SFGT-CD: Semantic Feature-Guided Building Change Detection From Bitemporal Remote-Sensing Images With Transformers
IEEE GEOSCIENCE AND REMOTE SENSING LETTERS(2024)
摘要
High-resolution remote-sensing-image change detection is widely used in urban dynamic monitoring, geographic information updating, natural disaster monitoring, illegal building investigation, and land resource surveys. Common change-detection algorithms are mainly implemented in a fully supervised manner that relies on a large number of high-quality samples. Compared with a building change-detection dataset, a building semantic-segmentation dataset is easier to accumulate and obtain. Making full use of this semantic information in the design of a building change-detection network can effectively reduce the sample size required to train a change-detection model. In view of this, a semantic feature-guided Siamese change-detection framework is devised in this letter. The framework effectively exploits the prior information of building semantic features and uses the popular transformer structure to improve the change analysis module. The results of extensive experiments on two public datasets show that the framework is more accurate than the other state-of-the-art change detection algorithms and can effectively reduce the dependence of data on change detection samples in the model training process.
更多查看译文
关键词
Feature extraction,Semantics,Transformers,Convolutional neural networks,Training,Remote sensing,Decoding,Change detection,high-resolution optical remote-sensing images,prior semantic information,transformers
AI 理解论文
溯源树
样例
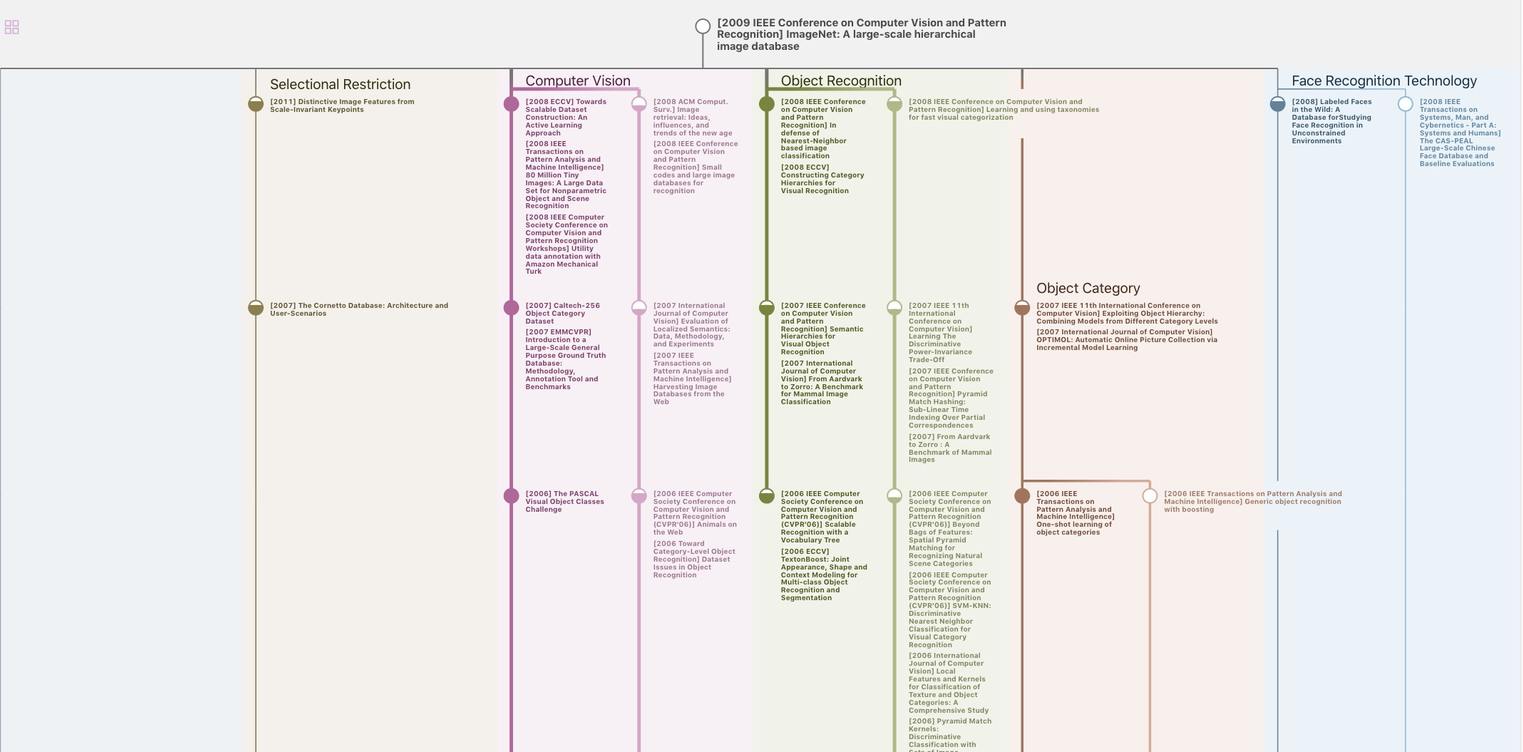
生成溯源树,研究论文发展脉络
Chat Paper
正在生成论文摘要