DAWSON: Data Augmentation using Weak Supervision On Natural Language
2023 IEEE INTERNATIONAL CONFERENCE ON WEB INTELLIGENCE AND INTELLIGENT AGENT TECHNOLOGY, WI-IAT(2023)
摘要
We propose a novel data augmentation model for text using all available data through weak supervision. To improve generalization, recent work in the field uses BERT and masked language modeling to conditionally augment data. These models involve a small, high-quality labeled dataset, but omit the abundance of unlabeled data which is likely to be present if one considers a model in the first place. Weak supervision methods make use of the vastness of unlabeled data, but largely omit the available ground truth labels. We combine data augmentation and weak supervision techniques into a holistic method, consisting of 4 training phases and 2 inference phases, to efficiently train an end-to-end model when only a small amount of annotated data is available. We outperform a conditional augmentation benchmark for the SST-2 task by 1.5, QQP task by 4.4, and QNLI task by 3.0 absolute accuracy percentage points, and show that data augmentation is also effective for natural language understanding tasks, such as QQP and QNLI.
更多查看译文
关键词
data augmentation,weakly supervised learning,weak supervision,BERT,natural language processing
AI 理解论文
溯源树
样例
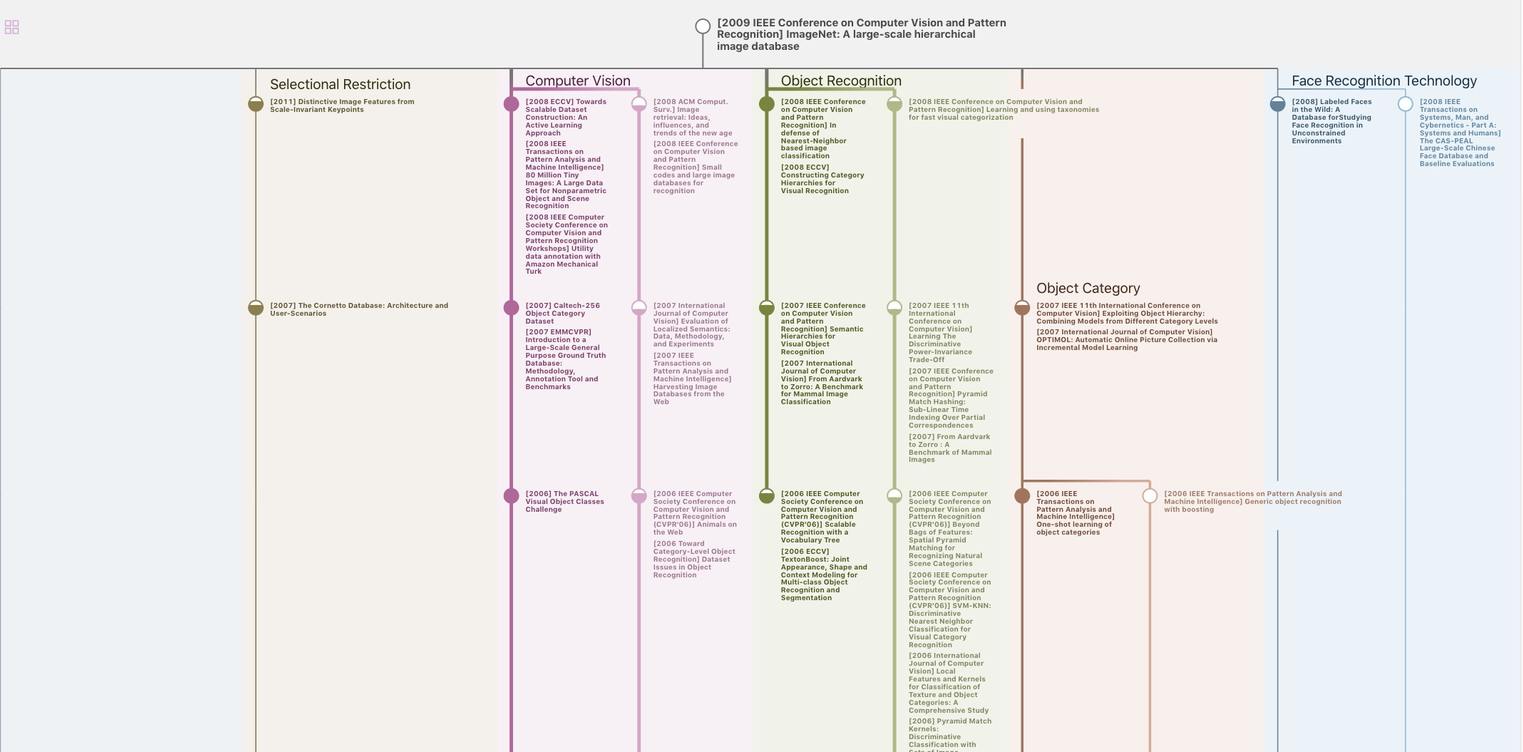
生成溯源树,研究论文发展脉络
Chat Paper
正在生成论文摘要