Prognostic pan-cancer and single-cancer models: A large-scale analysis using a real-world clinico-genomic database
medrxiv(2023)
摘要
Prognostic models in oncology have a profound impact on personalized cancer care and patient profiling, but tend to be heterogeneously developed and implemented in narrow patient cohorts. Here, we develop and benchmark multiple machine learning models to predict survival in pan-cancer and 16 single-cancer settings using a de-identified clinico-genomic database of 28,079 US patients with cancer. We identify key predictors of cancer prognosis, including 15 shared across seven or more cancer types, revealing strong consistency in cancer prognostic factors. We demonstrate that pan-cancer models generally outperform or match single-cancer models in predicting survival and risk stratifying patients, especially in smaller cancer cohorts, suggesting a unique transfer learning advantage of pan-cancer models. This work demonstrates the potential of pan-cancer approaches in enhancing the accuracy and applicability of prognostic models in oncology, paving the way for more personalized and effective cancer care strategies.
### Competing Interest Statement
R. Tibshirani and B. Narasimhan are paid consultants for Roche.
### Funding Statement
R. Tibshirani and B. Narasimhan received funding as paid consultants of Roche.
### Author Declarations
I confirm all relevant ethical guidelines have been followed, and any necessary IRB and/or ethics committee approvals have been obtained.
Yes
The details of the IRB/oversight body that provided approval or exemption for the research described are given below:
IRB of Copernicus Group gave ethical approval on 07Feb2018 for the electronic health records database used in this analysis.
I confirm that all necessary patient/participant consent has been obtained and the appropriate institutional forms have been archived, and that any patient/participant/sample identifiers included were not known to anyone (e.g., hospital staff, patients or participants themselves) outside the research group so cannot be used to identify individuals.
Yes
I understand that all clinical trials and any other prospective interventional studies must be registered with an ICMJE-approved registry, such as ClinicalTrials.gov. I confirm that any such study reported in the manuscript has been registered and the trial registration ID is provided (note: if posting a prospective study registered retrospectively, please provide a statement in the trial ID field explaining why the study was not registered in advance).
Yes
I have followed all appropriate research reporting guidelines, such as any relevant EQUATOR Network research reporting checklist(s) and other pertinent material, if applicable.
Yes
The data that support the findings of this study originated by Flatiron Health, Inc. and Foundation Medicine, Inc. Requests for data sharing by license or by permission for the specific purpose of replicating results in this manuscript can be submitted to PublicationsDataaccess{at}flatiron.com and cgdb-fmi{at}flatiron.com.
更多查看译文
AI 理解论文
溯源树
样例
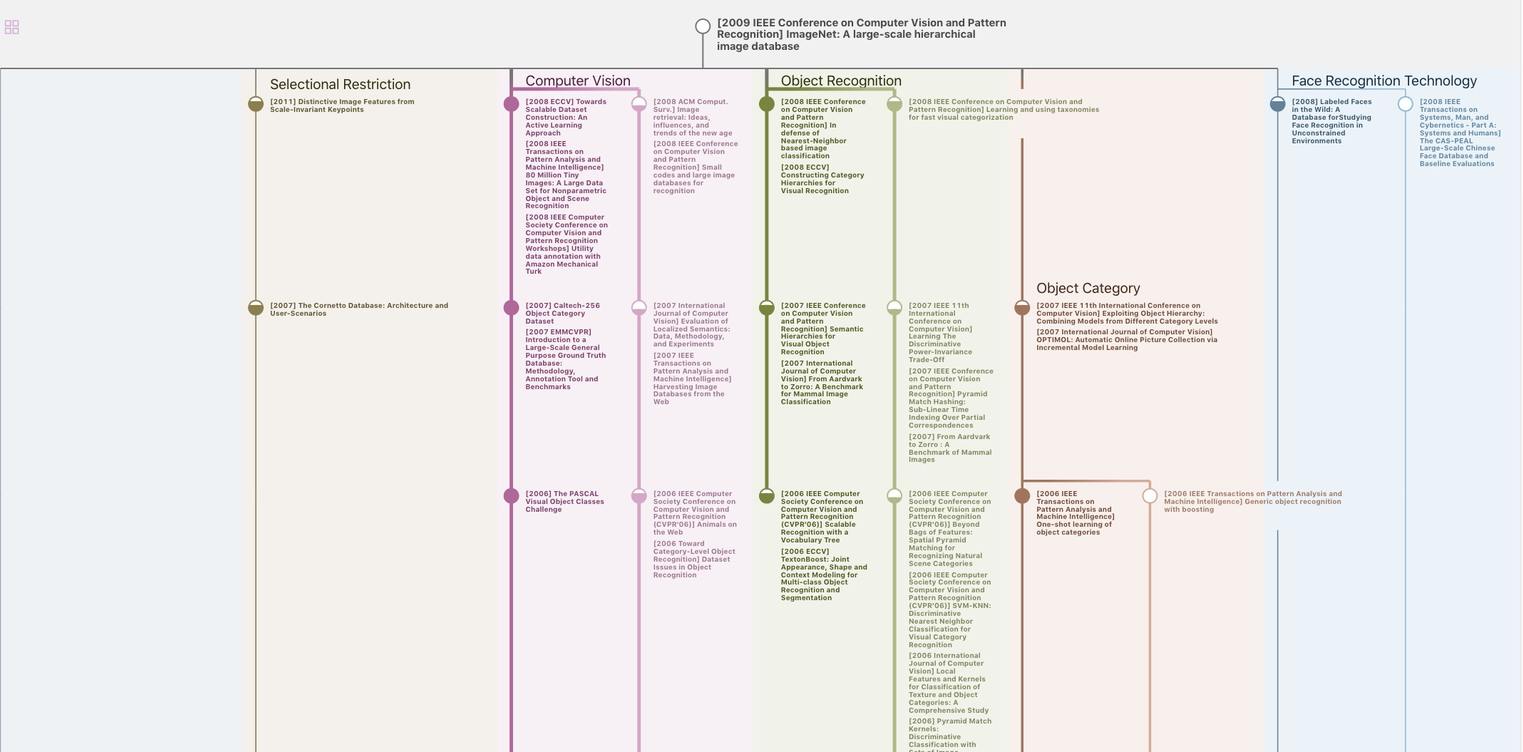
生成溯源树,研究论文发展脉络
Chat Paper
正在生成论文摘要