NS-IL: Neuro-Symbolic Visual Question Answering Using Incrementally Learnt, Independent Probabilistic Models for Small Sample Sizes
IEEE ACCESS(2023)
摘要
This paper is motivated by the challenge of providing accurate and contextually relevant answers to natural language questions about visual scenes, particularly in support of individuals with visual impairments. We present a system that is capable of incrementally learning both visual concepts and symbolic facts to answer natural language questions about visual scenes via rich concepts. Deep neural networks are used to learn a feature space from which visual classes are learned as independent probability distributions, allowing new classes to be added arbitrarily with small sample sizes and without the risk of catastrophic forgetting associated with traditional neural networks. Visual classes are not limited to object labels, but also include visual attributes. A knowledge graph is used to represent facts about objects, such as their actions, locations and the relationships between different objects. This allows facts to be stored explicitly and added incrementally. A large language model is used to translate between natural language questions and knowledge graph traversal queries, providing a natural visual question answering process.
更多查看译文
关键词
Neuro-symbolic system,visual question answering,classification system,Gaussian mixture model,incremental learning
AI 理解论文
溯源树
样例
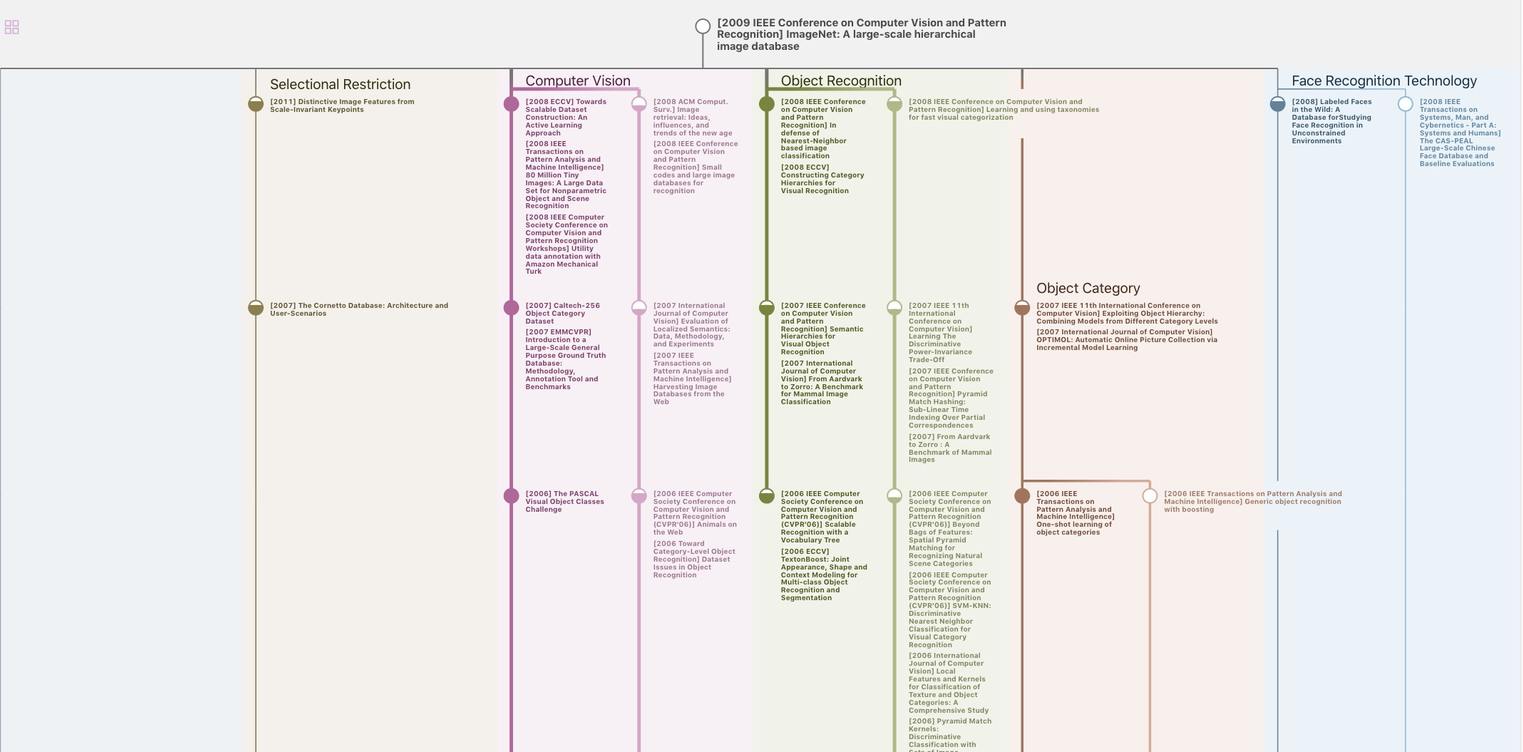
生成溯源树,研究论文发展脉络
Chat Paper
正在生成论文摘要