Multiscale Salient Alignment Learning for Remote-Sensing Image-Text Retrieval
IEEE TRANSACTIONS ON GEOSCIENCE AND REMOTE SENSING(2024)
摘要
Remote-sensing image-text (RSIT) retrieval involves the use of either textual descriptions or remote-sensing images (RSI) as queries to retrieve relevant RSIs or corresponding text descriptions. Many traditional cross-modal RSIT retrieval methods tend to overlook the importance of capturing salient information and establishing the prior similarity between RSIs and texts, leading to a decline in cross-modal retrieval performance. In this article, we address these challenges by introducing a novel approach known as multiscale salient image-guided text alignment (MSITA). This approach is designed to learn salient information by aligning text with images for effective cross-modal RSIT retrieval. The MSITA approach first incorporates a multiscale fusion module and a salient learning module to facilitate the extraction of salient information. In addition, it introduces an image-guided text alignment (IGTA) mechanism that uses image information to guide the alignment of texts, enabling the effective capture of fine-grained correspondences between RSI regions and textual descriptions. In addition to these components, a novel loss function is devised to enhance the similarity across different modalities and reinforce the prior similarity between RSIs and texts. Extensive experiments conducted on four widely adopted RSIT datasets affirm that the MSITA approach significantly enhances cross-modal RSIT retrieval performance in comparison to other state-of-the-art methods.
更多查看译文
关键词
Cross-modal retrieval,image-guided text alignment (IGTA),prior similarity,salient learning
AI 理解论文
溯源树
样例
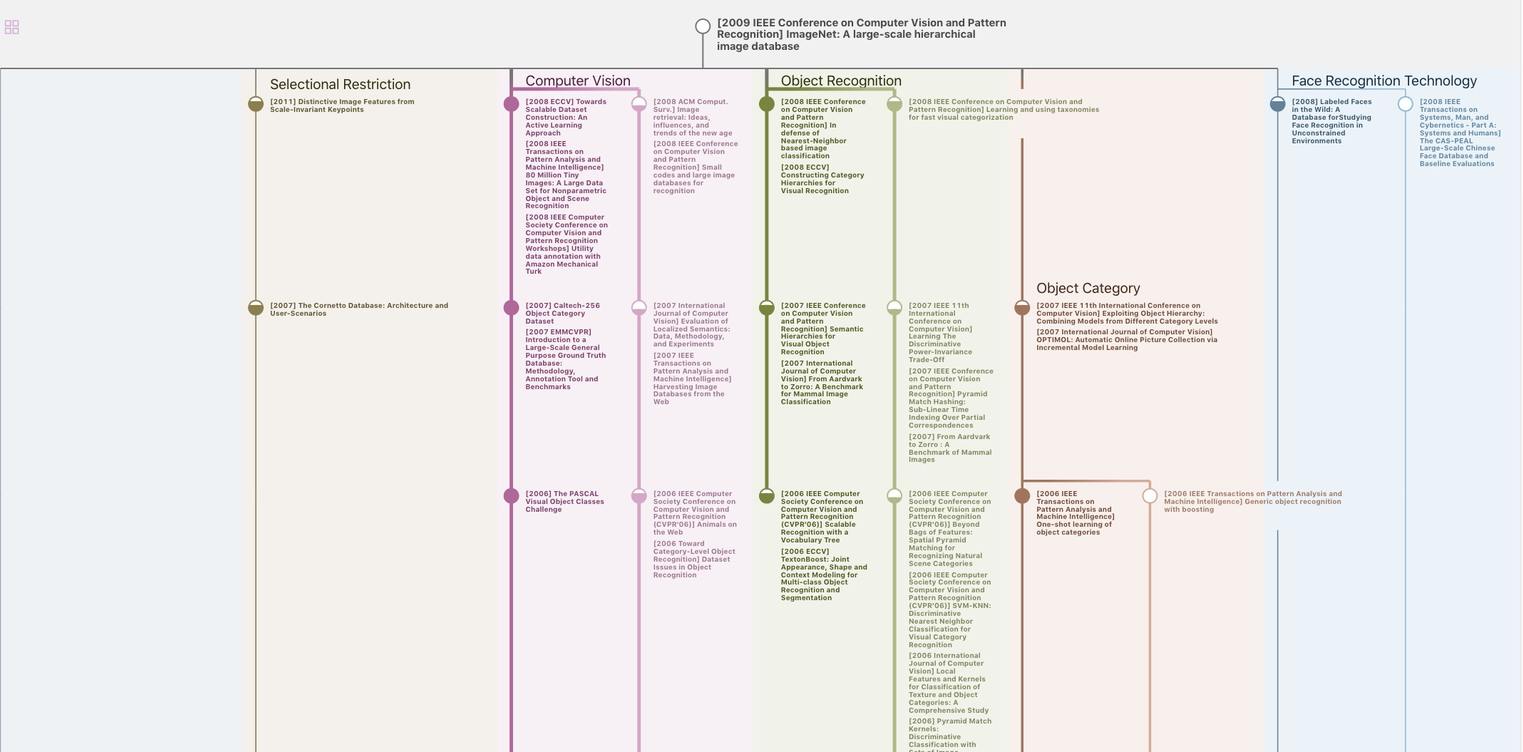
生成溯源树,研究论文发展脉络
Chat Paper
正在生成论文摘要