Longitudinal resting-state network connectivity changes in electroconvulsive therapy patients compared to healthy controls
BRAIN STIMULATION(2024)
摘要
Objective: Electroconvulsive therapy (ECT) is effective for major depressive episodes. Understanding of underlying mechanisms has been increased by examining changes of brain connectivity but studies often do not correct for test-retest variability in healthy controls (HC). In this study, we investigated changes in resting-state networks after ECT in a multicenter study. Methods: Functional resting-state magnetic resonance imaging data, acquired before start and within one week after ECT, from 90 depressed patients were analyzed, as well as longitudinal data of 24 HC. Group-information guided independent component analysis (GIG-ICA) was used to spatially restrict decomposition to twelve canonical resting-state networks. Selected networks of interest were the default mode network (DMN), salience network (SN), and left and right frontoparietal network (LFPN, and RFPN). Whole-brain voxel-wise analyses were used to assess group differences at baseline, group by time interactions, and correlations with treatment effectiveness. In addition, between-network connectivity and within-network strengths were computed. Results: Within-network strength of the DMN was lower at baseline in ECT patients which increased after ECT compared to HC, after which no differences were detected. At baseline, ECT patients showed lower whole-brain voxel-wise DMN connectivity in the precuneus. Increase of within-network strength of the LFPN was correlated with treatment effectiveness. We did not find whole-brain voxel-wise or between-network changes. Conclusion: DMN within-network connectivity normalized after ECT. Within-network increase of the LFPN in ECT patients was correlated with higher treatment effectiveness. In contrast to earlier studies, we found no wholebrain voxel-wise changes, which highlights the necessity to account for test-retest effects.
更多查看译文
关键词
Major depressive disorder,BOLD imaging,Functional networks
AI 理解论文
溯源树
样例
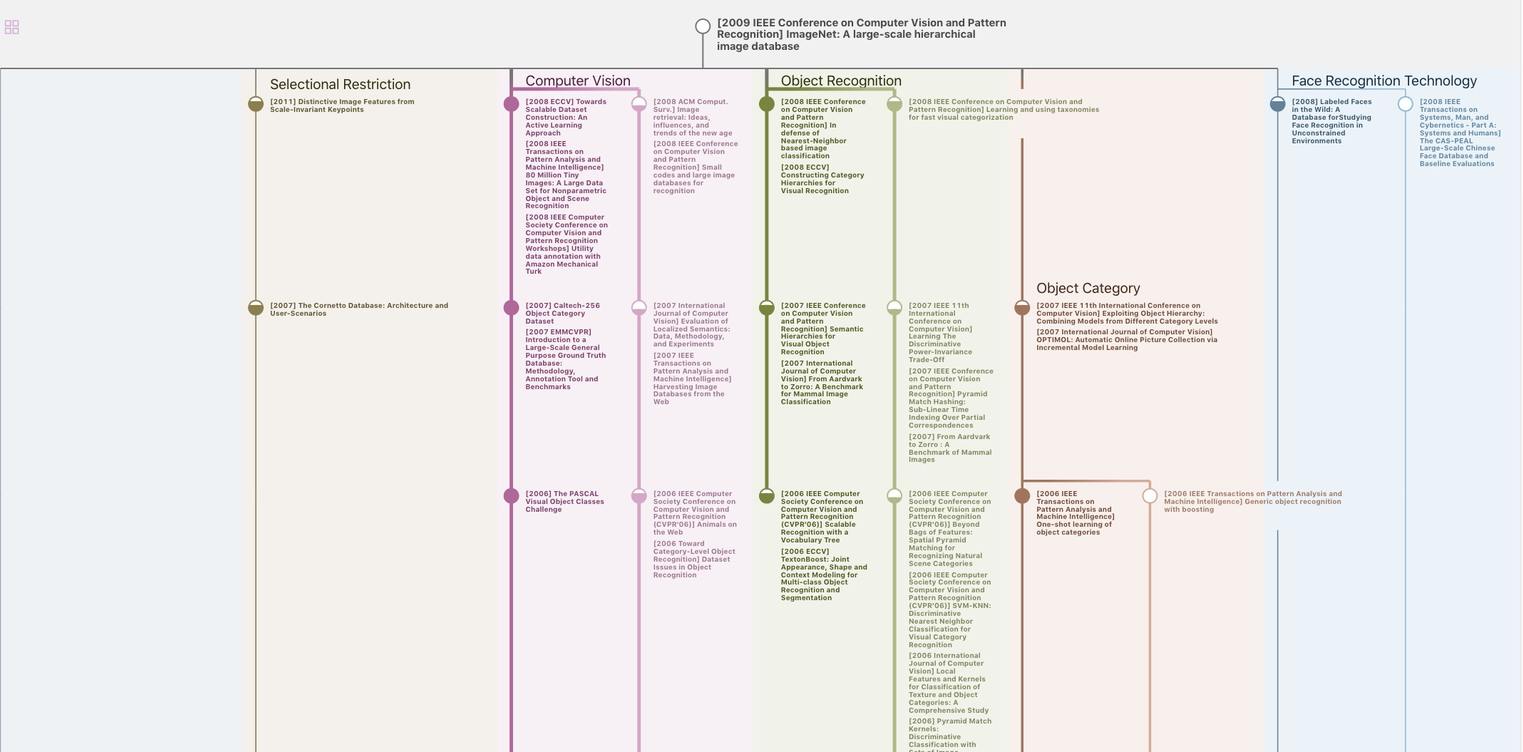
生成溯源树,研究论文发展脉络
Chat Paper
正在生成论文摘要