A Versatile Causal Discovery Framework to Allow Causally-Related Hidden Variables
CoRR(2023)
摘要
Most existing causal discovery methods rely on the assumption of no latent confounders, limiting their applicability in solving real-life problems. In this paper, we introduce a novel, versatile framework for causal discovery that accommodates the presence of causally-related hidden variables almost everywhere in the causal network (for instance, they can be effects of observed variables), based on rank information of covariance matrix over observed variables. We start by investigating the efficacy of rank in comparison to conditional independence and, theoretically, establish necessary and sufficient conditions for the identifiability of certain latent structural patterns. Furthermore, we develop a Rank-based Latent Causal Discovery algorithm, RLCD, that can efficiently locate hidden variables, determine their cardinalities, and discover the entire causal structure over both measured and hidden ones. We also show that, under certain graphical conditions, RLCD correctly identifies the Markov Equivalence Class of the whole latent causal graph asymptotically. Experimental results on both synthetic and real-world personality data sets demonstrate the efficacy of the proposed approach in finite-sample cases.
更多查看译文
关键词
Causal Discovery,Latent Variables
AI 理解论文
溯源树
样例
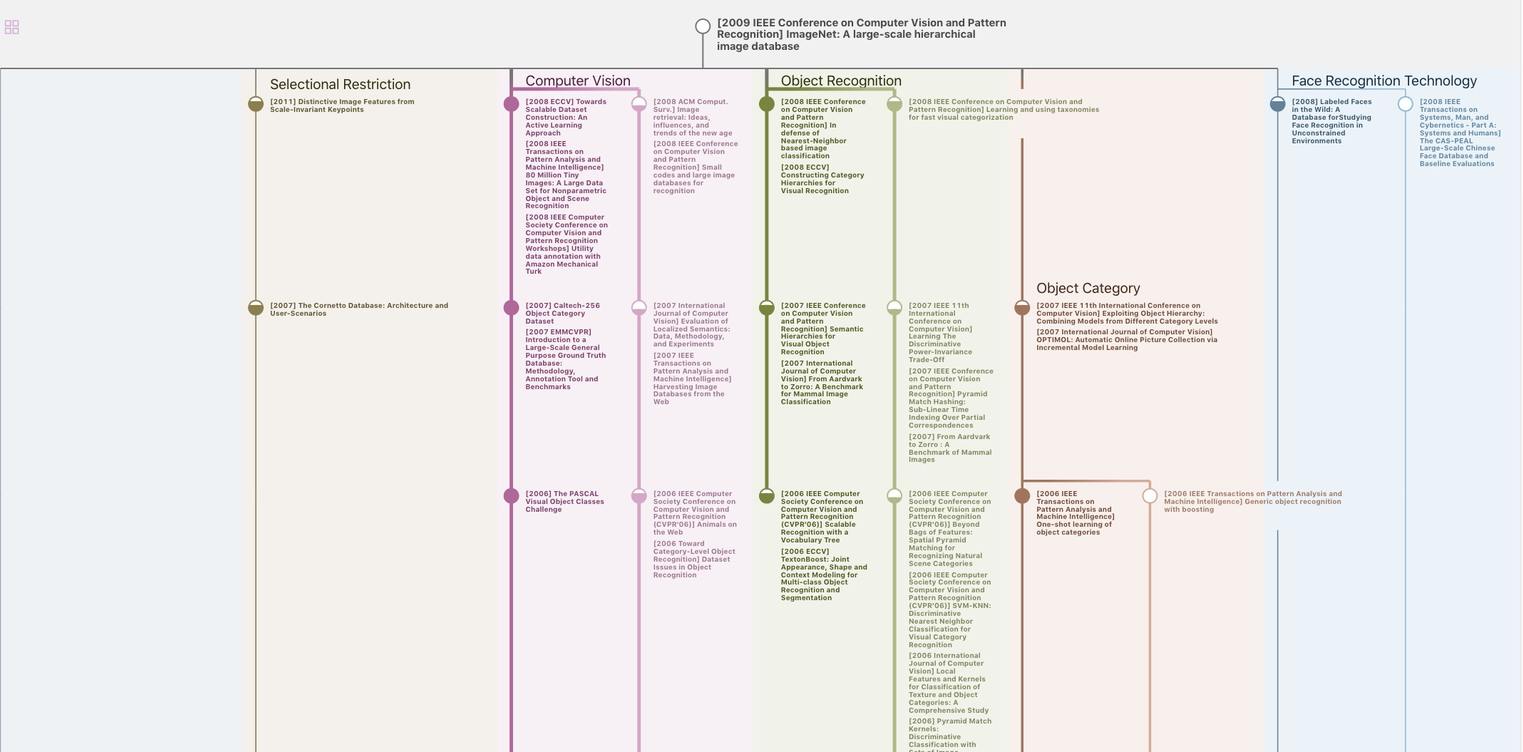
生成溯源树,研究论文发展脉络
Chat Paper
正在生成论文摘要