Dynamic Subcluster-Aware Network for Few-Shot Skin Disease Classification
IEEE TRANSACTIONS ON NEURAL NETWORKS AND LEARNING SYSTEMS(2023)
摘要
This article addresses the problem of few-shot skin disease classification by introducing a novel approach called the subcluster-aware network (SCAN) that enhances accuracy in diagnosing rare skin diseases. The key insight motivating the design of SCAN is the observation that skin disease images within a class often exhibit multiple subclusters, characterized by distinct variations in appearance. To improve the performance of few-shot learning (FSL), we focus on learning a high-quality feature encoder that captures the unique subclustered representations within each disease class, enabling better characterization of feature distributions. Specifically, SCAN follows a dual-branch framework, where the first branch learns classwise features to distinguish different skin diseases, and the second branch aims to learn features, which can effectively partition each class into several groups so as to preserve the subclustered structure within each class. To achieve the objective of the second branch, we present a cluster loss to learn image similarities via unsupervised clustering. To ensure that the samples in each subcluster are from the same class, we further design a purity loss to refine the unsupervised clustering results. We evaluate the proposed approach on two public datasets for few-shot skin disease classification. The experimental results validate that our framework outperforms the state-of-the-art methods by around 2%-5% in terms of sensitivity, specificity, accuracy, and F1-score on the SD-198 and Derm7pt datasets.
更多查看译文
关键词
Few-shot learning (FSL),rare skin disease classification
AI 理解论文
溯源树
样例
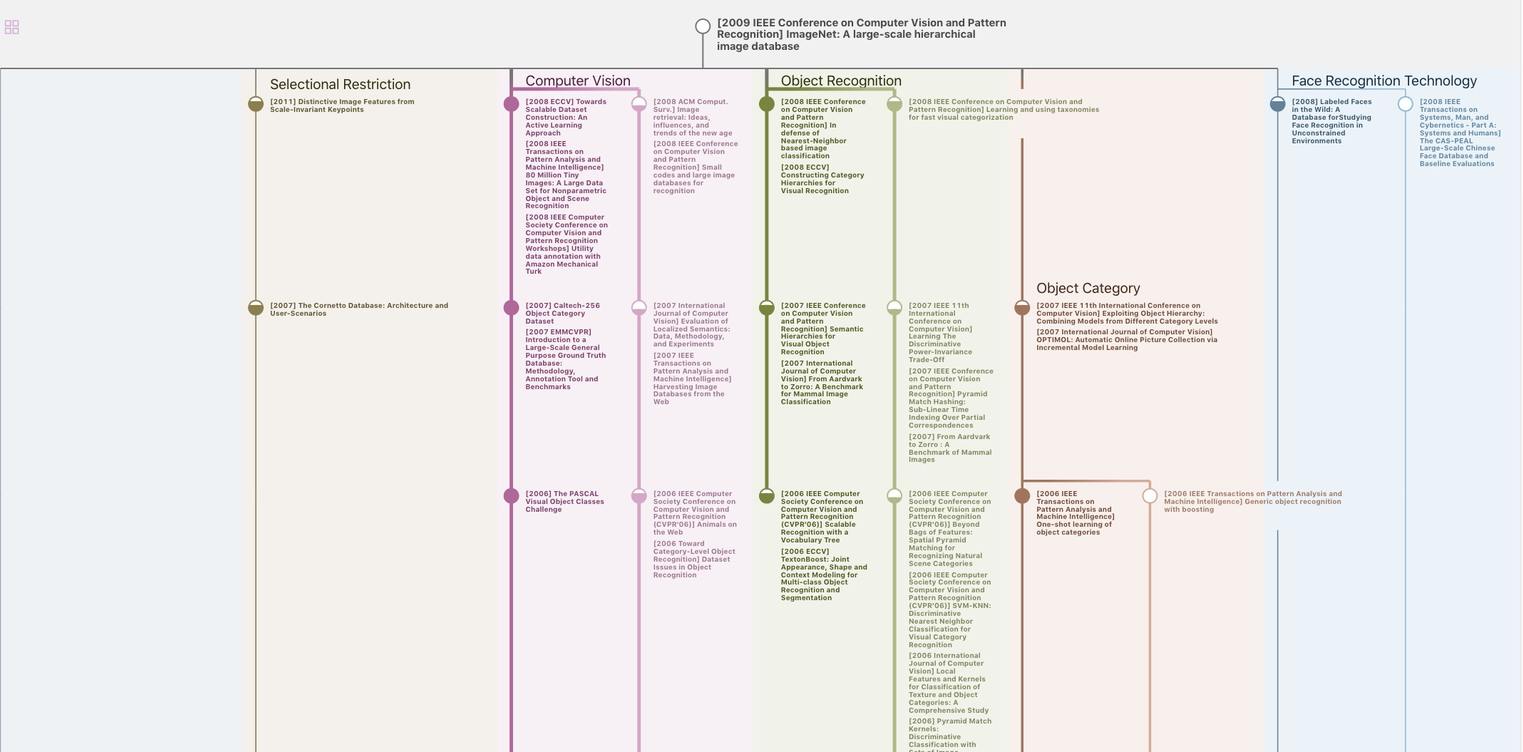
生成溯源树,研究论文发展脉络
Chat Paper
正在生成论文摘要