Dense Dual-Branch Cross Attention Network for Semantic Segmentation of Large-Scale Point Clouds
IEEE TRANSACTIONS ON GEOSCIENCE AND REMOTE SENSING(2024)
摘要
Semantic segmentation of large-scale point clouds provides foundational knowledge for various geodetic and cartographic applications, including autonomous driving, smart cities, and indoor navigation. However, point cloud data's unstructured and inherently disordered characteristics pose challenges in extracting accurate 3-D semantic information. In this study, we introduce a novel semantic segmentation network for large-scale point cloud scenes, referred to as dense dual-branch cross attention network (D2CAN). We propose a local multidimensional feature aggregation (LMFA) module to increase multidimensional feature representation types and preserve rich local details. Based on the augmented local features, an expanded dual-branch cross attention (EDCA) module establishes internal deep connections between multidimensional attributes and semantic features. This assists the network in reducing boundary ambiguities and expanding the receptive field, enabling the parallel capture of long-range contexts specifically adapted for large-scale scene point cloud segmentation. These two modules work collaboratively to constitute a local context deep perception (LCDP) block. To reduce information loss during feature sampling and propagation, we propose a global feature pyramid dense fusion (GFDF) block. This block adaptively integrates features across different scales and effectively captures global context with long-range dependencies. In conclusion, D2CAN combines LCDP and GFDF to aggregate both local and global contexts, resulting in robust feature discrimination for semantic segmentation of large-scale scenes. Our method's effectiveness and superior generation ability have been validated across three challenging benchmarks and achieve state-of-the-art performance on Toronto-3D, SensatUrban, and Stanford large-scale 3-D indoor spaces (S3DIS) datasets, with mean intersection over union (IoU) values of 83.5%, 61.1%, and 72.3%, respectively.
更多查看译文
关键词
Attention mechanism,deep learning,large-scale point clouds,multiscale fusion,point cloud segmentation,semantic segmentation
AI 理解论文
溯源树
样例
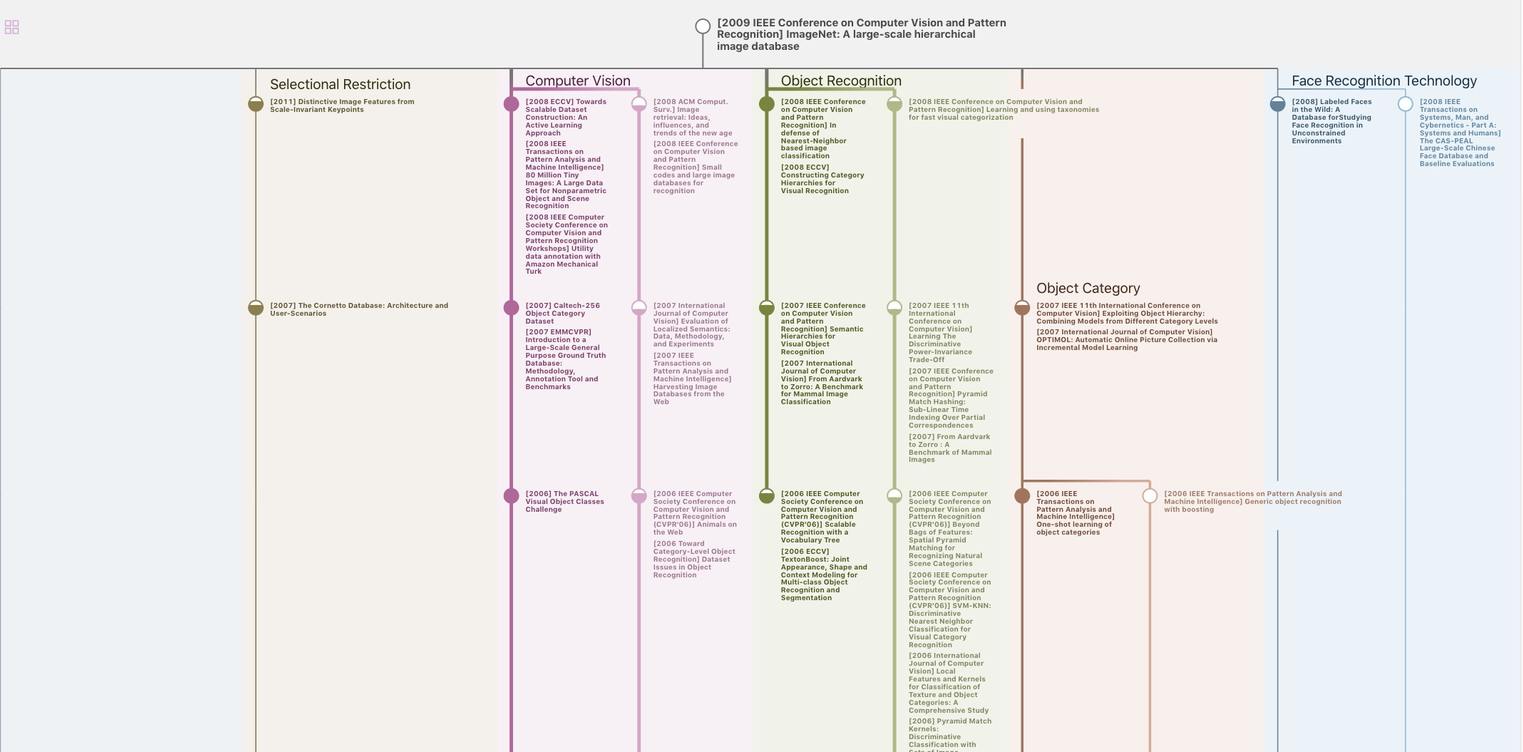
生成溯源树,研究论文发展脉络
Chat Paper
正在生成论文摘要