Semi-Supervised Clustering via Structural Entropy with Different Constraints
CoRR(2023)
摘要
Semi-supervised clustering techniques have emerged as valuable tools for leveraging prior information in the form of constraints to improve the quality of clustering outcomes. Despite the proliferation of such methods, the ability to seamlessly integrate various types of constraints remains limited. While structural entropy has proven to be a powerful clustering approach with wide-ranging applications, it has lacked a variant capable of accommodating these constraints. In this work, we present Semi-supervised clustering via Structural Entropy (SSE), a novel method that can incorporate different types of constraints from diverse sources to perform both partitioning and hierarchical clustering. Specifically, we formulate a uniform view for the commonly used pairwise and label constraints for both types of clustering. Then, we design objectives that incorporate these constraints into structural entropy and develop tailored algorithms for their optimization. We evaluate SSE on nine clustering datasets and compare it with eleven semi-supervised partitioning and hierarchical clustering methods. Experimental results demonstrate the superiority of SSE on clustering accuracy with different types of constraints. Additionally, the functionality of SSE for biological data analysis is demonstrated by cell clustering experiments conducted on four single-cell RNAseq datasets.
更多查看译文
AI 理解论文
溯源树
样例
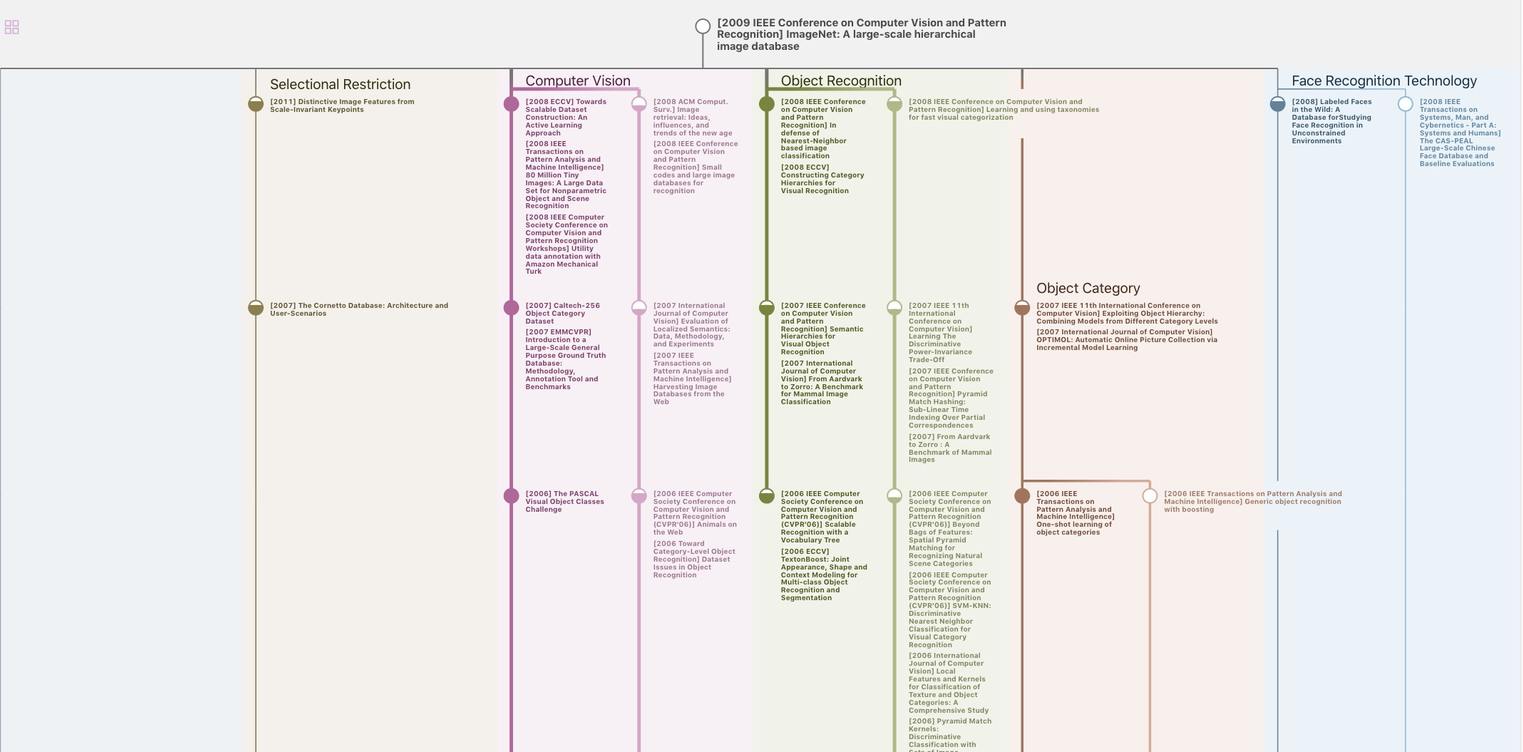
生成溯源树,研究论文发展脉络
Chat Paper
正在生成论文摘要