A Comparative Study of Machine Learning and Deep Learning Models for Microplastic Classification using FTIR Spectra
2023 18TH INTERNATIONAL JOINT SYMPOSIUM ON ARTIFICIAL INTELLIGENCE AND NATURAL LANGUAGE PROCESSING, ISAI-NLP(2023)
摘要
Microplastic contamination is a pressing environmental challenge, necessitating advanced detection and effective classification methods. As the prevalence of microplastics continues to rise globally, the need for innovative and accurate solutions becomes paramount. Aquatic ecosystems, in particular, are increasingly bearing the brunt of this contamination, emphasizing the urgency to address plastic pollution in our waters. Fourier-transform infrared (FTIR) spectroscopy, a widely recognized technique, offers promise but contends with spectral noise, particularly from membrane filters. In this context, our study contrasts machine learning (ML) and deep learning (DL) models for their ability to classify microplastics via FTIR spectra interfered by membrane filter noise. Utilizing an FTIR dataset from multiple sources, we rigorously assessed multiple ML algorithms and convolutional neural networks (CNNs). While ML models, specifically Support Vector Classification (SVC) and K-Nearest Neighbors (KNN), recorded accuracies of 95.99% and 95.55%, respectively, the DL model, LeNet5, surpassed them with 96.93%. Conversely, certain DL models underperformed, shedding light on the intricate nature of the identification task. Our findings provide valuable insights into the capabilities and constraints of ML and DL methodologies in the realm of microplastic classification through FTIR, encouraging further refinement and research in this critical environmental domain.
更多查看译文
关键词
convolutional neural networks (CNNs),deep learning (DL),Fourier-transform infrared (FTIR) spectroscopy,machine learning (ML),membrane filters,microplastic
AI 理解论文
溯源树
样例
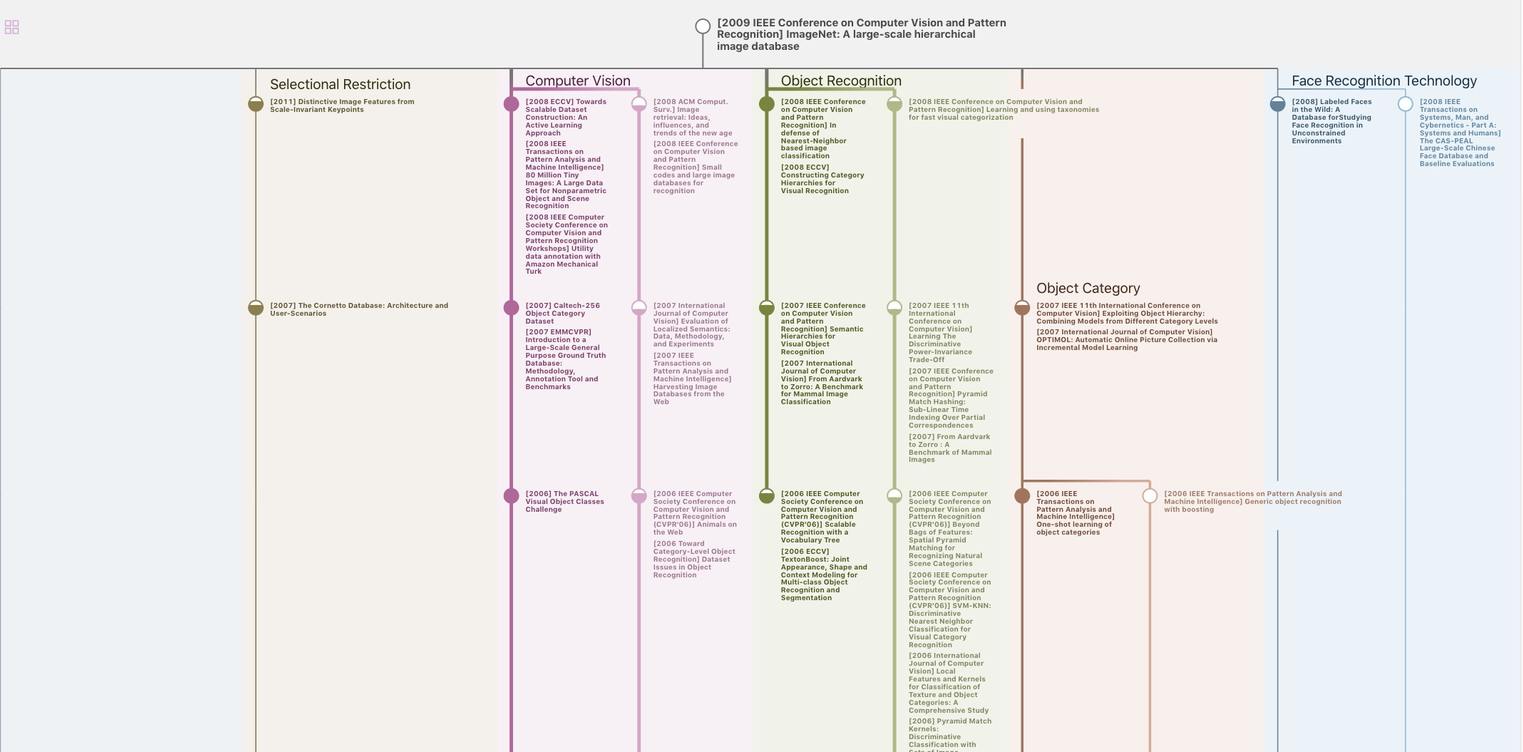
生成溯源树,研究论文发展脉络
Chat Paper
正在生成论文摘要