Fuzzy rough unlearning model for feature selection
INTERNATIONAL JOURNAL OF APPROXIMATE REASONING(2024)
摘要
In big data era, some data, becoming meaningless or illegal over time and space, need to be deleted from historical knowledge. It is a challenging problem, called machine unlearning, to efficiently forget the information of those outdated data from historical models. Some unlearning techniques have been proposed in loss-well-defined classification models, such as SVM, Random Forest, and Federated learning model. Yet, it is under study to remove outdated data from learned feature selection in fuzzy rough philosophy. To narrow this gap, we propose a fuzzy rough unlearning model for feature selection. Specifically, the outdated information is first identified in a compact set, called unlearning set, that remarkably shrinks the search space of feature selection. Then, the unlearning mechanisms containing two main theorems are proposed by leveraging unlearning set, followed by a feature selection unlearning algorithm. Theoretical analyses verify that the proposed unlearning algorithm is equivalent to the traditional algorithm retraining on the remaining data with the beginning of historical results. Experimentally, extensive results on 20 datasets demonstrate that our proposed unlearning methods perform effectively with remarkably less time cost. For the source code of ARU, please refer to: https://github.com/ yuxin370/ARU.
更多查看译文
关键词
Fuzzy rough sets,Unlearning,Feature selection,Positive region
AI 理解论文
溯源树
样例
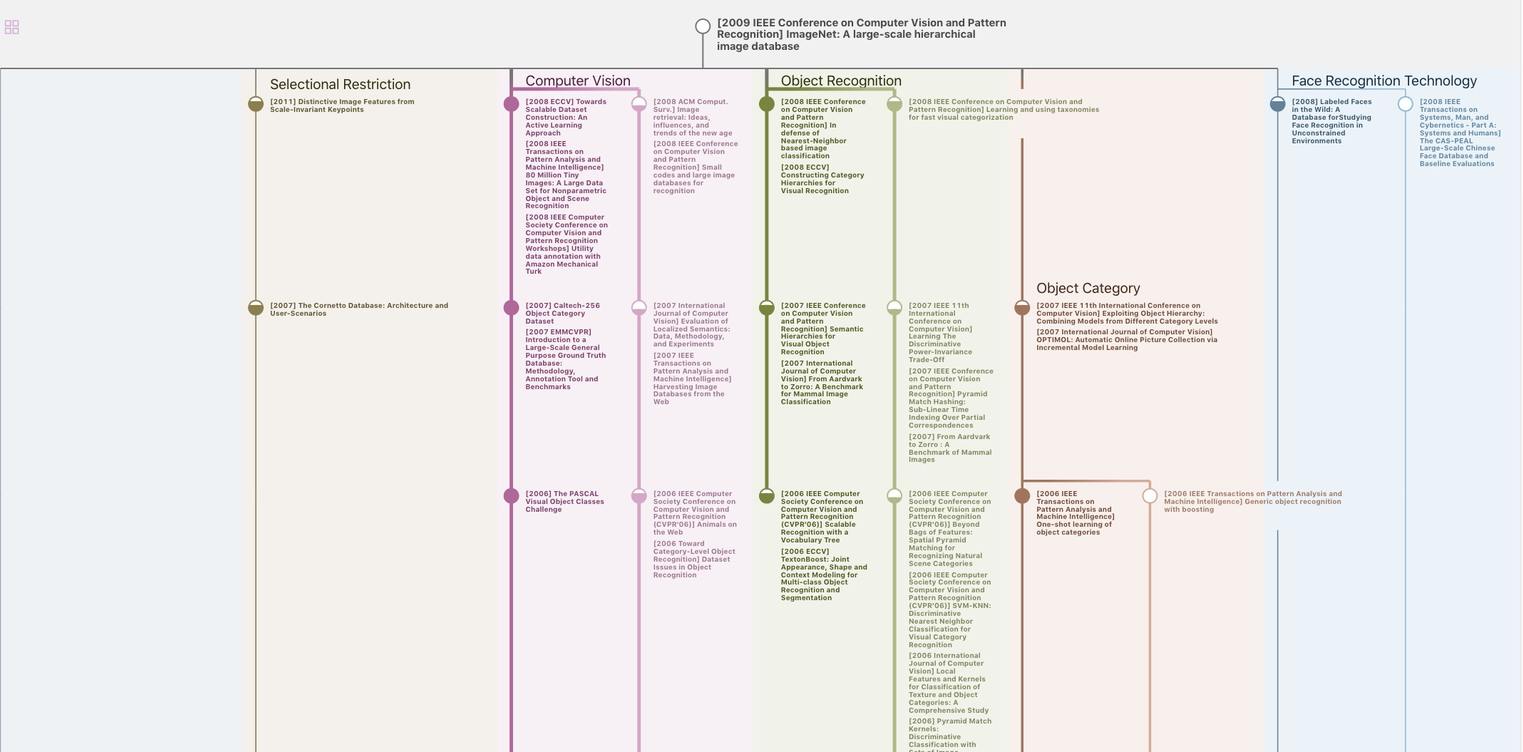
生成溯源树,研究论文发展脉络
Chat Paper
正在生成论文摘要