Fault anomaly detection method of aero-engine rolling bearing based on distillation learning
ISA TRANSACTIONS(2024)
摘要
In this study, we address the issue of limited generalization capabilities in intelligent diagnosis models caused by the lack of high-quality fault data samples for aero-engine rolling bearings. We provide a fault anomaly detection technique based on distillation learning to address this issue. Two Vision Transformer (ViT) models are specifically used in the distillation learning process, one of which serves as the teacher network and the other as the student network. By using a small-scale student network model, the computational efficiency of the model is increased without sacrificing model accuracy. For feature-centered representation, new loss and anomaly score functions are created, and an enhanced Transformer encoder with the residual block is proposed. Then, a rolling bearing dynamics simulation method is used to obtain rich fault sample data, and the pre-training of the teacher network is completed. For anomaly detection, the training of the student network is completed based on the proposed loss function and the pre-trained teacher network, using only the vibration acceleration samples obtained from the normal state. Finally, the trained completed network and the designed anomaly score function are used to achieve the anomaly detection of rolling bearing faults. The experimental validation was carried out on two sets of test data and one set of real vibration data of a whole aero-engine, and the detection accuracy reached 100 %. The results show that the proposed method has a high capability of rolling bearing fault anomaly detection.
更多查看译文
关键词
Distillation learning,Rolling bearings,Anomaly detection,Vision transformer,Aero-engine
AI 理解论文
溯源树
样例
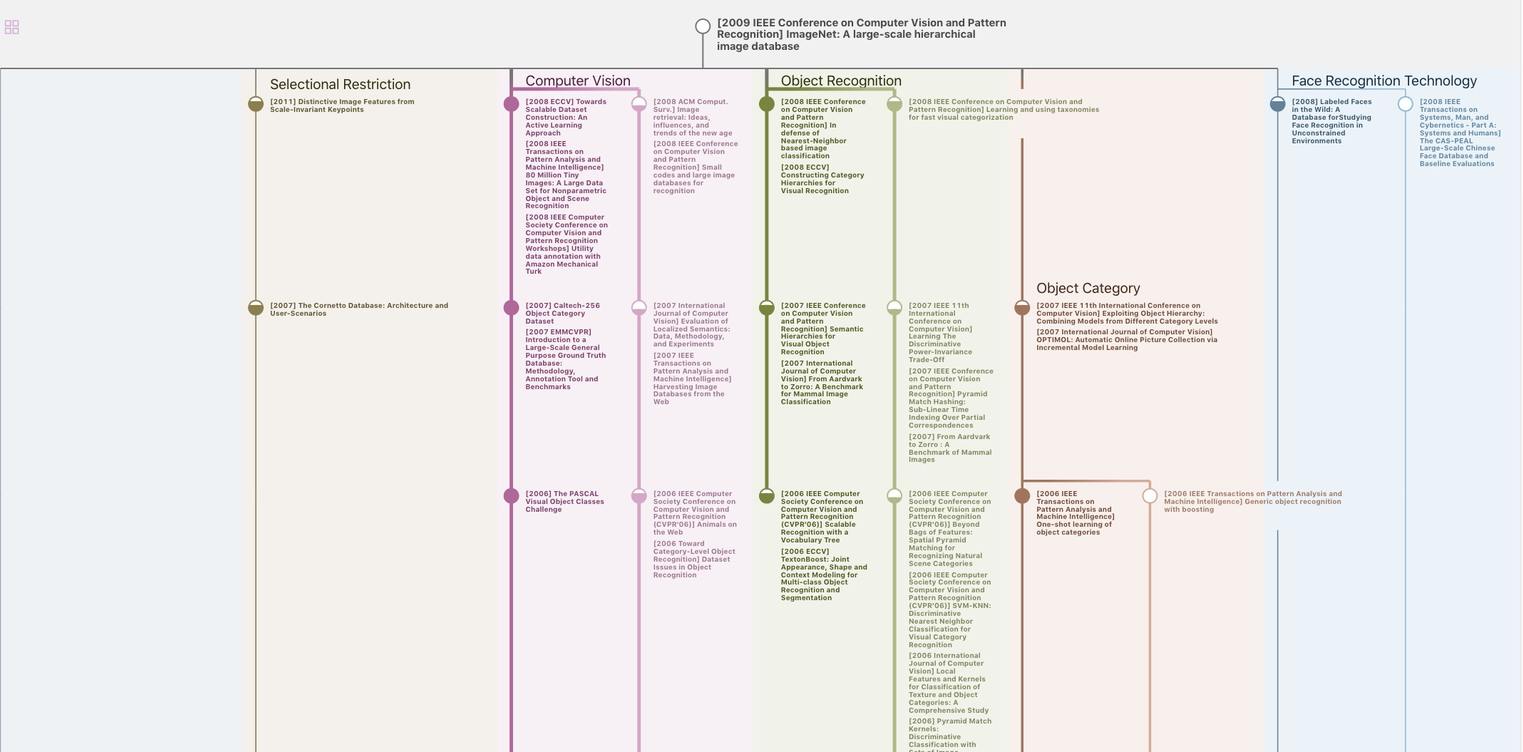
生成溯源树,研究论文发展脉络
Chat Paper
正在生成论文摘要