Quantification of contamination, ecological risk index, and health risk assessment of groundwater using artificial neural network and multi-linear regression modeling approaches within Egbema, Nigeria
Arabian Journal of Geosciences(2023)
摘要
This paper examined the efficiency of artificial neural network (ANN) and multivariate linear regression (MLR) models in the prediction of groundwater quality parameters such as ecological risk index (ERI), pollution load index (PLI), metal pollution index (MPI), Nemerow pollution index (NPI), and geoaccumulation index ( I geo ). Forty groundwater samples were collected systematically and analyzed for mainly heavy metals. Results revealed that adopting measured parameters is effective in modeling the parameters with a high level of accuracy. Contamination factor results reveal that Ni, Zn, Pb, Cd, and Cu have relatively low values < 1 within the region while the iron values ranged from low contamination to very high contamination (> 6). PLI, MPI, and ERI results indicated low pollution. NPI results indicated that the majority of the samples were heavily polluted. Quantification of contamination results revealed that most of the sample’s quality was geogenically influenced. I geo results revealed that most of the samples had extreme pollution. The health risk assessment results revealed that children are substantially prone to more health risk more than adults. The ANN and MLR models showed a high effective tendency in the prediction of ERI, PLI, MPI, NPI, and I geo . Principal component analysis results showed appreciable variable loadings while the correlation matrix results reveal that there exists weak and positive correlation among elements. Based on the outcome of this study, this research recommends the use of ANN and MLR models in the prediction of groundwater quality parameters as they yielded positive, reliable, acceptable, and appropriate accuracy performances.
更多查看译文
关键词
ecological risk index,groundwater,health risk assessment,risk assessment,artificial neural network,multi-linear
AI 理解论文
溯源树
样例
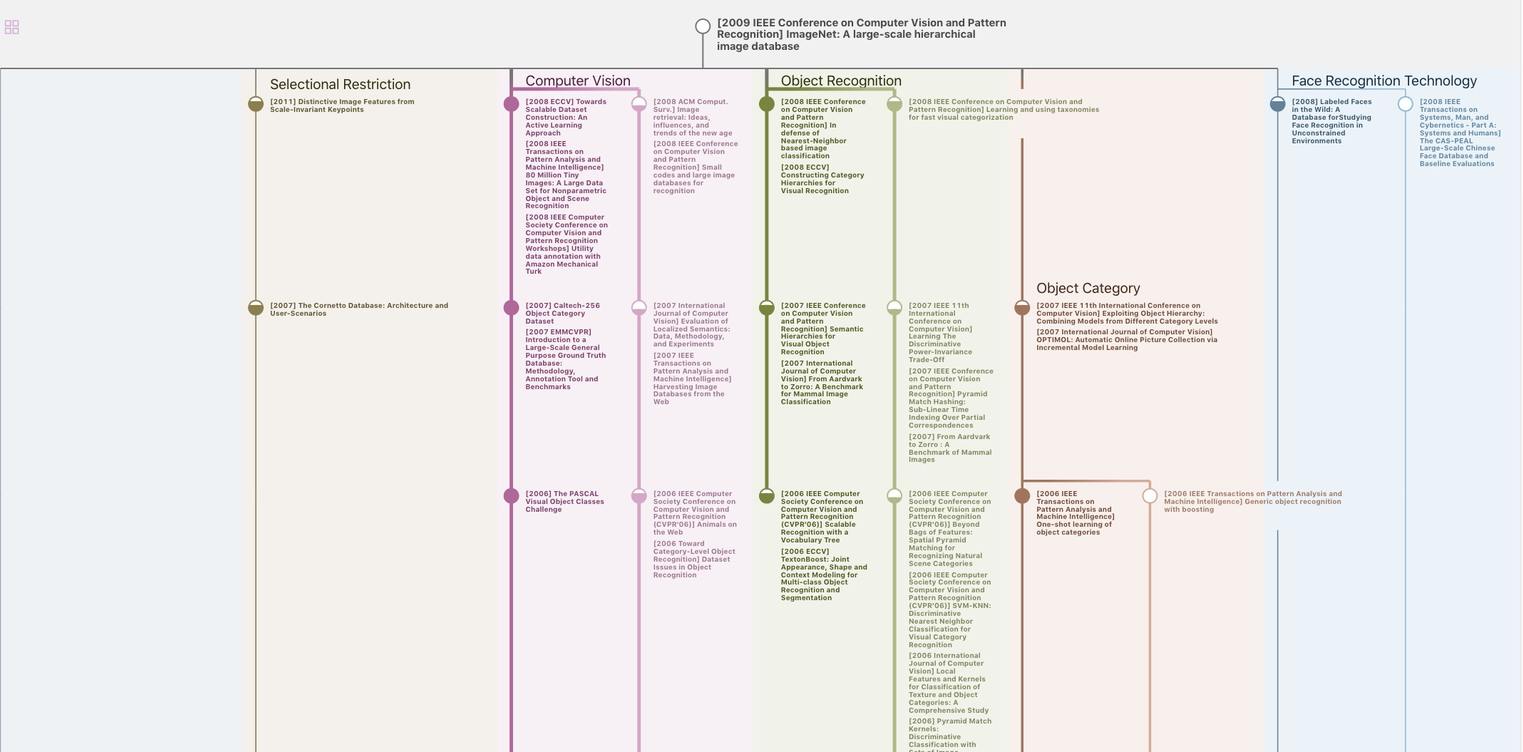
生成溯源树,研究论文发展脉络
Chat Paper
正在生成论文摘要