FedA3I: Annotation Quality-Aware Aggregation for Federated Medical Image Segmentation against Heterogeneous Annotation Noise
AAAI 2024(2024)
摘要
Federated learning (FL) has emerged as a promising paradigm for training segmentation models on decentralized medical data, owing to its privacy-preserving property. However, existing research overlooks the prevalent annotation noise encountered in real-world medical datasets, which limits the performance ceilings of FL. In this paper, we, for the first time, identify and tackle this problem. For problem formulation, we propose a contour evolution for modeling non-independent and identically distributed (Non-IID) noise across pixels within each client and then extend it to the case of multi-source data to form a heterogeneous noise model (i.e., Non-IID annotation noise across clients). For robust learning from annotations with such two-level Non-IID noise, we emphasize the importance of data quality in model aggregation, allowing high-quality clients to have a greater impact on FL. To achieve this, we propose Federated learning with Annotation quAlity-aware AggregatIon, named FedA3I, by introducing a quality factor based on client-wise noise estimation. Specifically, noise estimation at each client is accomplished through the Gaussian mixture model and then incorporated into model aggregation in a layer-wise manner to up-weight high-quality clients. Extensive experiments on two real-world medical image segmentation datasets demonstrate the superior performance of FedA3I against the state-of-the-art approaches in dealing with cross-client annotation noise. The code is available at https://github.com/wnn2000/FedAAAI.
更多查看译文
关键词
ML: Distributed Machine Learning & Federated Learning,CV: Medical and Biological Imaging,CV: Segmentation,ML: Calibration & Uncertainty Quantification
AI 理解论文
溯源树
样例
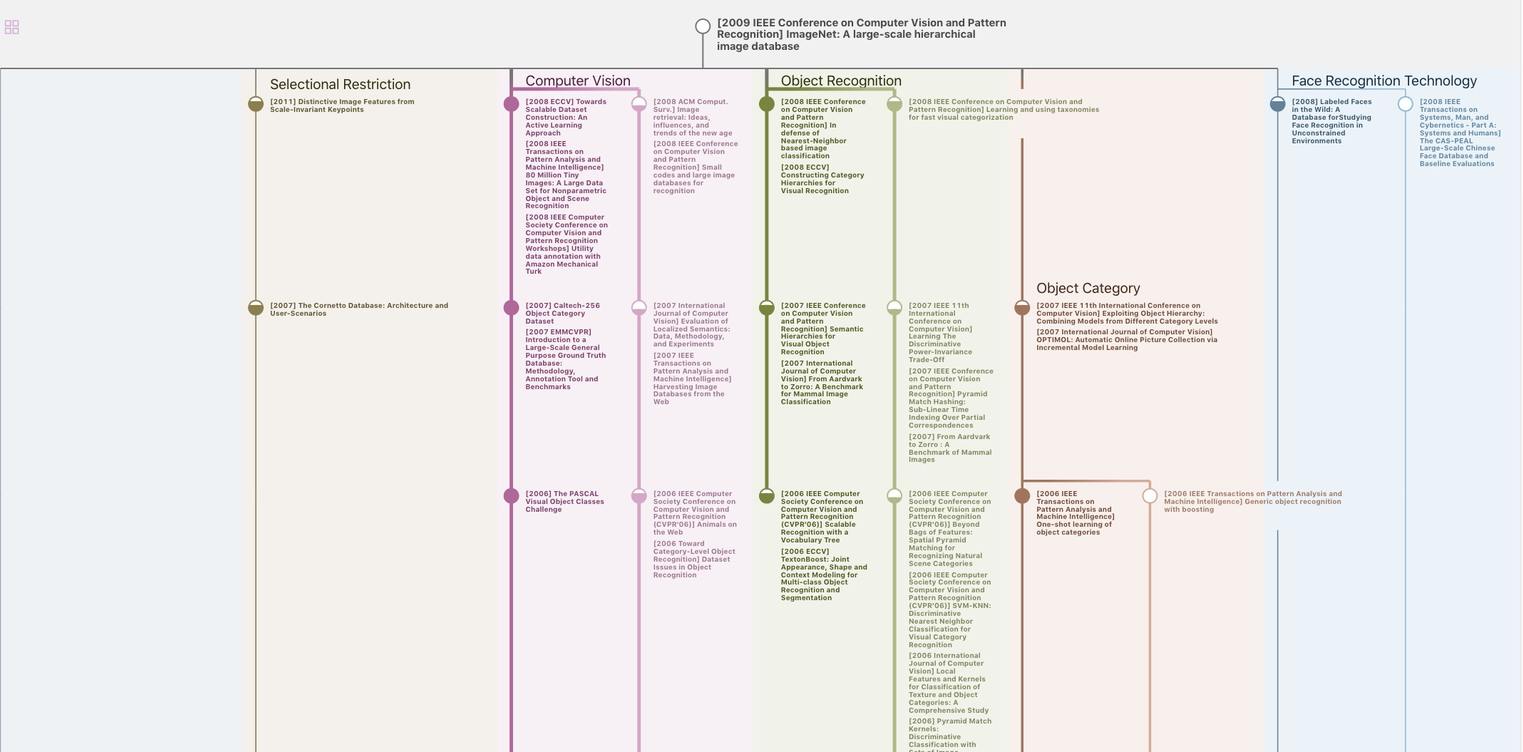
生成溯源树,研究论文发展脉络
Chat Paper
正在生成论文摘要