Environmental Knowledge-Driven Over-the-Horizon Propagation Loss Prediction Based on Short- and Long-Term Parallel Double-Flow TrellisNets
IEEE TRANSACTIONS ON ANTENNAS AND PROPAGATION(2023)
摘要
Accurate perceptual knowledge of atmospheric characteristics in the propagation path is of great significance for the design of communication systems. However, the atmosphere above the ocean is inhomogeneous, which brings challenges to accurate prediction of propagation loss. Moreover, the atmospheric refractive index distribution model calculated from atmospheric data requires at least two stations with near-sea meteorological data. In real maritime over-the-horizon communication or detection, only one station transmits and receives meteorological data, and the received meteorological data and propagation loss have large temporal noise. To address these issues, first, a denoising model based on the 1-D convolution autoencoder (1-DCAE) is constructed to filter out the temporal noise of input environmental meteorological data and propagation loss. Second, to accurately predict the influence of environmental factors on the prediction of propagation loss, a deep-learning framework called short- and long-term parallel double-flow TrellisNets (SL-TrellisNets) is proposed to predict the loss. Finally, the extensive experiments demonstrated that the root mean square and mean absolute errors (MAEs) of the proposed 1-DCAE are dramatically reduced. Our proposed SL-TrellisNets outperforms the other state-of-the-art techniques in terms of propagation loss prediction. In addition, we analyzed the impact of the environmental factors on the accuracy of over-the-horizon propagation loss (OHPL) prediction.
更多查看译文
关键词
1-D convolutional,autoencoder,environmental knowledge-driven,over-the-horizon propagation loss (OHPL) prediction,SL-TrellisNets
AI 理解论文
溯源树
样例
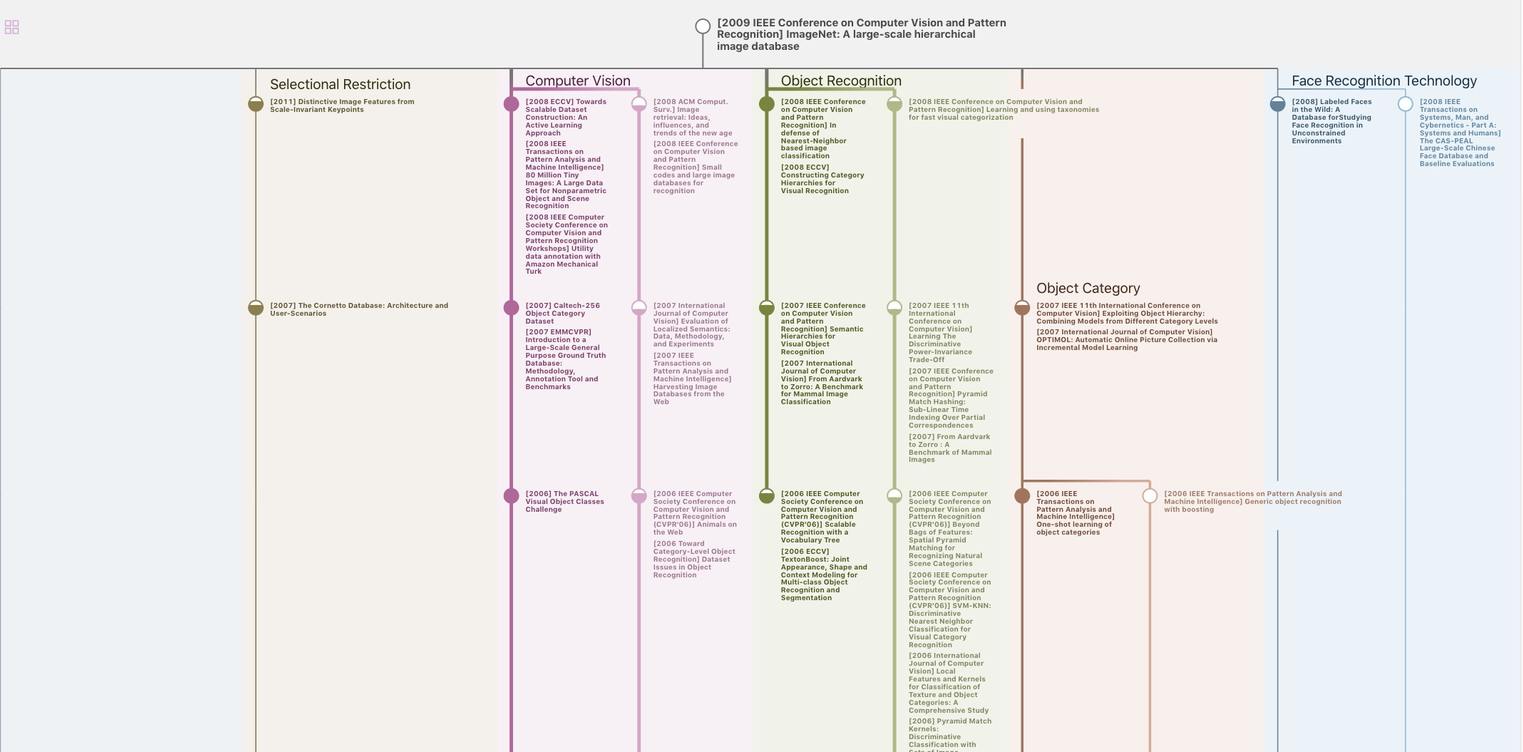
生成溯源树,研究论文发展脉络
Chat Paper
正在生成论文摘要