Blood clot and fibrin recognition method for serum images based on deep learning
CLINICA CHIMICA ACTA(2024)
摘要
Background: Detecting and identifying of clots and fibrins in serum is an important process in the analysis stage before laboratory analysis. Currently, visual examination is commonly employed in clinical laboratories for this purpose. However, this method is not only time-consuming but also highly subjective and may result in misjudgments.Method: A serum image blood clot and fibrin segmentation method based on improved UNeXt was proposed. The improved UNeXt segmentation network was used to train the self-built serum dataset, and the trained model was used for blood clot and fibrin segmentation in serum images. Whether the serum images contained blood clots and fibrins was identified according to the segmentation results.Results: The average Dice coefficient of the serum image segmentation network output was 0.8707, which realized more accurate segmentation of blood clots and fibrins in serum images. In 13,230 clinical serum samples, the sensitivity, specificity, and accuracy of blood clot and fibrins segmentation in serum images were 95.74%, 98.11% and 97.93%, respectively, which meet clinical test requirements.Conclusions: The improved UNeXt segmentation network rapidly and accurately segmented and recognized blood clots and fibrins in serum images, which provided an accurate basis for the sampling height of the sampling needle in the automated biochemical and immunological assembly line.
更多查看译文
关键词
UNeXt,Serum,Blood clots and fibrins,Segmentation and recognition
AI 理解论文
溯源树
样例
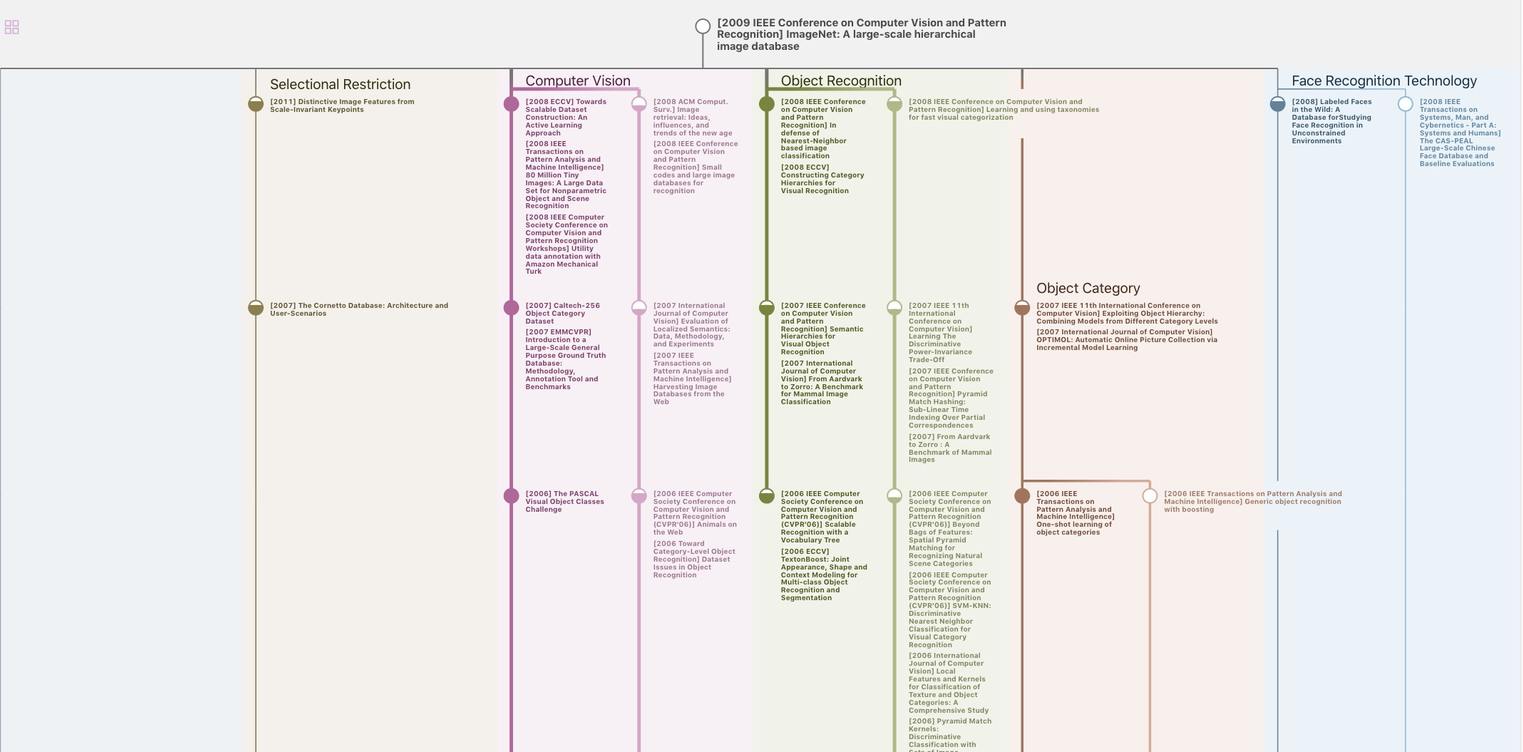
生成溯源树,研究论文发展脉络
Chat Paper
正在生成论文摘要