Discovery Precision: An effective metric for evaluating performance of machine learning model for explorative materials discovery
COMPUTATIONAL MATERIALS SCIENCE(2024)
摘要
The evaluation of machine learning (ML) models in identifying novel materials with superior Figure of Merit (FOM) compared to known materials is of utmost importance for exploring their potential applications. A welldesigned evaluation should distinguish between good and poor models, avoid bias in the validation and testing set, and offer straightforward interpretable. To fulfill these requirements, a novel metric called Discovery Precision (DP) was proposed to assess the efficiency of ML models for material discovery in terms of probability. To validate the applicability of DP, we have established a comprehensive testing framework for comparing various validation methods and metrics. Through this framework, the DP metric demonstrates excellent performance in material discovery. Additionally, the simulation results indicate that DP effectively assists in model selection and provides an unbiased estimation of model performance.
更多查看译文
关键词
Materia l discovery,Machine learning,Model validation,Extrapolation,Performance evaluation
AI 理解论文
溯源树
样例
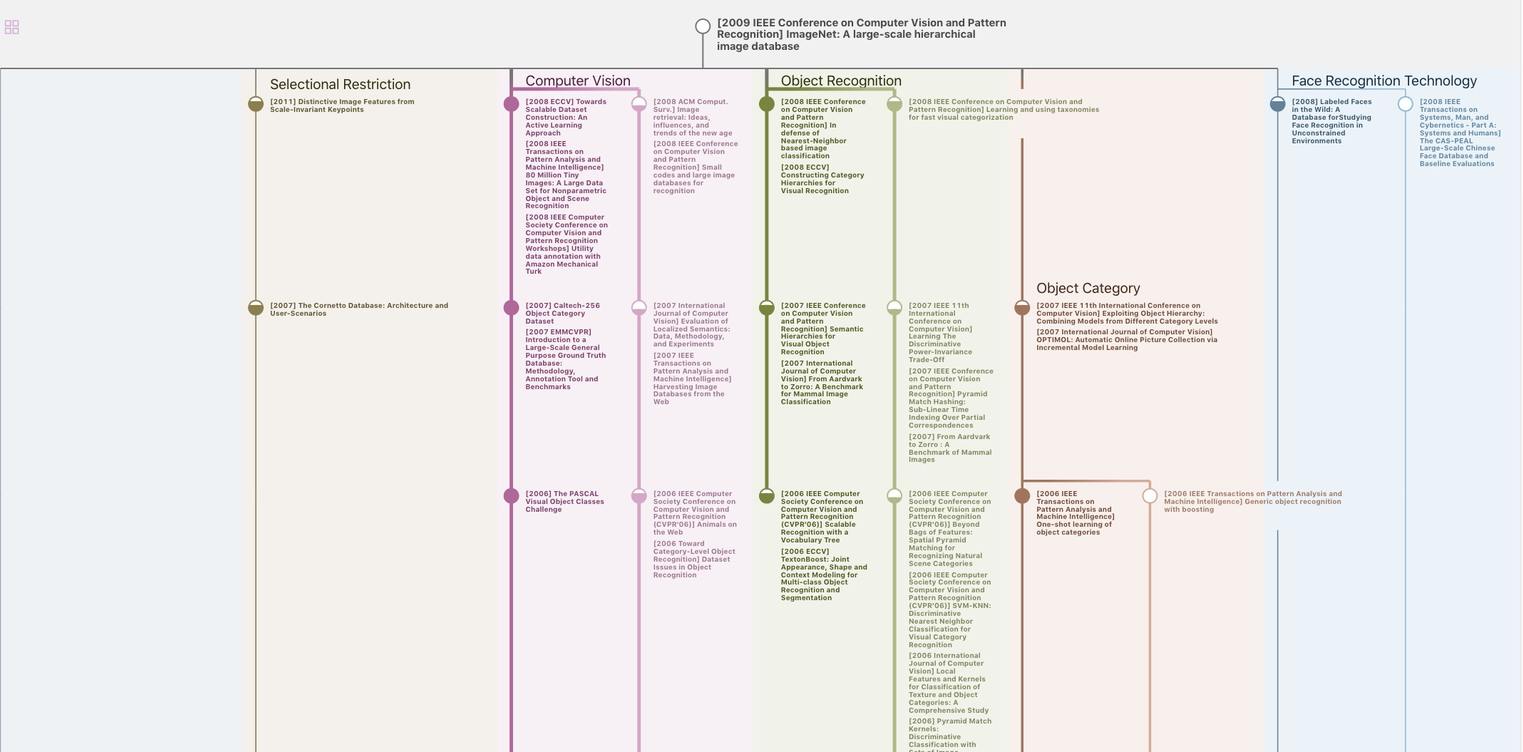
生成溯源树,研究论文发展脉络
Chat Paper
正在生成论文摘要