A Novel CNN-Based Radar Reflectivity Retrieval Network Using Geostationary Satellite Observations
IEEE GEOSCIENCE AND REMOTE SENSING LETTERS(2024)
摘要
A ground-based weather radar is commonly used for observing severe convective weather. However, the limited coverage of the radar poses difficulties in obtaining reliable radar observations for oceanic and mountainous regions. An effective solution is to derive radar data from meteorological satellite observations using deep-learning methods. This study proposes a novel feature redistribution module-based convolutional neural network (FR-CNN) to retrieve radar composite reflectivity (CREF) data from geostationary satellite observations. Differing from existing skip connection (SC)-based CNNs, FR-CNN adopts a feature redistribution module (FRM) to alleviate the problem of information scarcity during network propagation. In the FRM, a parallel attention block (PAB) is introduced to preserve key feature information and improve the retrieval ability of the FR-CNN. The evaluation results show that the FR-CNN can effectively reconstruct radar reflectivity data and has a better performance than other methods like U-Net in terms of assessment indices including the probability of detection (POD), false alarm ratio (FAR), and critical success index (CSI).
更多查看译文
关键词
Convolutional neural networks (CNNs),deep learning,geostationary satellite,weather radar
AI 理解论文
溯源树
样例
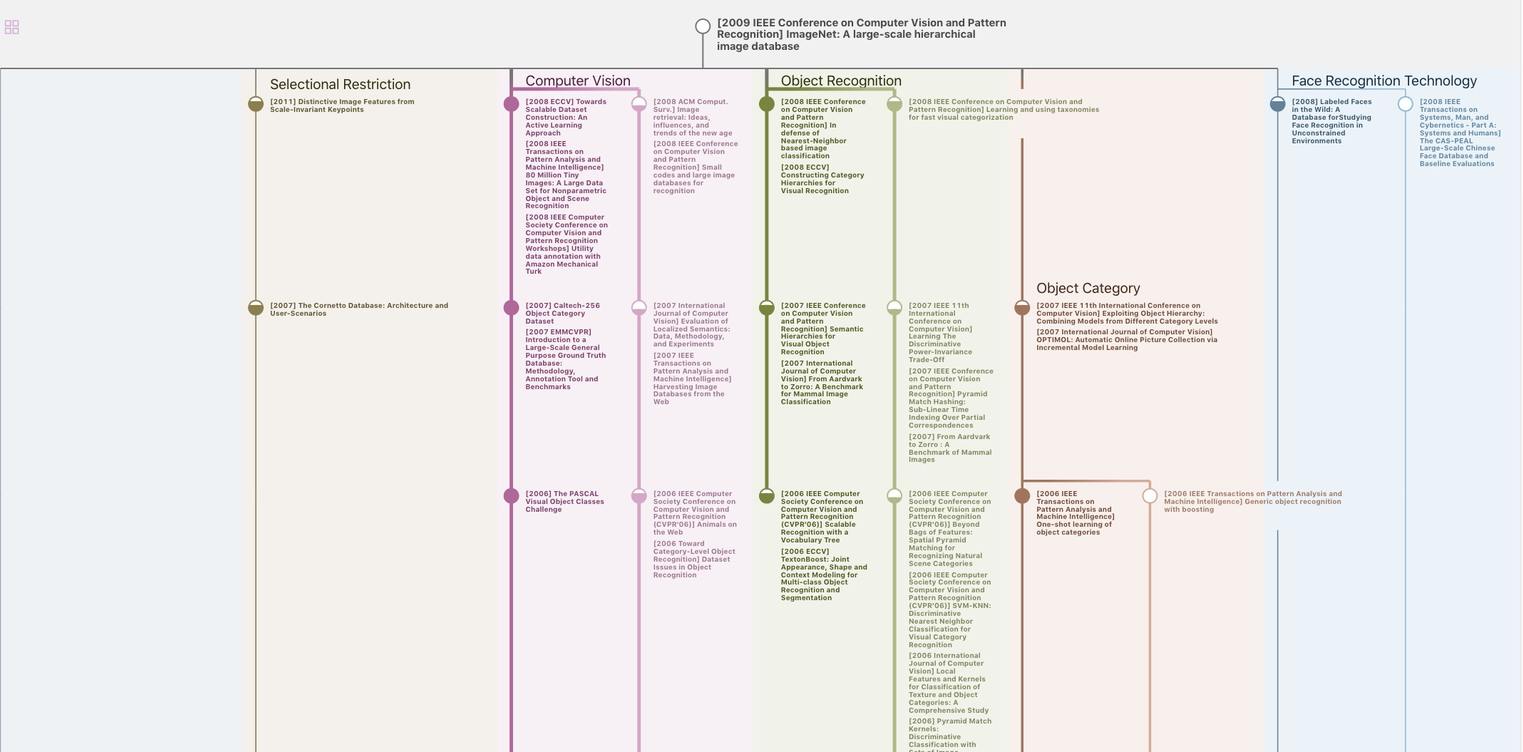
生成溯源树,研究论文发展脉络
Chat Paper
正在生成论文摘要