Deep Interaction Behavioral Feature Network for Click-Through Rate Prediction
2023 IEEE 35TH INTERNATIONAL CONFERENCE ON TOOLS WITH ARTIFICIAL INTELLIGENCE, ICTAI(2023)
摘要
Click-through rate prediction(CTR) is a critical task in recommendation system. Most previous work on modelling the interaction features between user history behavior and target item focused only on mining the single interaction feature between user's single historical behavior and the target item, or only modeling the user behavior sequence to capture user interest, which may lead to lower prediction accuracy. To solve the above problem, we propose the Deep Interaction Behavioral Feature Network(DIBFN), which is a dual-module approach that focuses on both the interaction features of user interest and the target item and on the interaction features of user's single historical behavior and the target item. First, the Evolving Interaction Module (EIM), which processes user behavior sequence to explore the user interest over time, captures the interaction features of the user interest with the target item. Then, the Behavioral Feature Module (BFM) is proposed to learn behavior representations from multi-space through multi-head self-attention and use a two-layer attention mechanism to mine the interaction features between user's single historical behavior and the target item. Experimental results on real datasets show that the proposed DIBFN model outperforms previous main-stream approaches.
更多查看译文
关键词
CTR Prediction,Recommender systems,Neural Networks
AI 理解论文
溯源树
样例
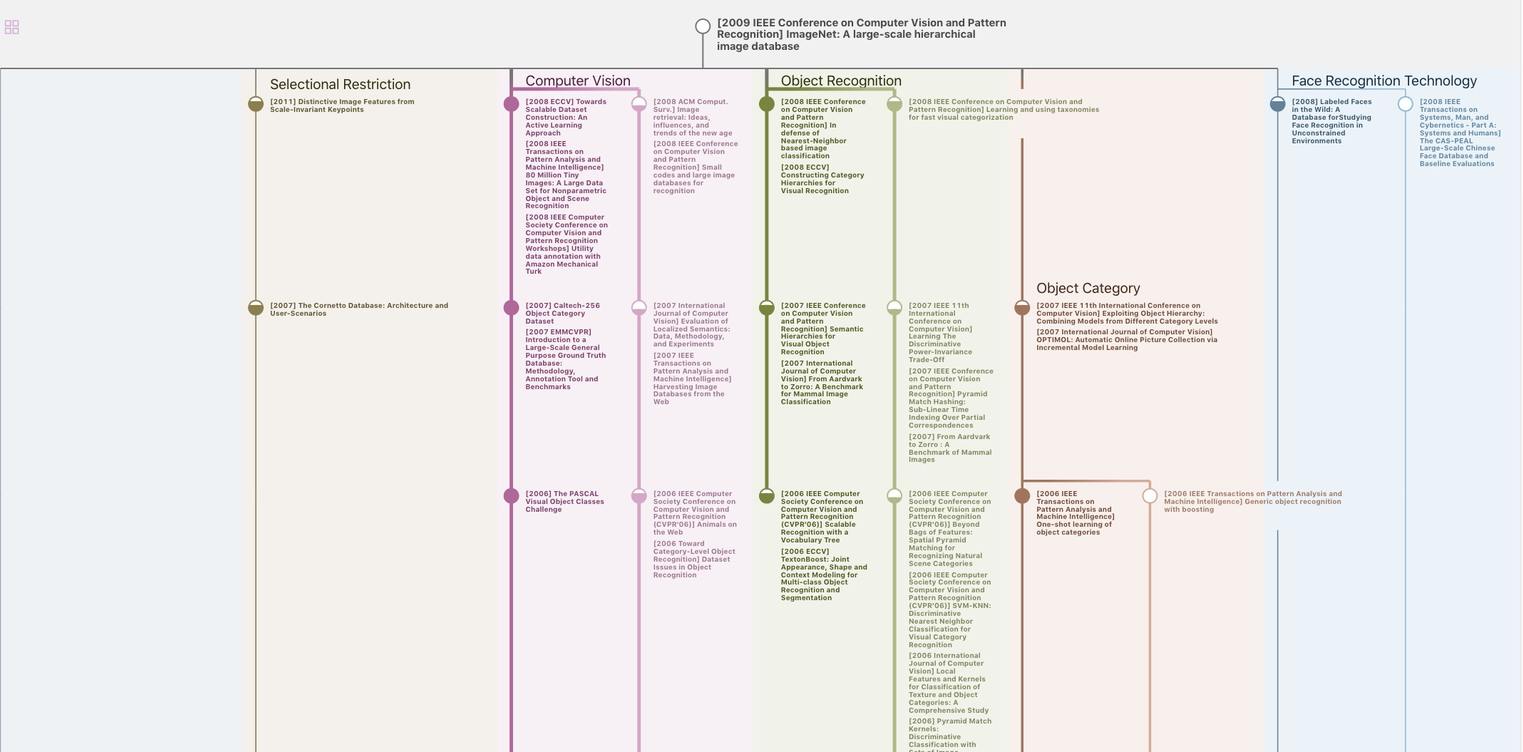
生成溯源树,研究论文发展脉络
Chat Paper
正在生成论文摘要