Variant Histogram Equalization based Enhancement to Transfer Learning in Detection of COVID-19 Chest X-Ray Images
2023 IEEE 2nd National Biomedical Engineering Conference (NBEC)(2023)
摘要
COVID-19 is a new pulmonary disease that has been straining the global healthcare system because of its high occurrence. It has been found that early-stage COVID-19 can be diagnosed using chest X-ay (CXR) images. Till now, most of the research has concentrated solely on the application of deep learning algorithms, which are valuable but lack proper pre-processing of CXR images. In this context, the purpose of this work is to study the cumulative effects of enhancement approaches on the performance of deep learning models. Within this research, four distinct iterations of histogram equalization image enhancement techniques were utilized on the chest X-ray (CXR) images. These encompass Median-Mean based Sub-Image Histogram Equalization (MMSICHE), Exposure based Sub-Image Histogram Equalization (ESIHE), Dominant Orientation based Texture Histogram Equalization (DOTHE), and Edge-based Texture Histogram Equalization (ETHE). The improved images are subsequently input into two pre-trained neural networks from the Visual Geometry Group (VGG) family, namely the VGG-16 and VGG-19 models, for the purposes of categorizing the CXR images into three categories: COVID-19, normal, and pneumonia. Ultimately, it was observed that the VGG-16 model employing the ESIHE image enhancement technique yielded the highest accuracy, reaching 92.17%.
更多查看译文
关键词
COVID-19,Chest X-Ray Images,Variant Histogram Equalization,Transfer Learning,VGG
AI 理解论文
溯源树
样例
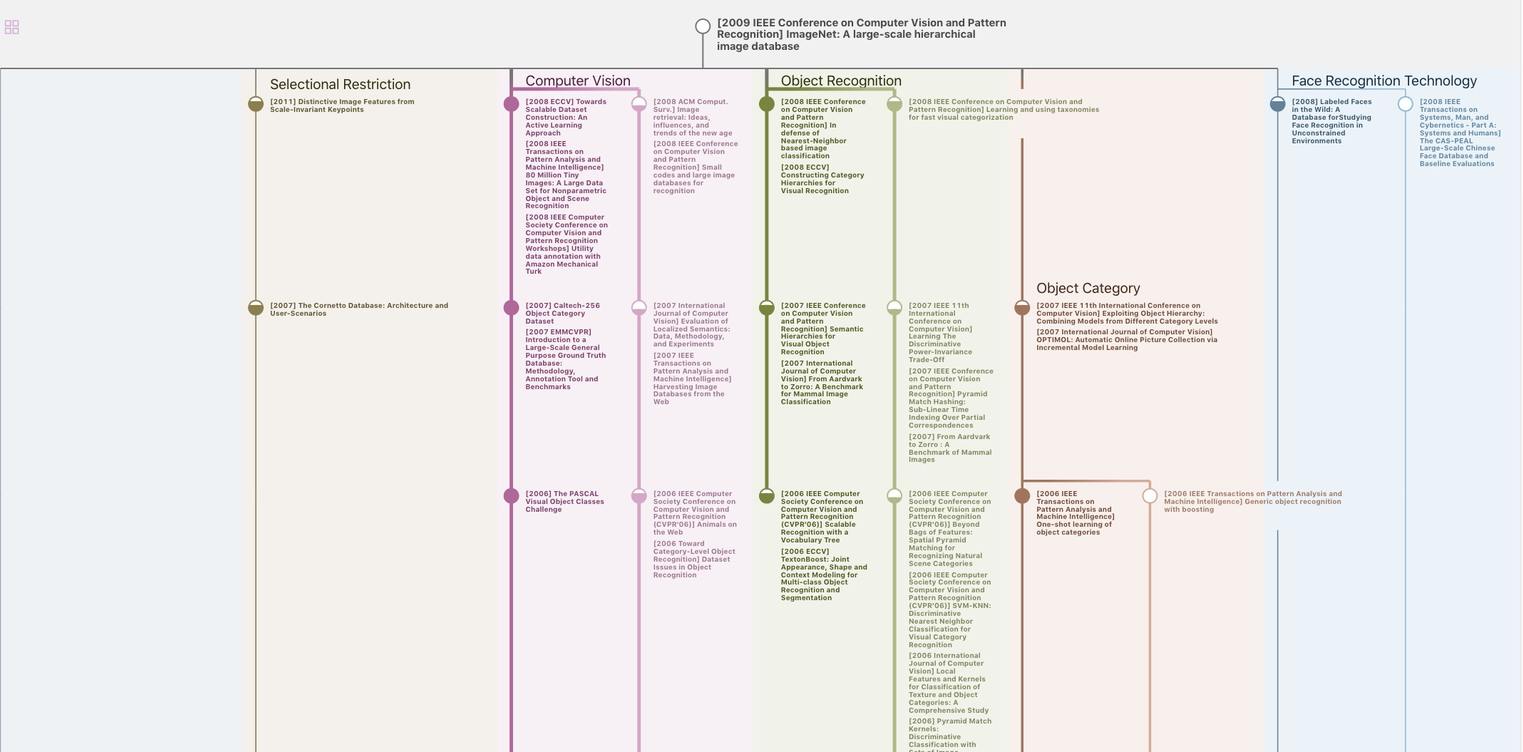
生成溯源树,研究论文发展脉络
Chat Paper
正在生成论文摘要