Clutter Suppression for Radar via Deep Joint Sparse Recovery Network
IEEE GEOSCIENCE AND REMOTE SENSING LETTERS(2024)
摘要
In radar detection, small and slow targets are easily overwhelmed by strong clutter. Traditional methods, such as singular value decomposition (SVD) and robust principal component analysis (RPCA), can suppress clutter and recover targets by using low-rank and sparse models. However, these methods rely on fixed prior information, which lacks adaptivity and suffers from unfavorable extensive manual hyperparameter tuning. To address these issues, a data-driven deep network model combined with an iterative algorithm called unfolding joint sparse recovery network (UFJSR-Net) is proposed to achieve the improved target detection performance. First, a joint sparse recovery (JSR) model is established and the fast iterative shrinkage/thresholding algorithm (FISTA) is derived to solve this model. Then, one iteration consisting of a linear operation and a nonlinear one can be recast into a single network layer and the stacking and combination of all layers will form the UFSJR-Net. Finally, the properties of the target and clutter can be learned by paired inputs and outputs training data to optimize the hyperparameters in the iterative algorithm to obtain the JSR model. Experiments on simulation data and measured radar data demonstrate that the proposed method exhibits advantages in detection performance over traditional decomposition methods under strong clutter with different intensities.
更多查看译文
关键词
Clutter,Radar,Doppler effect,Radar detection,Dictionaries,Radar cross-sections,Radar clutter,Clutter suppression,deep-unfolding network,joint sparse recovery (JSR),radar detection
AI 理解论文
溯源树
样例
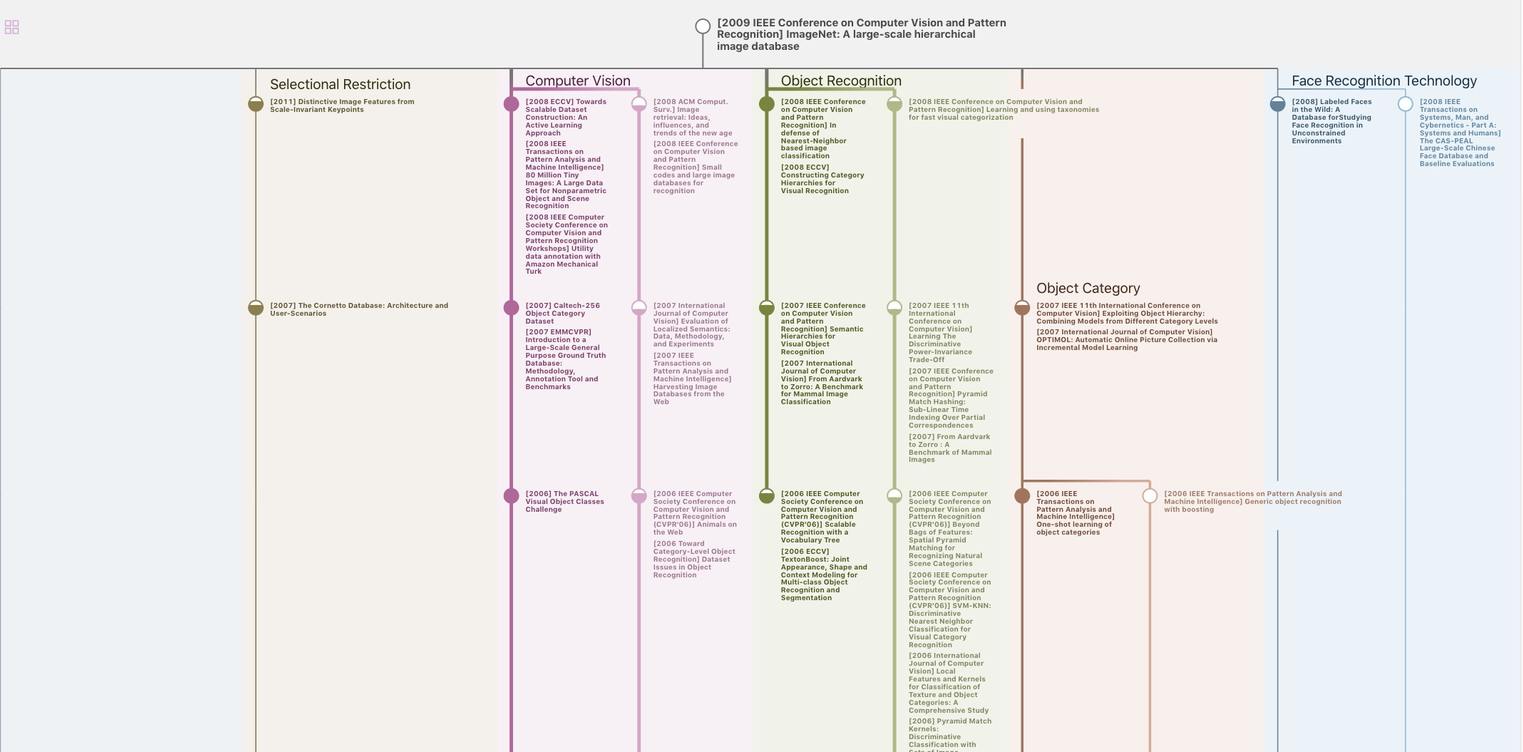
生成溯源树,研究论文发展脉络
Chat Paper
正在生成论文摘要