Neural Network Learning-Based Control for Nonlinear Systems With Time-Varying Powers
IEEE CONTROL SYSTEMS LETTERS(2023)
摘要
This letter focuses on the design of a state feedback controller for nonlinear systems with time-varying powers that utilize neural network (NN) to approximate unknown nonlinear functions. The dual homogeneity-based control is designed to recursively set up a controller with time-varying monotone degrees and a set of recursive Lyapunov functions. Neural networks approximate complex nonlinear functions by stacking multiple linear and nonlinear layers and adjusting their weights and biases through training. Approximation theory is developed to illustrate the relationship between the sophisticated architecture of neural networks and the intrinsic structure of unknown nonlinear functions that require approximation. Under the designed dual homogeneity-based control approach, the considered nonlinear system is globally uniformly asymptotically stable and the neural network error analysis is performed on unknown functions.
更多查看译文
关键词
Neural network approximations,smooth state feedback,time-varying powers,global stabilization
AI 理解论文
溯源树
样例
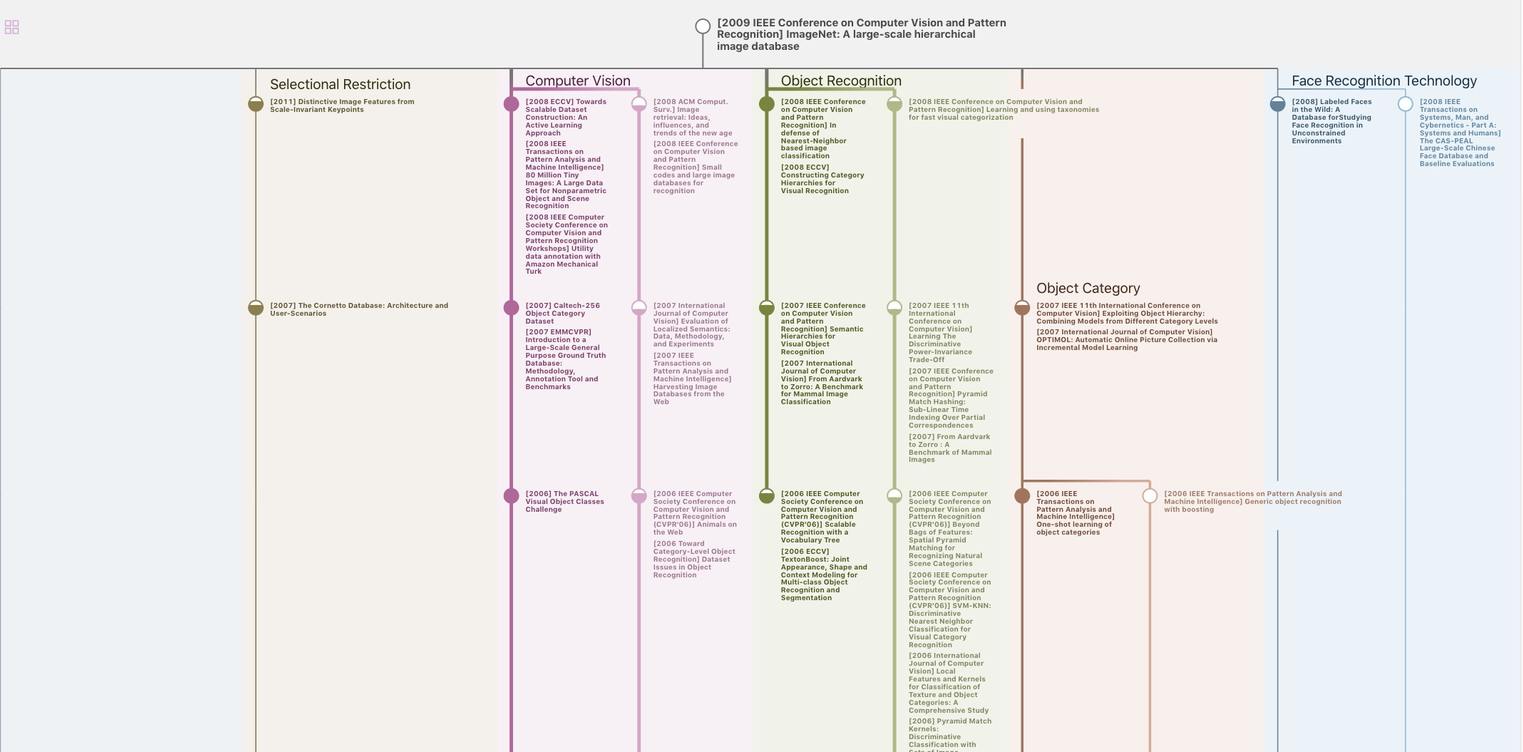
生成溯源树,研究论文发展脉络
Chat Paper
正在生成论文摘要