CSANet: Cross-self attention guided by semantic click embedding for interactive segmentation
ENGINEERING APPLICATIONS OF ARTIFICIAL INTELLIGENCE(2024)
摘要
In click-based deep interactive segmentation, click encoding and fusion with multi-scale features are vital for manipulating segmentation performance. Existing click encoding methods only incorporate position priors but lack semantics, leading to unstable interaction efficiency. Meanwhile, in order to fuse multi-scale features, current methods extract these features at the abstract semantic level but neglect the constraints imposed by detailed information on semantic features. This oversight makes the network prone to over-segmentation. To address these challenges, we propose a cross-self attention guided by semantic click embedding for interactive segmentation. First, we build semantic click embeddings from the semantic features by embedding positive clicks into continuous connected semantic regions while preserving the role of correction for negative clicks. This enriches the semantic priors for appropriate clicks. Next, we utilize the self-attention mechanism to leverage both detailed and semantic features of the network, constructing a cross-attention mechanism that suppresses the over-segmentation phenomenon. Finally, the semantic click embedding is utilized to weight the affinity matrix of the attention mechanism, ensuring that long-distance dependencies are only relevant to the target of interest. Comprehensive experiments prove that our approach improves interaction efficiency and achieves state-of-the-art performance on public datasets.
更多查看译文
关键词
Interactive segmentation,Click encoding,Semantic click embedding,Cross-self attention block,Affinity matrix
AI 理解论文
溯源树
样例
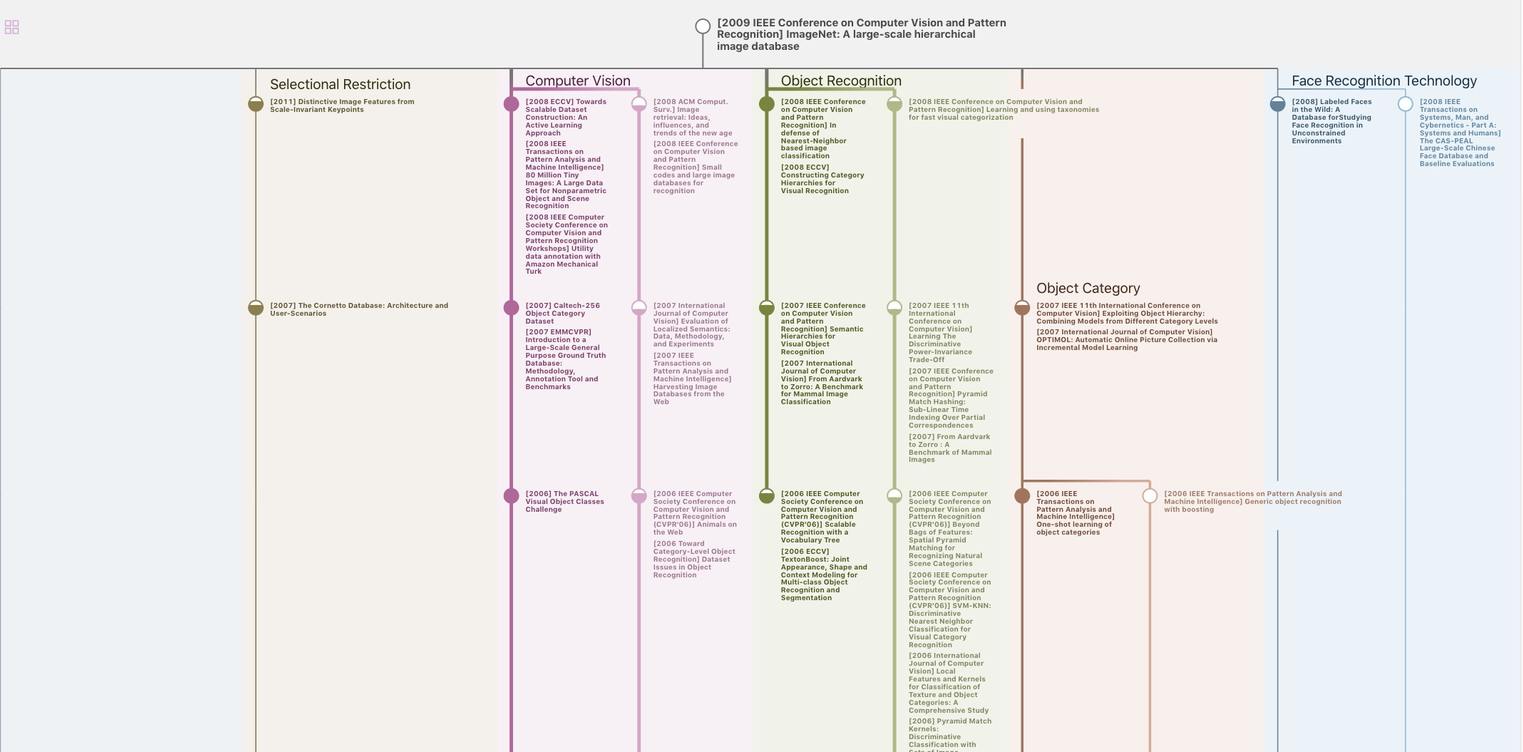
生成溯源树,研究论文发展脉络
Chat Paper
正在生成论文摘要