Dual-Channel Enhanced Decoder Network for Blind Hyperspectral Unmixing
IEEE GEOSCIENCE AND REMOTE SENSING LETTERS(2024)
摘要
Recently, deep learning has been widely applied in the field of blind hyperspectral unmixing (HU), which aims to simultaneously estimate constitutive endmembers and their abundances in hyperspectral images (HSIs). Generally, the HU process based on deep-learning methods consists of two parts: an encoder and a decoder. In many networks, the decoder stage uses the extracted semantic information of the HSI by the encoder, without direct access to the manifold structure of the HSI. To address this limitation and simultaneously capture both the semantic information and manifold structure of the HSI, in this letter, we propose a dual-channel enhanced decoder network (DED-Net) for the HU problem. Specifically, DED-Net redesigns a decoder by adding a dual-channel graph regularizer that establishes a physically meaningful immediate connection between the abundance and the HSI, effectively integrating both the information from the encoder and the original HSI to enhance endmembers and abundance estimation. Experimental results demonstrate the superiority of our proposed method, which leads to a more accurate unmixing performance.
更多查看译文
关键词
Decoding,Manifolds,Hyperspectral imaging,Transformers,Semantics,Feature extraction,Estimation,Deep learning,encoder and decoder,graph regularizer,hyperspectral unmixing (HU),manifold structure
AI 理解论文
溯源树
样例
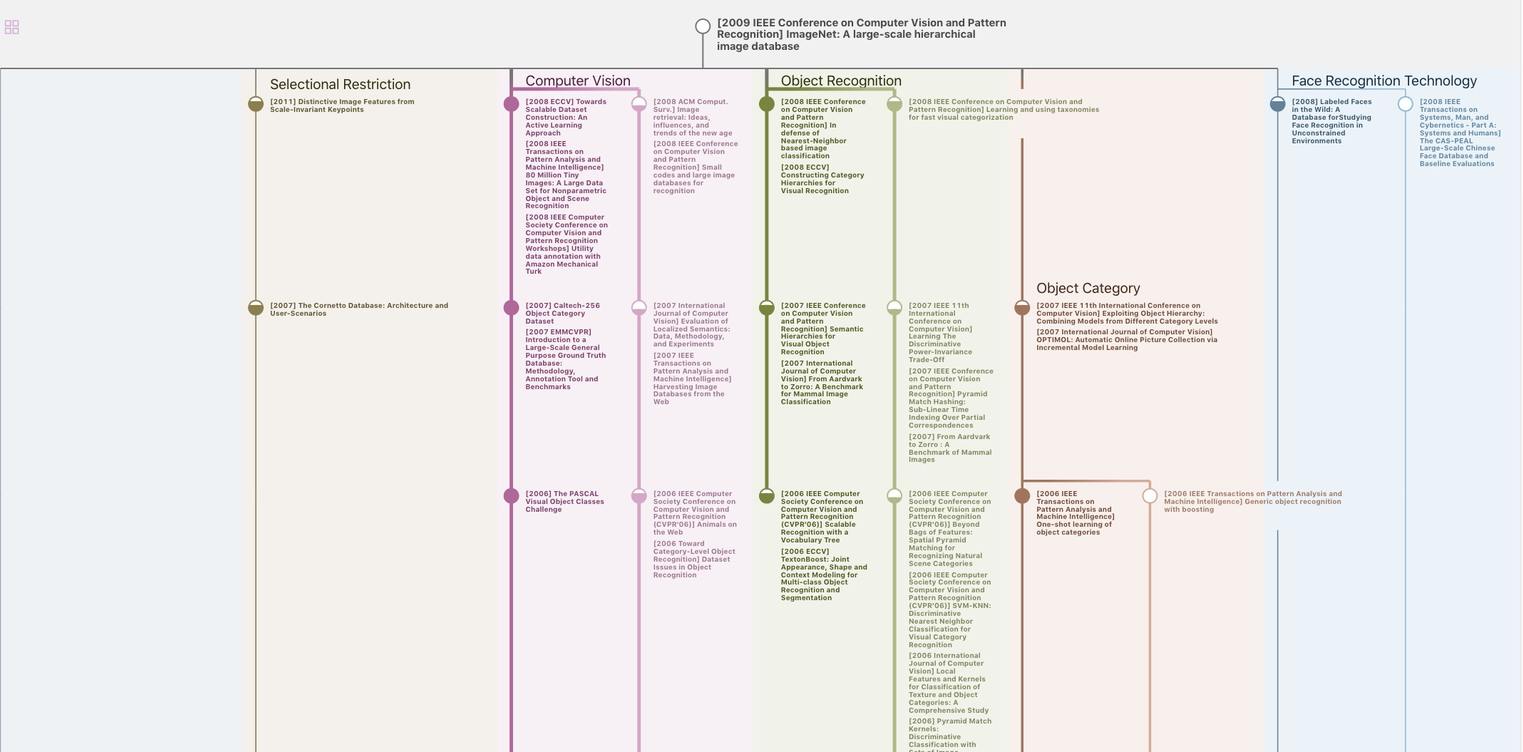
生成溯源树,研究论文发展脉络
Chat Paper
正在生成论文摘要