The q-gradient LMS spline adaptive filtering algorithm and its variable step-size variant
INFORMATION SCIENCES(2024)
摘要
In this study, an innovation q-gradient least-mean-square (LMS) spline adaptive filtering (S-AF) algorithm (SAF-qLMS) on the basis of the theory of q-derivative is proposed. The q-calculus confronts the issue of slow convergence by mitigating the over-reliance of LMS-type algorithms on the diffusion of eigenvalues in the input correlation matrix. Compared to conventional derivatives, the SAF-qLMS exploits q-calculus to compute the secant of the cost function, enabling it to take larger steps in the search direction for q > 1. Furthermore, for balancing the convergence rate and steady-state error of SAF-qLMS and solving the deficiency of the fixed step-size, the SAFVqLMS based on variable step-size is further proposed. Finally, the convergence conditions of the SAF-qLMS are discussed. Simulations in a correlated Gaussian input environment confirm the outstanding performance of the proposed algorithms for nonlinear system identification.
更多查看译文
关键词
Spline adaptive filter,Nonlinear signal,Variable step-size,Gaussian noise
AI 理解论文
溯源树
样例
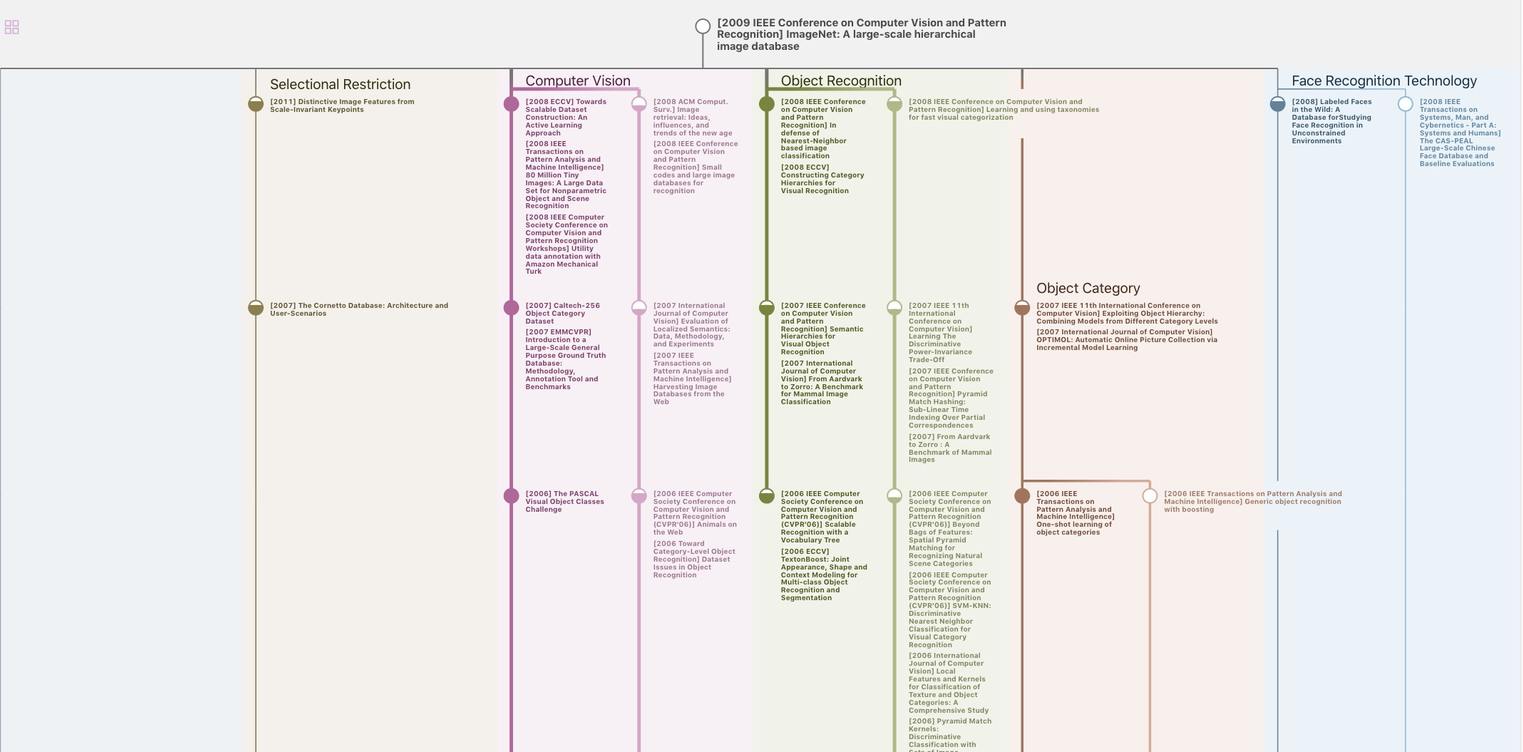
生成溯源树,研究论文发展脉络
Chat Paper
正在生成论文摘要