Neural Graph Refinement for Robust Recognition of Nuclei Communities in Histopathological Landscape
IEEE TRANSACTIONS ON IMAGE PROCESSING(2024)
摘要
Accurate classification of nuclei communities is an important step towards timely treating the cancer spread. Graph theory provides an elegant way to represent and analyze nuclei communities within the histopathological landscape in order to perform tissue phenotyping and tumor profiling tasks. Many researchers have worked on recognizing nuclei regions within the histology images in order to grade cancerous progression. However, due to the high structural similarities between nuclei communities, defining a model that can accurately differentiate between nuclei pathological patterns still needs to be solved. To surmount this challenge, we present a novel approach, dubbed neural graph refinement, that enhances the capabilities of existing models to perform nuclei recognition tasks by employing graph representational learning and broadcasting processes. Based on the physical interaction of the nuclei, we first construct a fully connected graph in which nodes represent nuclei and adjacent nodes are connected to each other via an undirected edge. For each edge and node pair, appearance and geometric features are computed and are then utilized for generating the neural graph embeddings. These embeddings are used for diffusing contextual information to the neighboring nodes, all along a path traversing the whole graph to infer global information over an entire nuclei network and predict pathologically meaningful communities. Through rigorous evaluation of the proposed scheme across four public datasets, we showcase that learning such communities through neural graph refinement produces better results that outperform state-of-the-art methods.
更多查看译文
关键词
Nuclei communities,graph representational learning,histology images,colorectal cancer
AI 理解论文
溯源树
样例
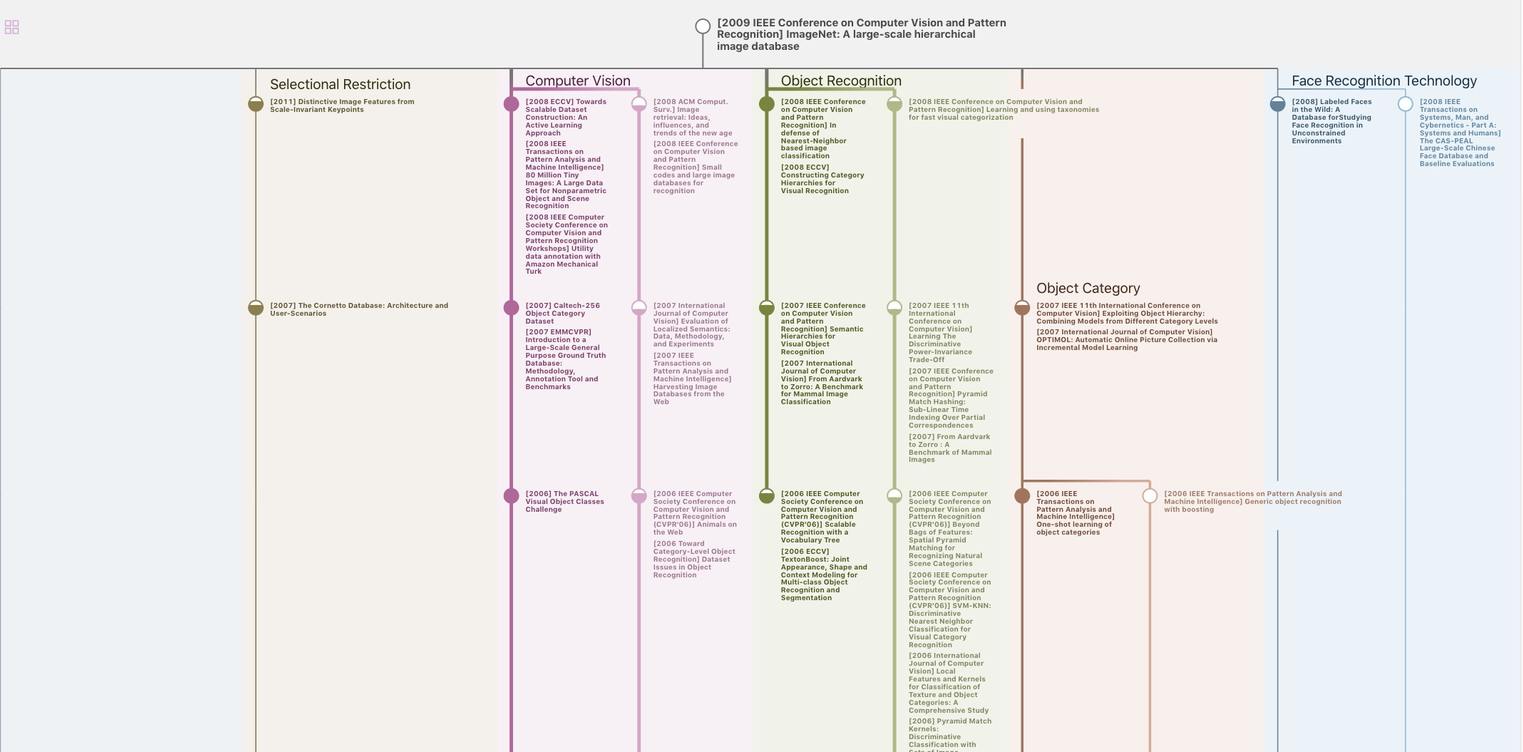
生成溯源树,研究论文发展脉络
Chat Paper
正在生成论文摘要