Deep Causality Variational Autoencoder Network for Identifying the Potential Biomarkers of Brain Disorders
IEEE TRANSACTIONS ON NEURAL SYSTEMS AND REHABILITATION ENGINEERING(2024)
摘要
Identifying causality from observational time-series data is a key problem in dealing with complex dynamic systems. Inferring the direction of connection between brain regions (i.e., causality) has become the central topic in the domain of fMRI. The purpose of this study is to obtain causal graphs that characterize the causal relationship between brain regions based on time series data. To address this issue, we designed a novel model named deep causal variational autoencoder (CVAE) to estimate the causal relationship between brain regions. This network contains a causal layer that can estimate the causal relationship between different brain regions directly. Compared with previous approaches, our method relaxes many constraints on the structure of underlying causal graph. Our proposed method achieves excellent performance on both the Alzheimer's Disease Neuroimaging Initiative (ADNI) and the Autism Brain Imaging Data Exchange 1 (ABIDE1) databases. Moreover, the experimental results show that deep CVAE has promising applications in the field of brain disease identification.
更多查看译文
关键词
Causal inference,fMRI,autoencoder,Alzheimer's disease (AD),autism spectrum disorder (ASD)
AI 理解论文
溯源树
样例
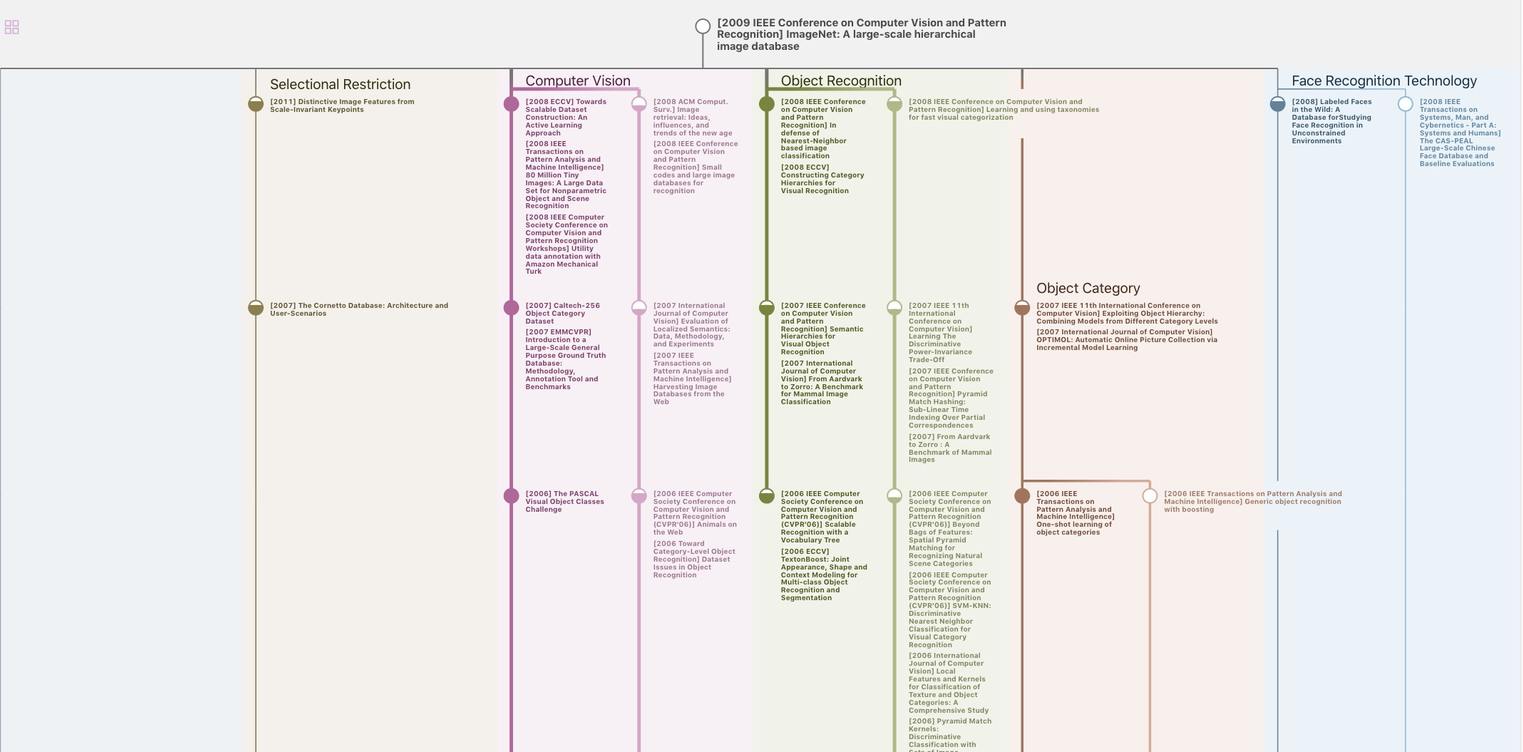
生成溯源树,研究论文发展脉络
Chat Paper
正在生成论文摘要