Application of automated machine learning for histological evaluation of feline endoscopic samples
JOURNAL OF VETERINARY MEDICAL SCIENCE(2024)
摘要
. Differentiating intestinal T-cell lymphoma from chronic enteropathy (CE) in endoscopic samples is often challenging. In the present study, automated machine learning systems were developed to distinguish between the two diseases, predict clonality, and detect prognostic factors of intestinal lymphoma in cats. Four models were created for four experimental conditions: experiment 1 to distinguish between intestinal T-cell lymphoma and CE; experiment 2 to distinguish large cell lymphoma, small cell lymphoma, and CE; experiment 3 to distinguish granzyme B+ lymphoma, granzyme B- lymphoma, and CE; and experiment 4 to distinguish between T-cell receptor (TCR) clonal population and TCR polyclonal population. After each experiment, a pathologist reviewed the test images and scored for lymphocytic infiltration, epitheliotropism, and epithelial injury. The models of experiments 1-4 achieved area under the receiver operating characteristic curve scores of 0.943 (precision, 87.59%; recall, 87.59%), 0.962 (precision, 86.30%; recall, 86.30%), 0.904 (precision, 82.86%; recall, 80%), and 0.904 (precision, 81.25%; recall, 81.25%), respectively. The images predicted as intestinal T-cell lymphoma showed significant infiltration of lymphocytes and epitheliotropism than CE. These models can provide evaluation tools to assist pathologists with differentiating between intestinal T-cell lymphoma and CE.
更多查看译文
关键词
cat,intestinal T-cell lymphoma,machine learning
AI 理解论文
溯源树
样例
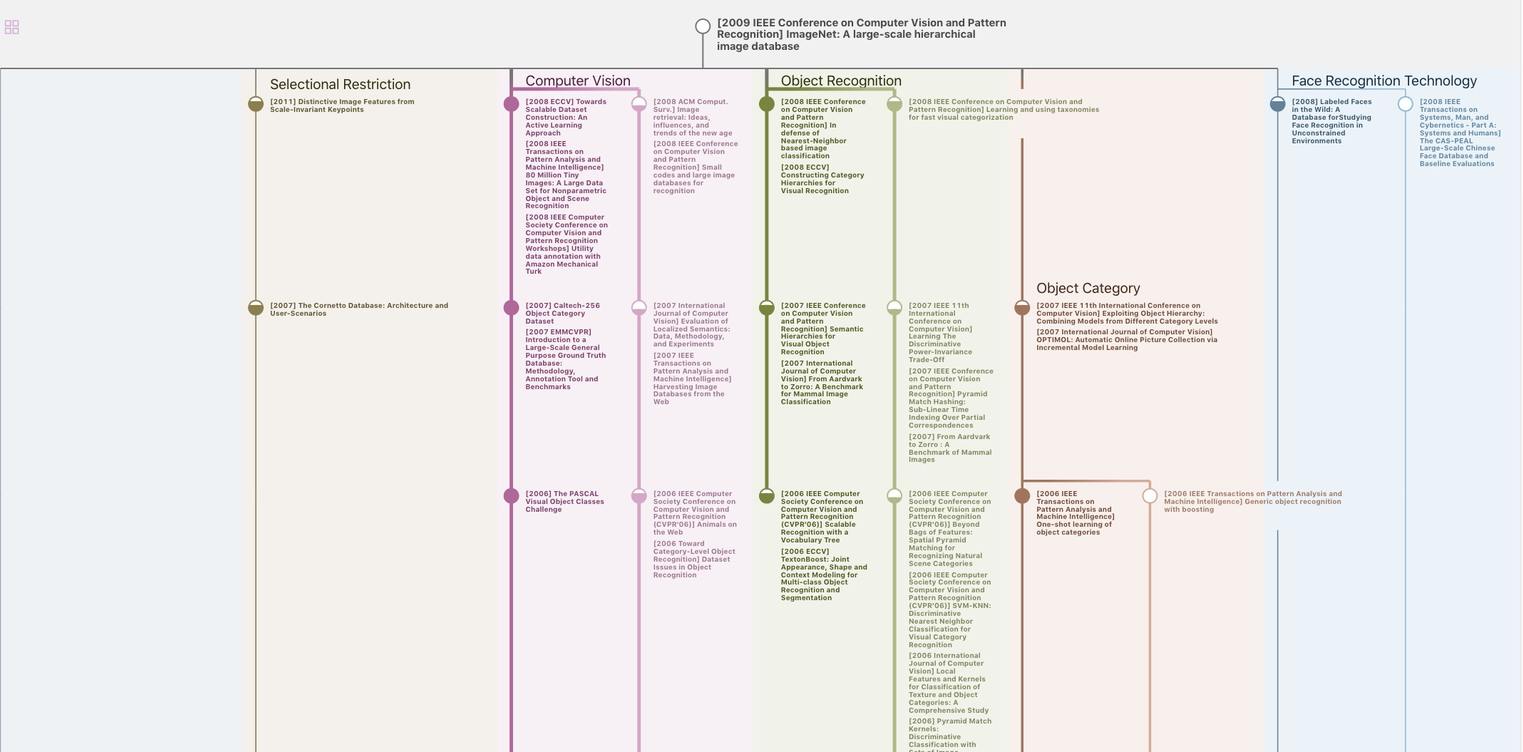
生成溯源树,研究论文发展脉络
Chat Paper
正在生成论文摘要