Explainability of the Effects of Non-perturbative Data Protection in Supervised Classification
2023 IEEE INTERNATIONAL CONFERENCE ON WEB INTELLIGENCE AND INTELLIGENT AGENT TECHNOLOGY, WI-IAT(2023)
摘要
The increasing availability of online data has meant that data-driven models have been applied to more and more tasks in recent years. In some domains and/or applications, such data must be protected before they are used. Hence, one of the problems only partially addressed in the literature is to determine how the performance of Machine Learning models is affected by data protection. More important, the explainability of the results of such models as a consequence of data protection has been even less investigated to date. In this paper, we refer to this very problem by considering non-perturbative data protection, and by studying the explainability of supervised models applied to the data classification task.
更多查看译文
关键词
Explainability,Data Protection,Machine Learning,Privacy,Classification
AI 理解论文
溯源树
样例
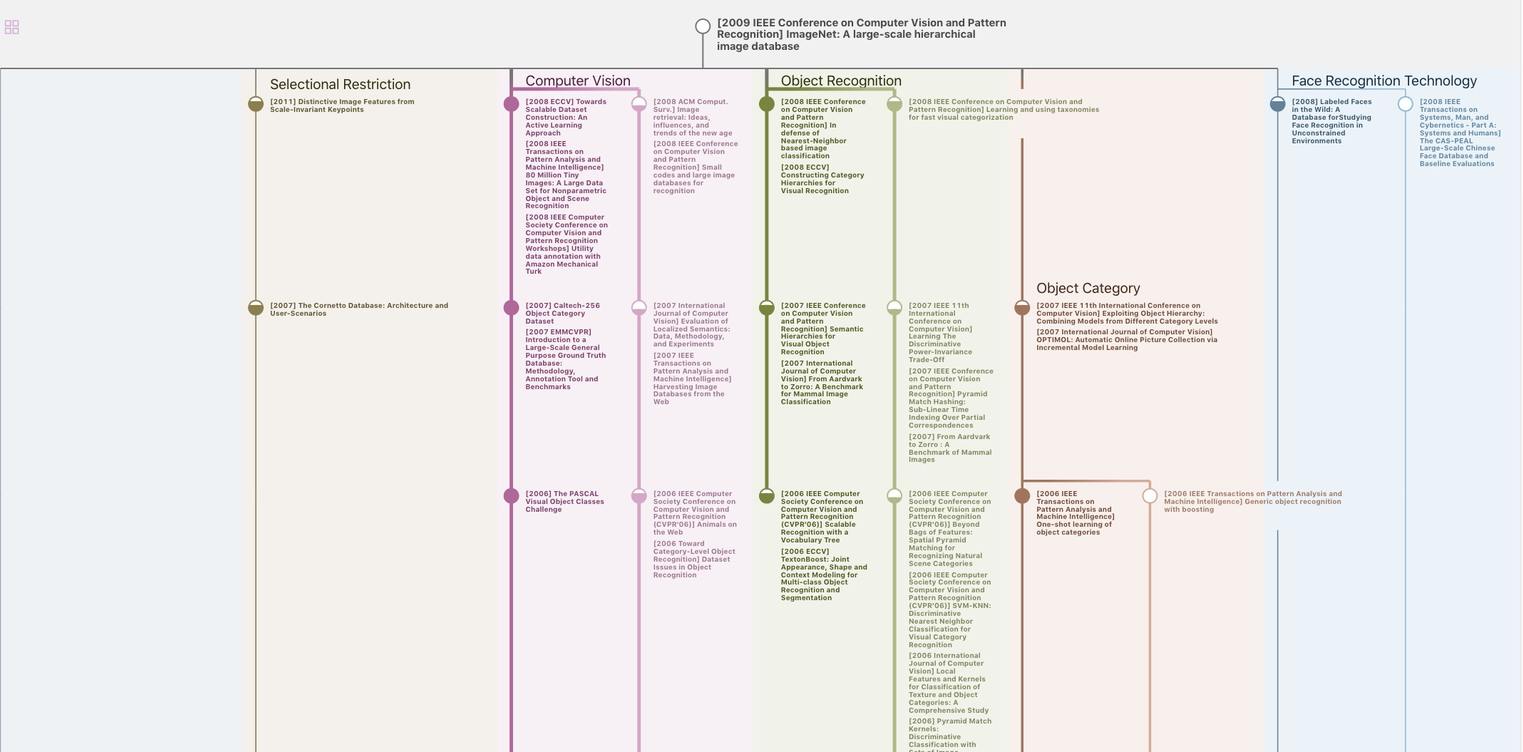
生成溯源树,研究论文发展脉络
Chat Paper
正在生成论文摘要