Multimodal deep learning water level forecasting model for multiscale drought alert in Feiyun River basin
EXPERT SYSTEMS WITH APPLICATIONS(2024)
摘要
Hydrological forecasting is an indispensable tool in intelligent water conservation for flood control and drought mitigation. Due to the influences of human activities and climate variability, accurate water level prediction poses a significant challenge. To address this, we develop a novel hybrid deep architecture, that is Dual-Stage Attention-based Multi-modal Deep Learning (DSAMDL), for reliable and interpretable multi-scale water level forecasting. Unlike previous techniques, multisource data is treated as different modalities in the proposed model. Firstly, we employ the one-dimensional Convolution to capture local trend features, followed by the Bidirectional Long Short-term Memory network to learn long-term dependencies. Subsequently, we design a dual-stage attention mechanism, which assigns contributions in a phased manner to different temporal and spatial. Finally, an adaptive fusion method is applied to enhance overall performance. To validate its accuracy and efficiency, short long-term drought water level forecasting and predictions under various events are implemented over six reservoir stations in the Feiyun River basin, Wenzhou City. Four evaluation metrics, namely RMSE, MAE, CORR, and NSE, are introduced for comprehensive assessment against the start-of-the art baselines. The results well-document that the DSAMDL framework achieves satisfactory accuracy, with an average improvement of 22.4%, 27.8%, 29.7%, and 11.5% in these four metrics, showcasing the model's effectiveness in handling complex multiscale drought water level forecasting.
更多查看译文
关键词
Water level forecast,Multiscale drought alert,Multimodal deep learning,Attention mechanism,Hydrological forecasting
AI 理解论文
溯源树
样例
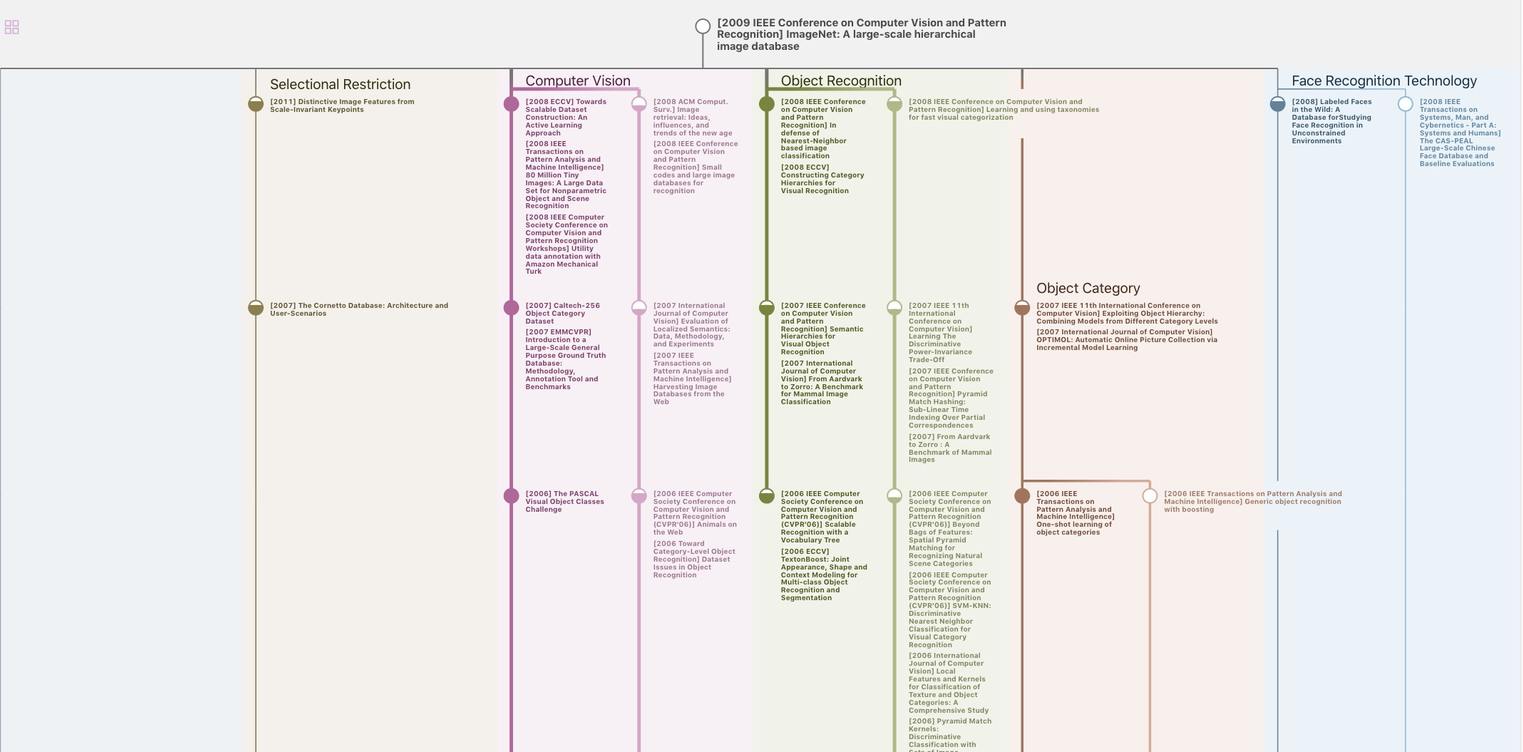
生成溯源树,研究论文发展脉络
Chat Paper
正在生成论文摘要