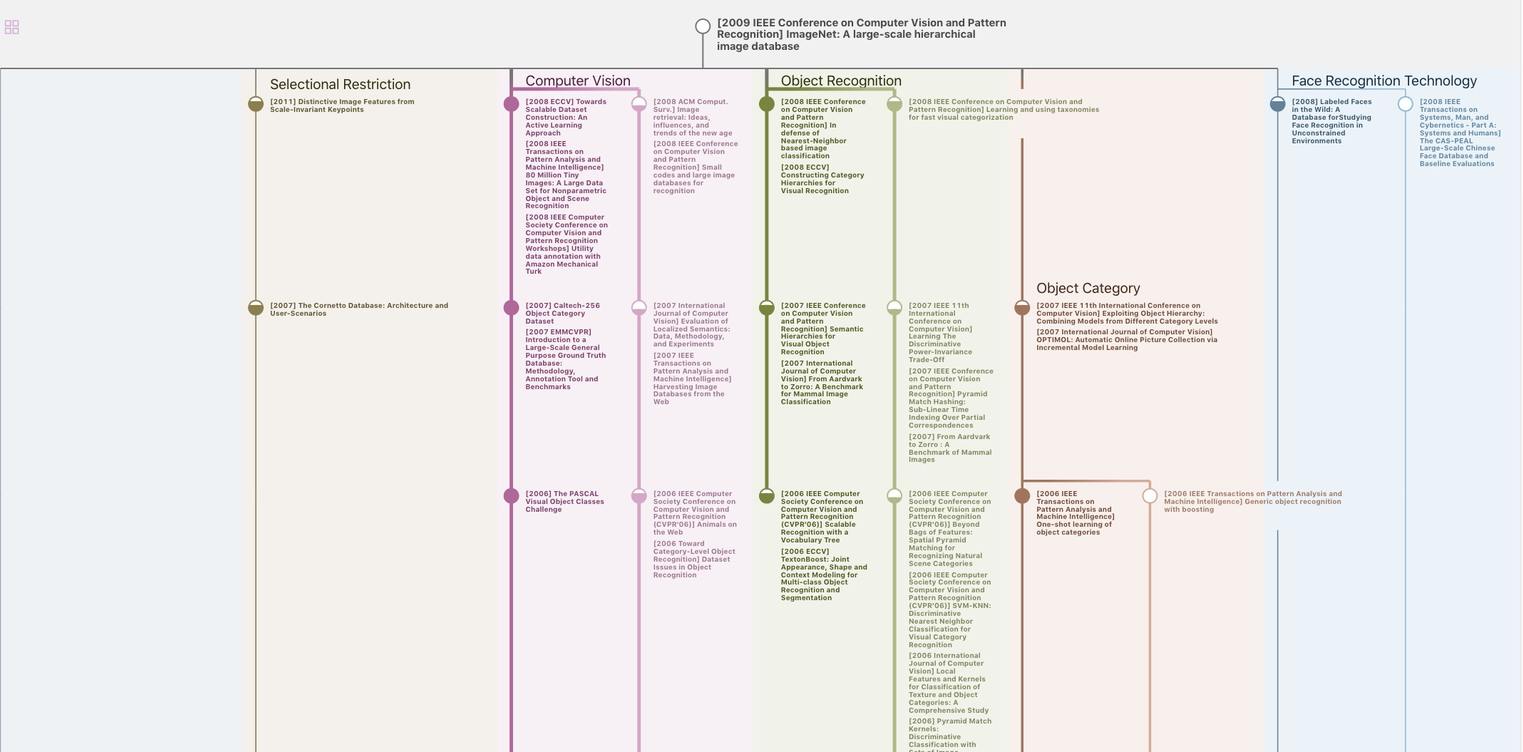
生成溯源树,研究论文发展脉络
Chat Paper
正在生成论文摘要