Guiding Principles to Address the Impact of Algorithm Bias on Racial and Ethnic Disparities in Health and Health Care
JAMA NETWORK OPEN(2023)
摘要
Importance Health care algorithms are used for diagnosis, treatment, prognosis, risk stratification, and allocation of resources. Bias in the development and use of algorithms can lead to worse outcomes for racial and ethnic minoritized groups and other historically marginalized populations such as individuals with lower income. Objective To provide a conceptual framework and guiding principles for mitigating and preventing bias in health care algorithms to promote health and health care equity. Evidence Review The Agency for Healthcare Research and Quality and the National Institute for Minority Health and Health Disparities convened a diverse panel of experts to review evidence, hear from stakeholders, and receive community feedback. Findings The panel developed a conceptual framework to apply guiding principles across an algorithm's life cycle, centering health and health care equity for patients and communities as the goal, within the wider context of structural racism and discrimination. Multiple stakeholders can mitigate and prevent bias at each phase of the algorithm life cycle, including problem formulation (phase 1); data selection, assessment, and management (phase 2); algorithm development, training, and validation (phase 3); deployment and integration of algorithms in intended settings (phase 4); and algorithm monitoring, maintenance, updating, or deimplementation (phase 5). Five principles should guide these efforts: (1) promote health and health care equity during all phases of the health care algorithm life cycle; (2) ensure health care algorithms and their use are transparent and explainable; (3) authentically engage patients and communities during all phases of the health care algorithm life cycle and earn trustworthiness; (4) explicitly identify health care algorithmic fairness issues and trade-offs; and (5) establish accountability for equity and fairness in outcomes from health care algorithms. Conclusions and Relevance Multiple stakeholders must partner to create systems, processes, regulations, incentives, standards, and policies to mitigate and prevent algorithmic bias. Reforms should implement guiding principles that support promotion of health and health care equity in all phases of the algorithm life cycle as well as transparency and explainability, authentic community engagement and ethical partnerships, explicit identification of fairness issues and trade-offs, and accountability for equity and fairness.
更多查看译文
AI 理解论文
溯源树
样例
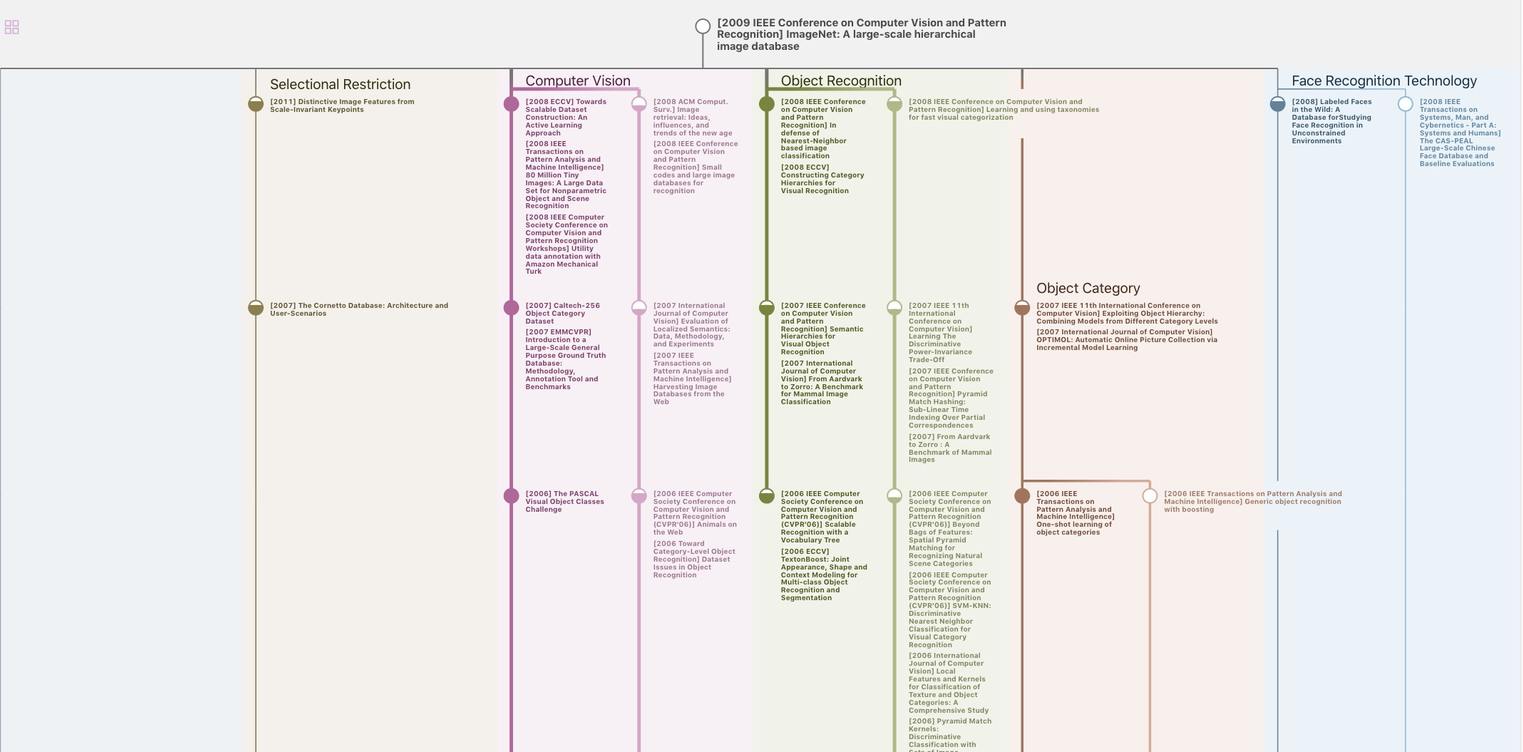
生成溯源树,研究论文发展脉络
Chat Paper
正在生成论文摘要