Backpropagation and gradient descent for an optimized dynamic mode decomposition
arxiv(2023)
摘要
We present a robust and flexible optimization approach for dynamic mode
decomposition analysis of data with complex dynamics and low signal-to-noise
ratios. The approach borrows techniques and insights from the field of deep
learning. Specifically, we employ automatic differentiation and stochastic
gradient descent to compute eigenvalues, modes, and mode amplitudes
simultaneously. The method allows embedding regularization or physical
constraints into the operator definition. The optimization approach is applied
to three examples of increasing complexity, the most challenging of which is an
experimental dataset of transonic shock buffet on a swept at realistic flight
conditions.
更多查看译文
AI 理解论文
溯源树
样例
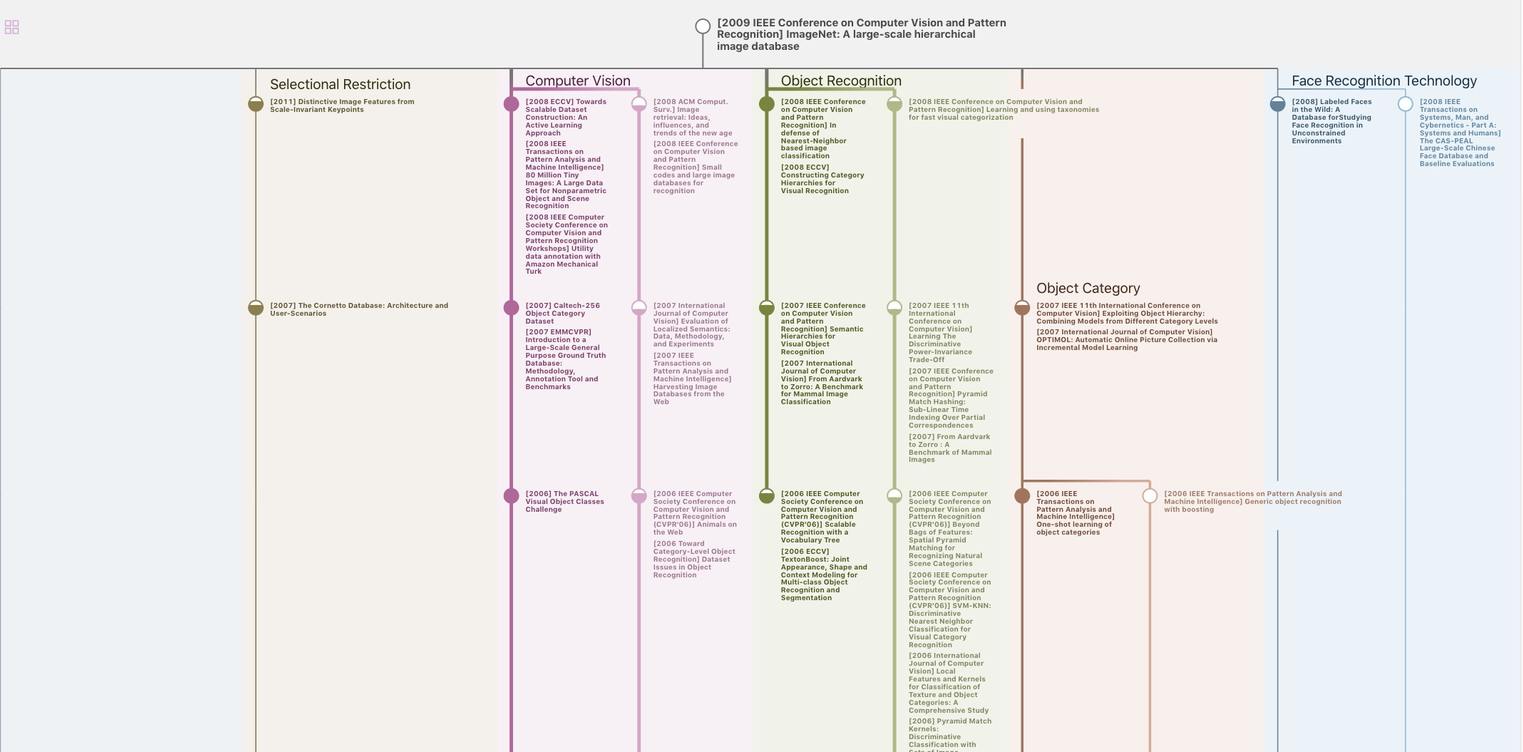
生成溯源树,研究论文发展脉络
Chat Paper
正在生成论文摘要