Spatial correlation-based machine learning framework for evaluating shale gas production potential: A case study in southern Sichuan Basin, China
APPLIED ENERGY(2024)
摘要
Assessment of production potential and prediction of sweet spots in unexploited shale gas wells are crucial technologies for achieving a high success rate in drilling. Most existing methods are only applicable for yield forecasting of shale gas wells with known geological and drilling/completion factors after production. In addition, the shale gas reservoir in the Longmaxi Formation in the southern Sichuan Basin of China is characterized by low porosity, low permeability, and diverse gas reservoir states. Thus, forecasting production from unexploited wells and predicting the exact location of the sweet spot under complex geological conditions have become challenging topic for both academia and industry. According to the first law of geography, shale gas wells that are closer in space have more similar geologic features. Based on this foundation, a machine-learning prediction model based on spatial correlation (SC-MLPM) is proposed for evaluating potential production of shale gas wells in the Changning play located in Longmaxi Formation in the southern Sichuan basin, China. Specifically, an improved K-nearest neighbor algorithm (SC-KNN) based on the spatial correlation characteristics of the exploited wells is designed to estimate the relevant geological factors of the unexploited wells in the same area. In addition, an extreme gradient lifting model based on Huber loss function (Hu-XGB) is developed to predict the potential production of unexploited shale gas wells, and then the shale gas sweet spot area in the research area is predicted quickly and accurately. The experimental results on 88 shale gas wells from Changning play show that the mean relative error of the prediction results of the proposed method is 9.8%. Furthermore, the results also determine that the sweet spots of shale gas wells in the research area are mainly developed in the northwest and northeast directions, and gradually become worse in the southeast direction. Eventually, experimental results for the Fox Creek play in Canada show that the proposed model obtains better predictive performance as the number of gas wells increases, and also verifies the generalizability. This work provides a basis for the efficient development of the research area.
更多查看译文
关键词
Machine learning,Spatial correlation,Shale gas,Production potential,Sweet spot
AI 理解论文
溯源树
样例
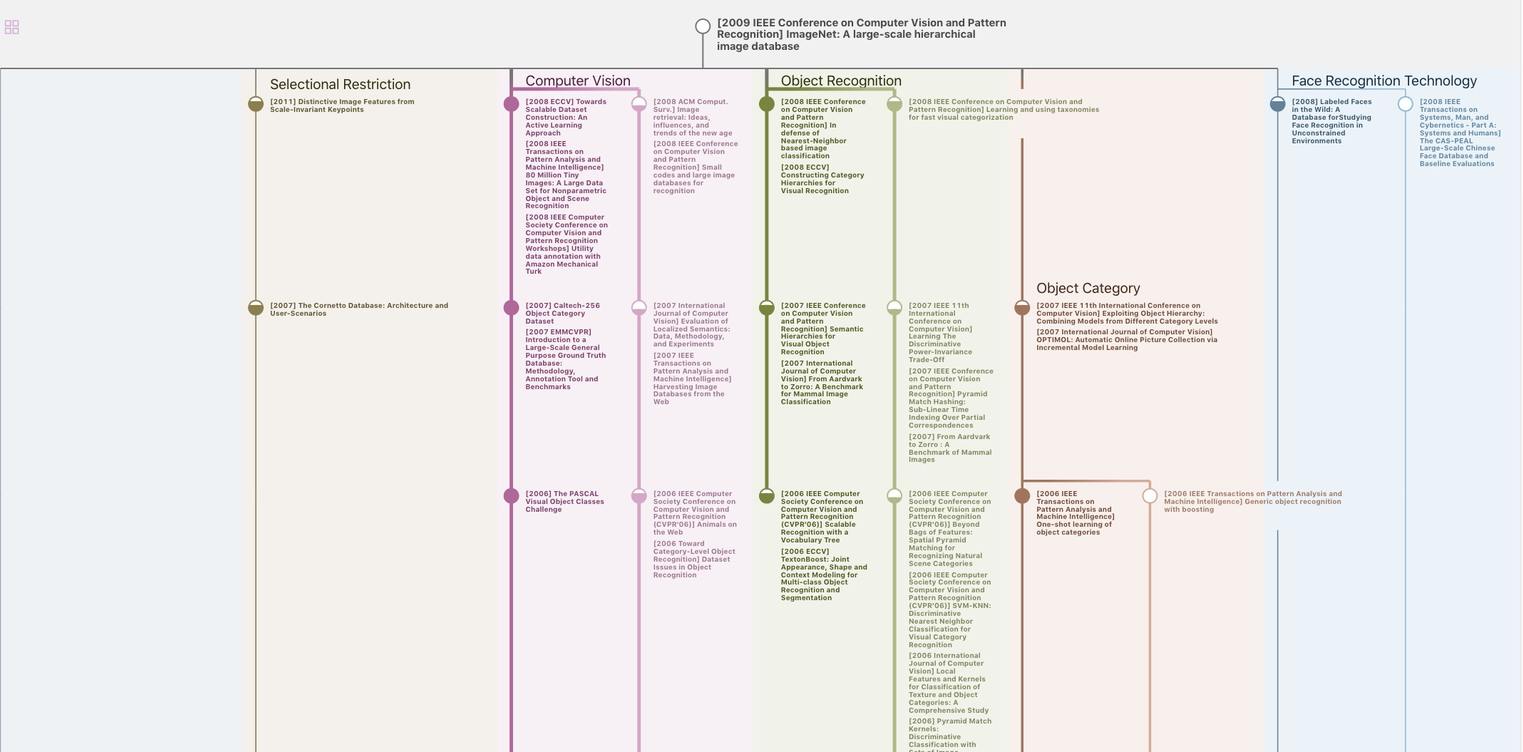
生成溯源树,研究论文发展脉络
Chat Paper
正在生成论文摘要