Federated Learning Aggregation based on Weight Distribution Analysis.
2023 IEEE International Conference on Imaging Systems and Techniques (IST)(2023)
摘要
Federated learning has recently been proposed as a solution to the problem of using private or sensitive data for training a central deep model, without exchanging the local data. In federated learning, local models are trained on the client side using the available data, while a server is responsible for aggregating the weights of these models into a global model. However, the traditional weight averaging approach does not take into consideration the importance of the different weights for the performance of a model. To this end, this work proposes a novel federated learning weight aggregation method that estimates the statistical distance of each client’s parameters from the Gaussianity, and weighs the contribution of each client to the global model accordingly so that the most significant information is retained and enhanced. To create an accurate global model, a complex weighted averaging of the parameters of clients’ models at the layer level is performed, considering as low quality the parameters following the Gaussian distribution. The proposed method can be employed to both convolutional and linear layers and it is based on the notion that parameters following a Gaussian distribution do not significantly affect the output of a model. Experiments with different network architectures and a comparison with a plethora of state-of-the-art approaches on three well-known image classification datasets demonstrate the superiority of the proposed method for federated learning weight aggregation.
更多查看译文
关键词
federated learning,Gaussian distribution,image classification
AI 理解论文
溯源树
样例
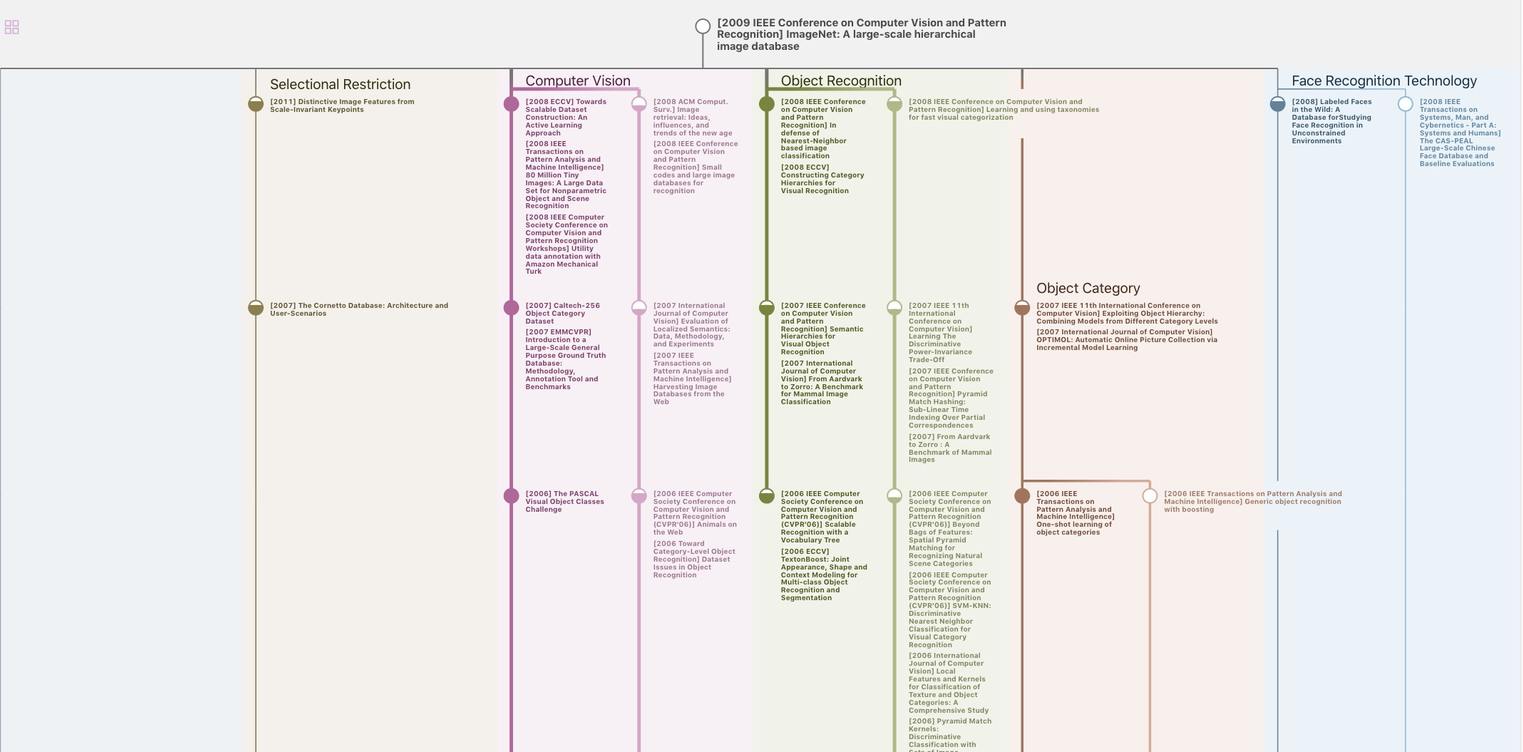
生成溯源树,研究论文发展脉络
Chat Paper
正在生成论文摘要