NDAMA: A Novel Deep Autoencoder and Multivariate Analysis Approach for IoT-Based Methane Gas Leakage Detection
IEEE ACCESS(2023)
摘要
Natural gas is widely used for domestic and industrial purposes, and whether it is being leaked into the air cannot be directly known. The current problem is that gas leakage is not only economically harmful but also detrimental to health. Therefore, much research has been done on gas damage and leakage risks, but research on predicting gas leakages is just beginning. In this study, we propose a method based on deep learning to predict gas leakage from environmental data. Our proposed method has successfully improved the performance of machine learning classification algorithms by efficiently preparing training data using a deep autoencoder model. The proposed method was evaluated on an open dataset containing natural gas and environmental information and compared with extreme gradient boost (XGBoost), K-nearest neighbors (KNN), decision tree (DT), random forest (RF), and naive Bayes (NB) algorithms. The proposed method is evaluated using accuracy, F1-score, mean square error (MSE), mean intersection over union (mIoU), and area under the ROC curve (AUC). The presented method in this study outperformed all compared methods. Moreover, the deep autoencoder and ordinal encoder-based XGBoost (DA-MA-XGBoost) showed the best performance by giving 99.51% accuracy, an F1-score of 99.53%, an MSE of 0.003, mIoU of 99.40 and an AUC of 99.62%.
更多查看译文
关键词
Leak detection,Machine learning algorithms,Data models,Predictive models,Pipelines,Labeling,Surveys,Encoding,Multivariate regression,Deep autoencoder,multivariate analysis,methane gas,risk detection,XGBoost
AI 理解论文
溯源树
样例
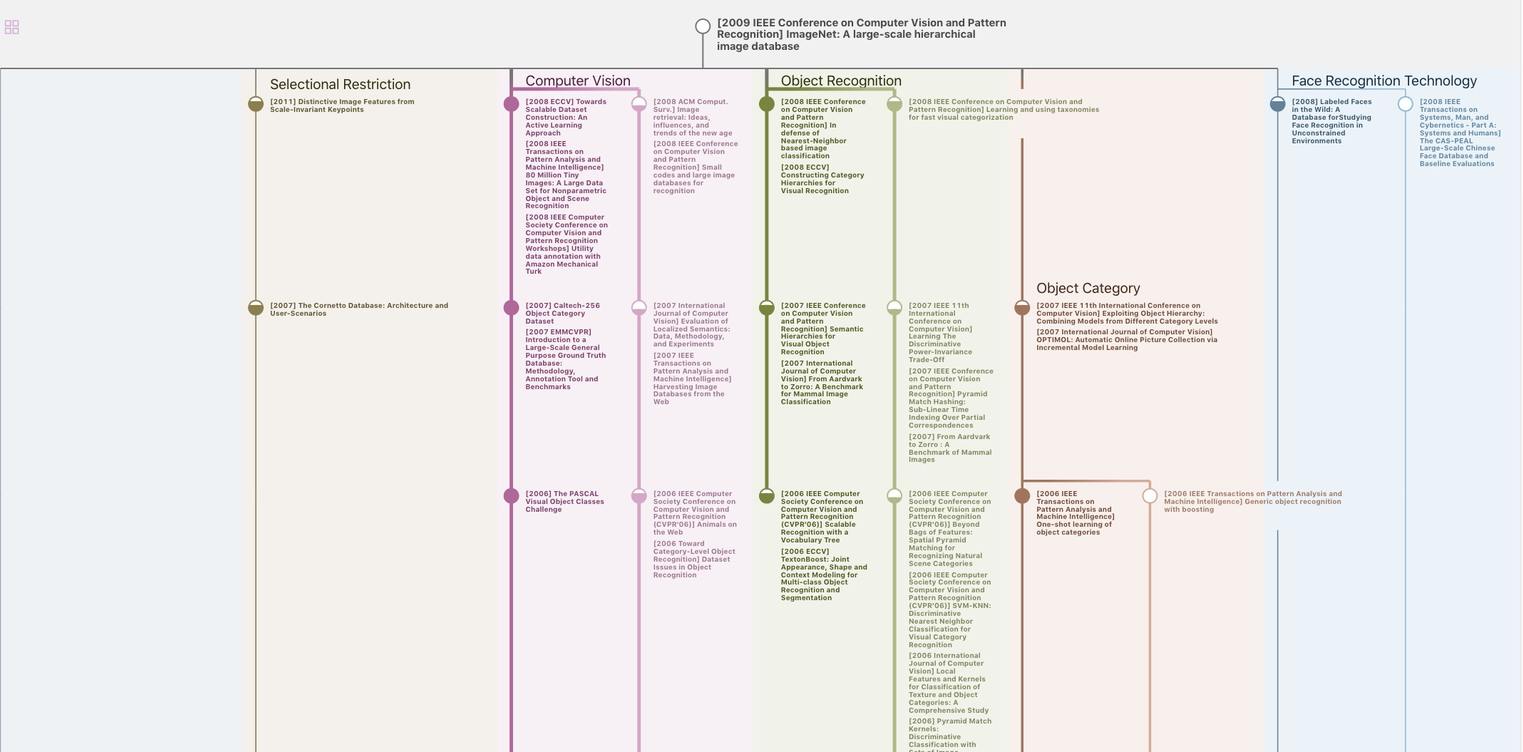
生成溯源树,研究论文发展脉络
Chat Paper
正在生成论文摘要