Toward Causality-Based Explanation of Aerial Scene Classifiers
IEEE GEOSCIENCE AND REMOTE SENSING LETTERS(2024)
摘要
Recently, convolutional neural networks (CNNs) have achieved great success by attaining state-of-the-art accuracies for aerial scene classification. However, there is a serious lack of good explanations for understanding the decision-making process of such black-box models. To establish the trustworthiness of the classifiers, various local and global explainers are commonly used nowadays. These primarily provide us with explanations in terms of the most influential features leading to the model decision. However, developing an explainer showing causal relationships among these features can offer more visibility, interpretability, and trustworthiness to the model users or stakeholders. To the best of our knowledge, this area is still underexplored in the context of scene-level classification of aerial images. We address this issue by proposing a novel causality-based CNN explainer based on gradient-weighted class activation mapping (Grad-CAM) and generative flow network (GFlowNet). Our proposed model is termed causal grad-CAM (CG-CAM), where Grad-CAM is used to highlight the relevant regions (layer-wise) in an aerial scene, and the GFlowNet is utilized to generate the directed acyclic graph (DAG) representing causal relationships between the feature maps at different layers of a deep CNN classifier, to achieve better understandability. Experimentation using the benchmark UCMerced and NWPU-RESISC45 datasets demonstrates the effectiveness of our CG-CAM-based explanations for aerial scene classification.
更多查看译文
关键词
Visualization,Scene classification,Predictive models,Decision making,Heating systems,Feature extraction,Training,Causality,convolutional neural network (CNN),explainer model,remote sensing,scene classification
AI 理解论文
溯源树
样例
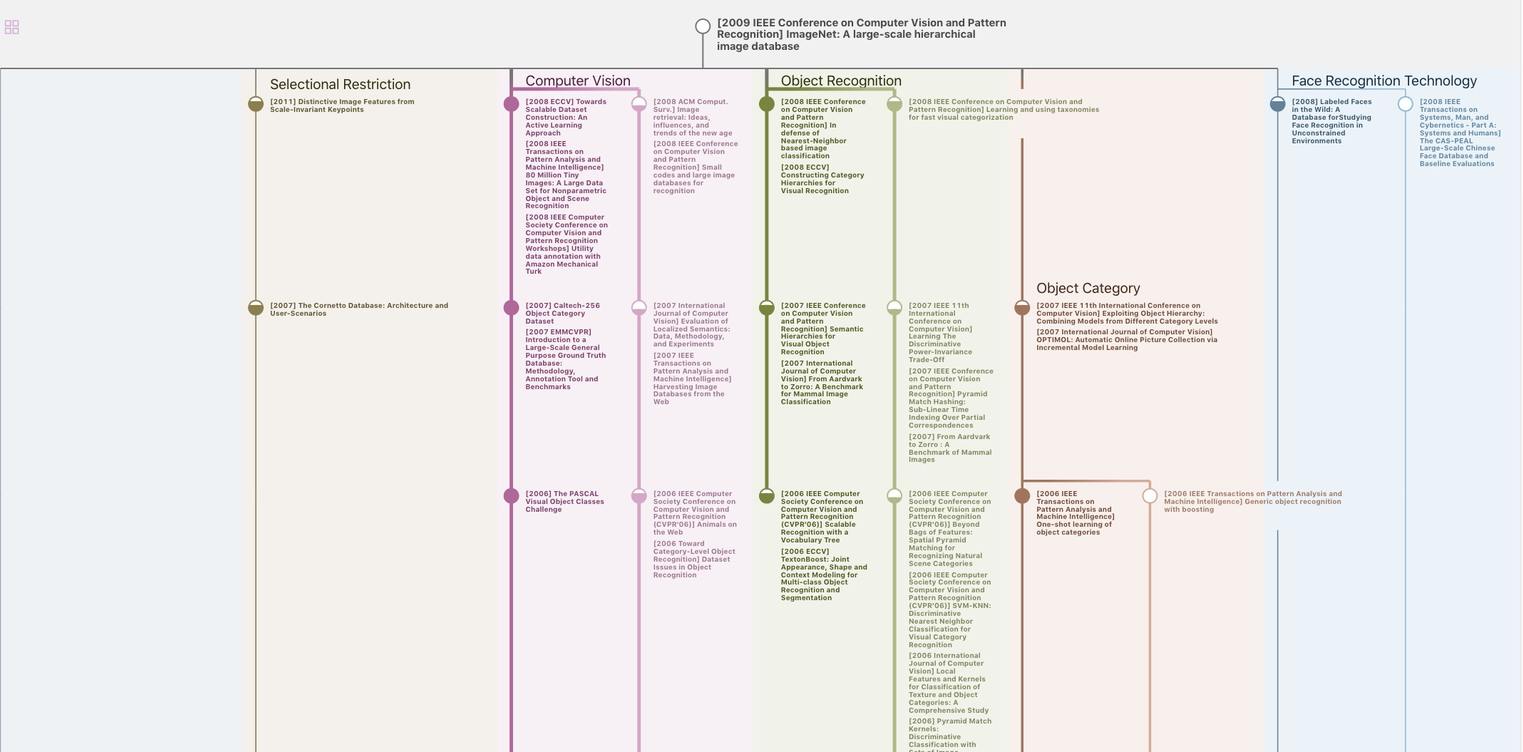
生成溯源树,研究论文发展脉络
Chat Paper
正在生成论文摘要