Safeguarded Progress in Reinforcement Learning: Safe Bayesian Exploration for Control Policy Synthesis
AAAI 2024(2024)
摘要
This paper addresses the problem of maintaining safety during training in Reinforcement Learning (RL), such that the safety constraint violations are bounded at any point during learning. As enforcing safety during training might severely limit the agent’s exploration, we propose here a new architecture that handles the trade-off between efficient progress and safety during exploration. As the exploration progresses, we update via Bayesian inference Dirichlet-Categorical models of the transition probabilities of the Markov decision process that describes the environment dynamics. We then propose a way to approximate moments of belief about the risk associated to the action selection policy. We demonstrate that this approach can be easily interleaved with RL and we present experimental results to showcase the performance of the overall architecture.
更多查看译文
关键词
General
AI 理解论文
溯源树
样例
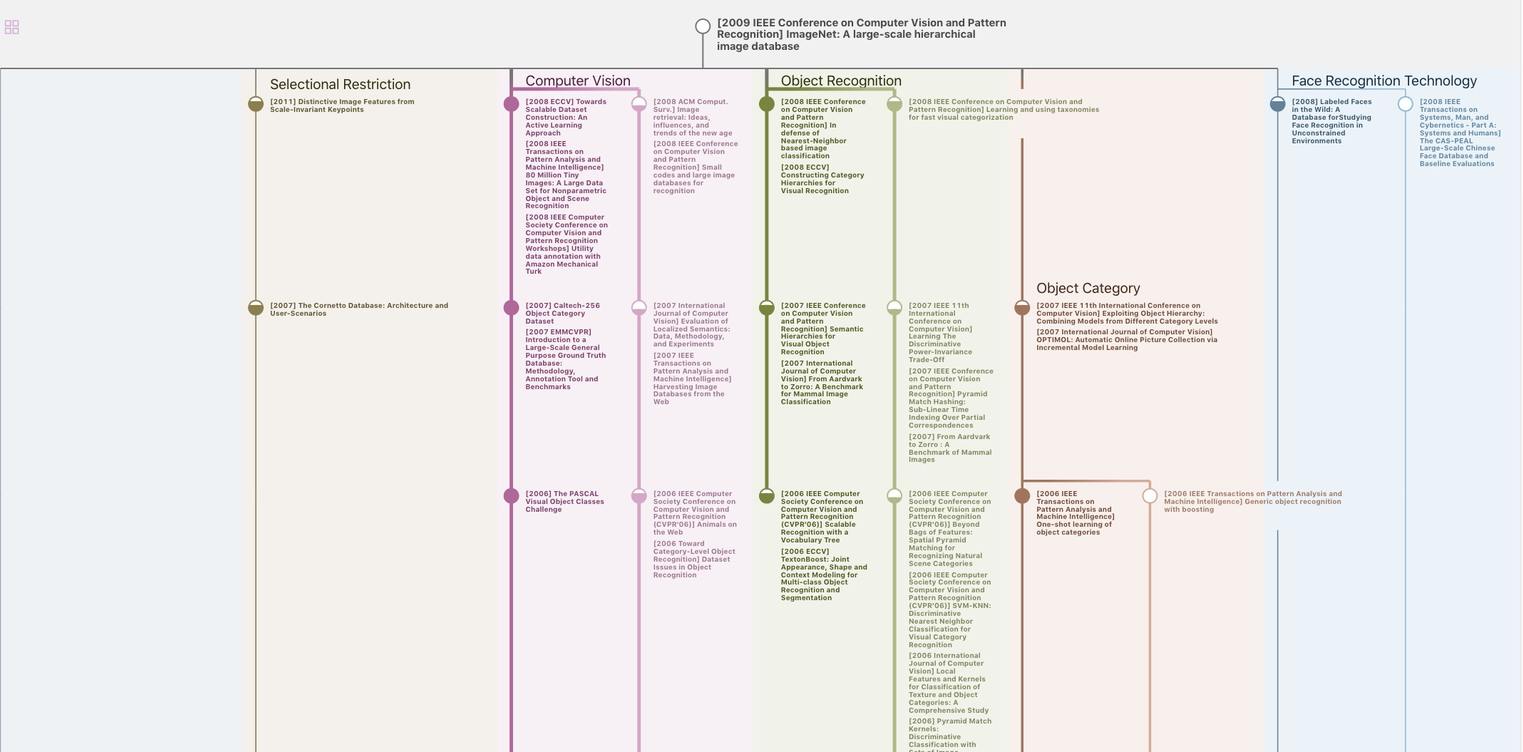
生成溯源树,研究论文发展脉络
Chat Paper
正在生成论文摘要