Cross-User Electromyography Pattern Recognition Based on a Novel Spatial-Temporal Graph Convolutional Network
IEEE TRANSACTIONS ON NEURAL SYSTEMS AND REHABILITATION ENGINEERING(2024)
摘要
With the goal of promoting the development of myoelectric control technology, this paper focuses on exploring graph neural network (GNN) based robust electromyography (EMG) pattern recognition solutions. Given that high-density surface EMG (HD-sEMG) signal contains rich temporal and spatial information, the multi-view spatial-temporal graph convolutional network (MSTGCN)is adopted as the basic classifier, and a feature extraction convolutional neural network (CNN) module is designed and integrated into MSTGCN to generate a new model called CNN-MSTGCN. The EMG pattern recognition experiments are conducted on HD-sEMG data of 17 gestures from 11 subjects. The ablation experiments show that each functional module of the proposed CNN-MSTGCN network has played a more or less positive role in improving the performance of EMG pattern recognition. The user-independent recognition experiments and the transfer learning-based cross-user recognition experiments verify the advantages of the proposed CNN-MSTGCN network in improving recognition rate and reducing user training burden. In the user-independent recognition experiments, CNN-MSTGCN achieves the recognition rate of 68%, which is significantly better than those obtained by residual network-50 (ResNet50, 47.5%, p < 0.001) and long-short-term-memory (LSTM, 57.1%, p=0.045). In the transfer learning-based cross-user recognition experiments, TL-CMSTGCN achieves an impressive recognition rate of 92.3%, which is significantly superior to both TL-ResNet50 (84.6%, p = 0.003) and TL-LSTM (85.3%, p = 0.008). The research results of this paper indicate that GNN has certain advantages in overcoming the impact of individual differences, and can be used to provide possible solutions for achieving robust EMG pattern recognition technology.
更多查看译文
关键词
EMG,gesture recognition,graph neural network,transfer learning,user-independent
AI 理解论文
溯源树
样例
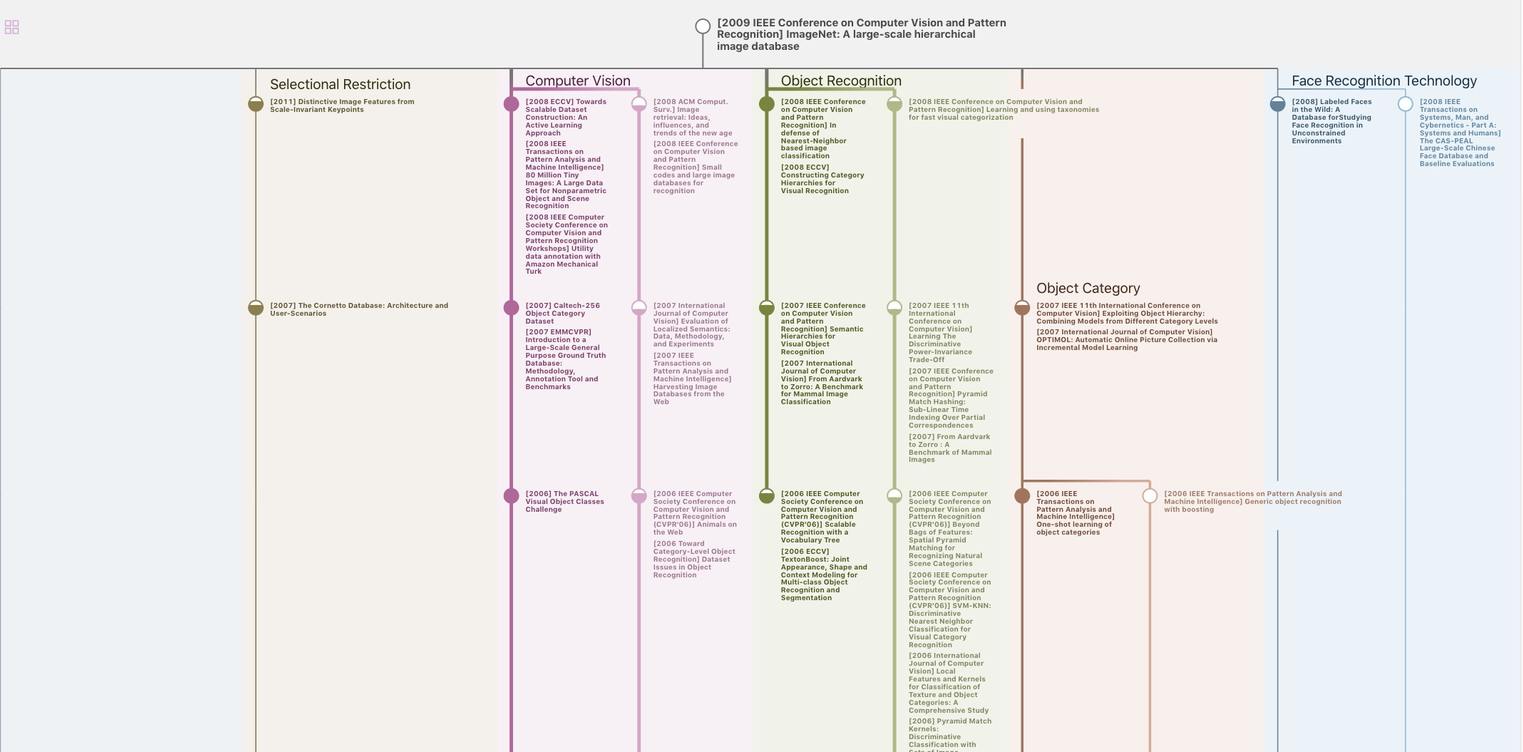
生成溯源树,研究论文发展脉络
Chat Paper
正在生成论文摘要